On-Policy Distillation of Language Models: Learning from Self-Generated Mistakes
ICLR 2024(2023)
摘要
Knowledge distillation (KD) is widely used for compressing a teacher model to
reduce its inference cost and memory footprint, by training a smaller student
model. However, current KD methods for auto-regressive sequence models suffer
from distribution mismatch between output sequences seen during training and
those generated by the student during inference. To address this issue, we
introduce Generalized Knowledge Distillation (GKD). Instead of solely relying
on a fixed set of output sequences, GKD trains the student on its
self-generated output sequences by leveraging feedback from the teacher on such
sequences. Unlike supervised KD approaches, GKD also offers the flexibility to
employ alternative loss functions between the student and teacher, which can be
useful when the student lacks the expressivity to mimic the teacher's
distribution. Furthermore, GKD facilitates the seamless integration of
distillation with RL fine-tuning (RLHF). We demonstrate the efficacy of GKD for
distilling auto-regressive language models on summarization, translation, and
arithmetic reasoning tasks, and task-agnostic distillation for
instruction-tuning.
更多查看译文
关键词
Language models,Distillation,RLHF
AI 理解论文
溯源树
样例
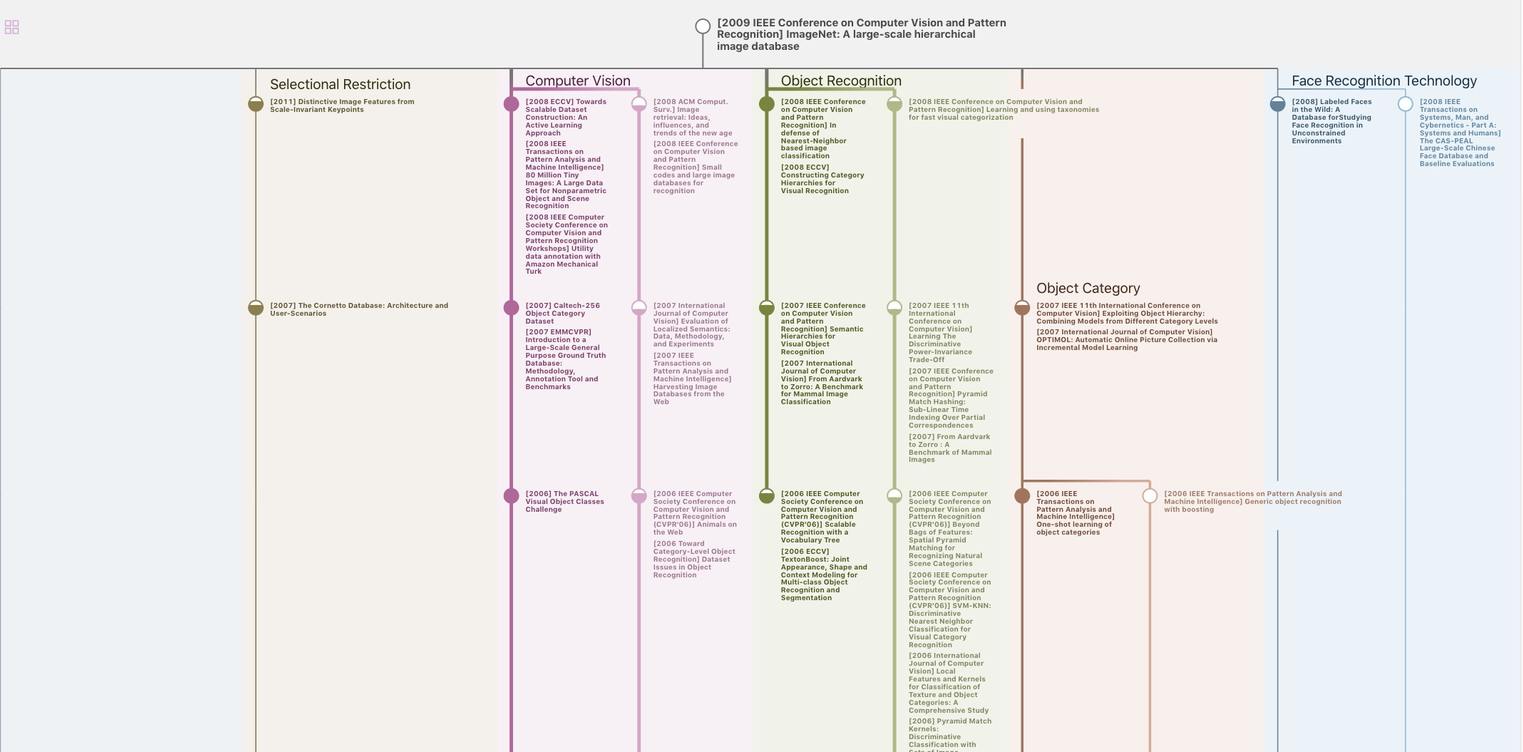
生成溯源树,研究论文发展脉络
Chat Paper
正在生成论文摘要