Best Practices for Machine Learning Systems: An Industrial Framework for Analysis and Optimization
CoRR(2023)
摘要
In the last few years, the Machine Learning (ML) and Artificial Intelligence community has developed an increasing interest in Software Engineering (SE) for ML Systems leading to a proliferation of best practices, rules, and guidelines aiming at improving the quality of the software of ML Systems. However, understanding their impact on the overall quality has received less attention. Practices are usually presented in a prescriptive manner, without an explicit connection to their overall contribution to software quality. Based on the observation that different practices influence different aspects of software-quality and that one single quality aspect might be addressed by several practices we propose a framework to analyse sets of best practices with focus on quality impact and prioritization of their implementation. We first introduce a hierarchical Software Quality Model (SQM) specifically tailored for ML Systems. Relying on expert knowledge, the connection between individual practices and software quality aspects is explicitly elicited for a large set of well-established practices. Applying set-function optimization techniques we can answer questions such as what is the set of practices that maximizes SQM coverage, what are the most important ones, which practices should be implemented in order to improve specific quality aspects, among others. We illustrate the usage of our framework by analyzing well-known sets of practices.
更多查看译文
关键词
machine learning systems,industrial framework,best practices,optimization
AI 理解论文
溯源树
样例
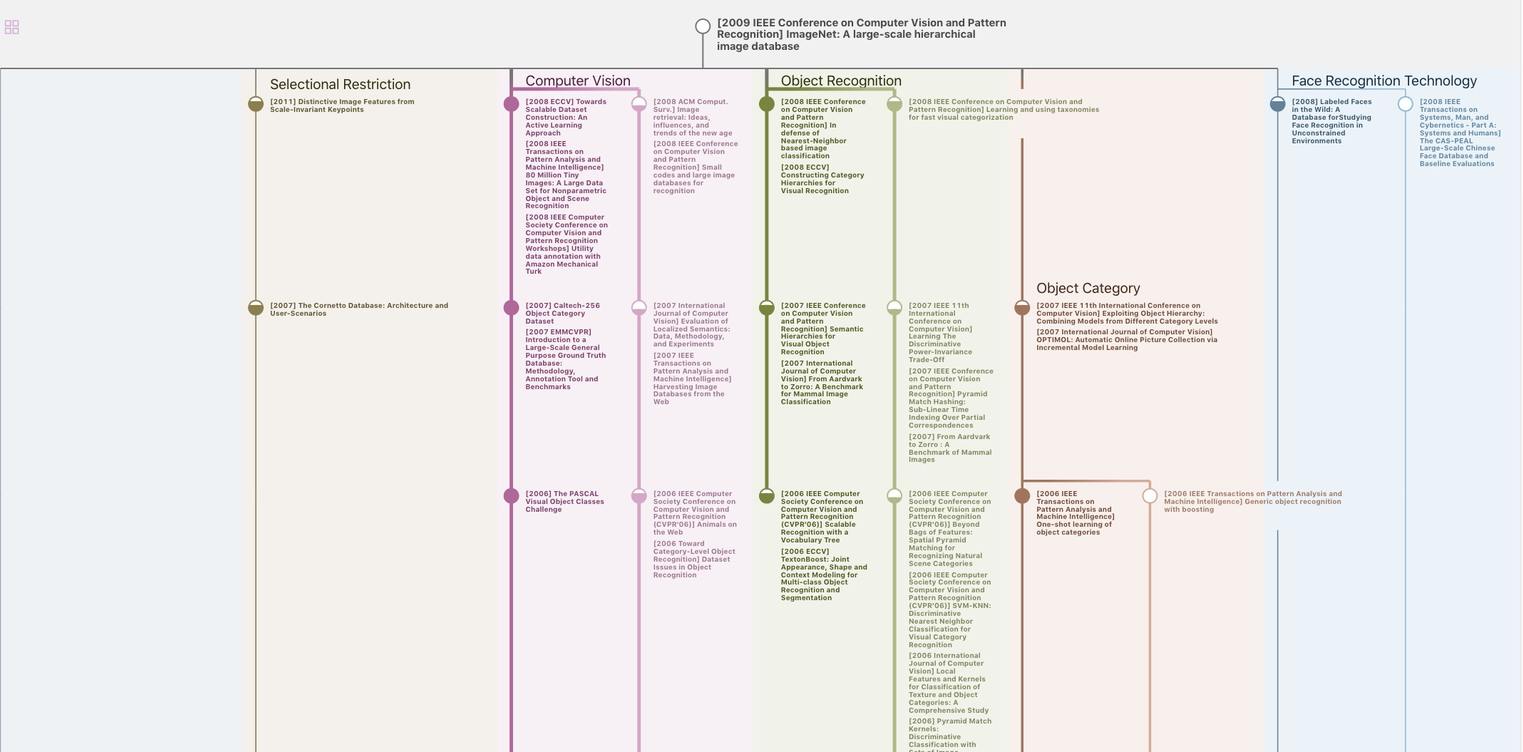
生成溯源树,研究论文发展脉络
Chat Paper
正在生成论文摘要