Tuning structure learning algorithms with out-of-sample and resampling strategies
CoRR(2023)
摘要
One of the challenges practitioners face when applying structure learning algorithms to their data involves determining a set of hyperparameters; otherwise, a set of hyperparameter defaults is assumed. The optimal hyperparameter configuration often depends on multiple factors, including the size and density of the usually unknown underlying true graph, the sample size of the input data, and the structure learning algorithm. We propose a novel hyperparameter tuning method, called the Out-of-sample Tuning for Structure Learning (OTSL), that employs out-of-sample and resampling strategies to estimate the optimal hyperparameter configuration for structure learning, given the input data set and structure learning algorithm. Synthetic experiments show that employing OTSL as a means to tune the hyperparameters of hybrid and score-based structure learning algorithms leads to improvements in graphical accuracy compared to the state-of-the-art. We also illustrate the applicability of this approach to real datasets from different disciplines.
更多查看译文
关键词
tuning,structure,resampling
AI 理解论文
溯源树
样例
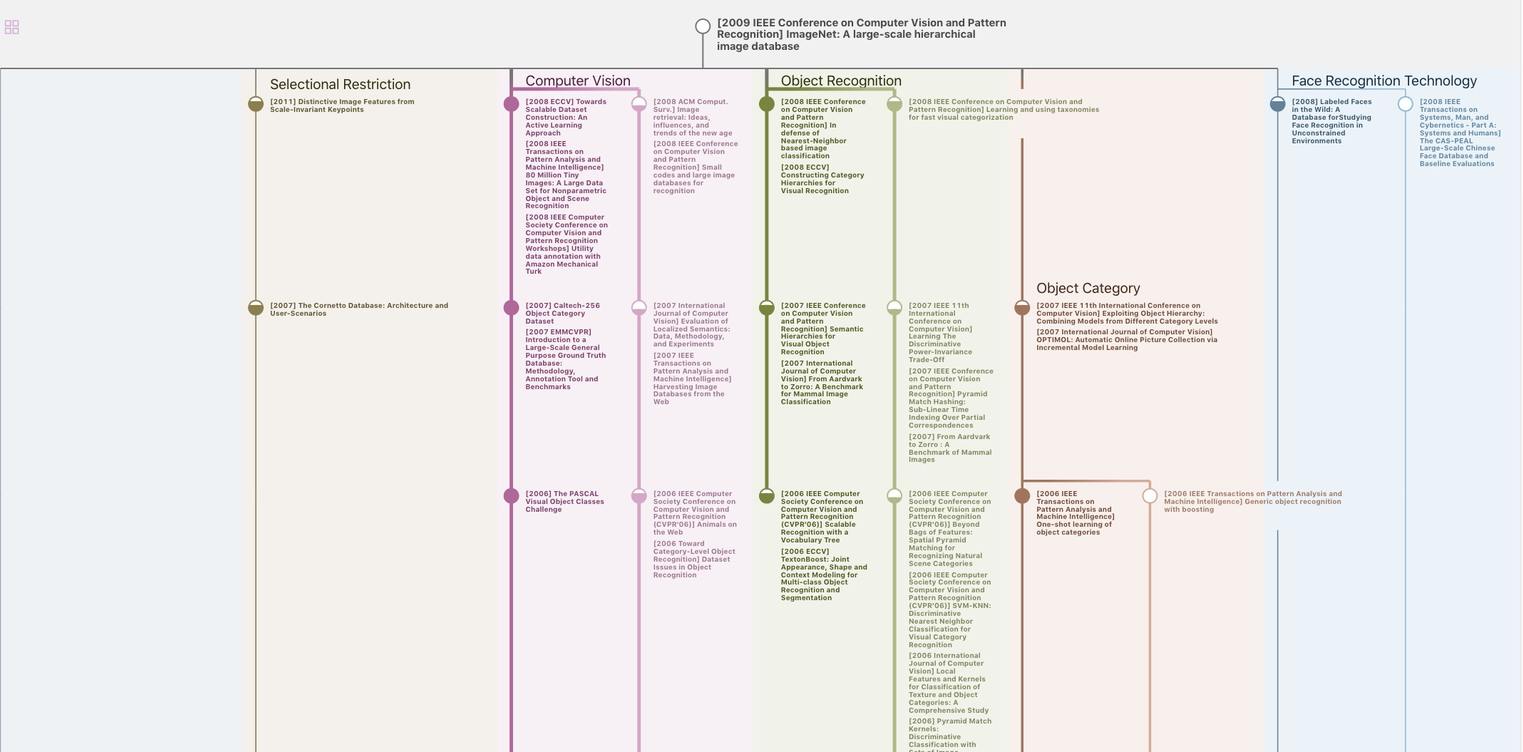
生成溯源树,研究论文发展脉络
Chat Paper
正在生成论文摘要