A Physics-Informed Deep Learning Model of the Hot Tail Runaway Electron Seed
Physics of Plasmas(2023)
摘要
A challenging aspect of the description of a tokamak disruption is evaluating
the hot tail runaway electron (RE) seed that emerges during the thermal quench.
This problem is made challenging due to the requirement of describing a
strongly non-thermal electron distribution, together with the need to
incorporate a diverse range of multiphysics processes including
magnetohydrodynamic instabilities, impurity transport, and radiative losses.
The present work develops a physics-informed neural network (PINN) tailored to
the solution of the hot tail seed during an idealized axisymmetric thermal
quench. Here, a PINN is developed to identify solutions to the adjoint
relativistic Fokker-Planck equation in the presence of a rapid quench of the
plasma's thermal energy. It is shown that the PINN is able to accurately
predict the hot tail seed across a range of parameters including the thermal
quench time scale, initial plasma temperature, and local current density, in
the absence of experimental or simulation data. The hot tail PINN is verified
by comparison with a direct Monte Carlo solution, with excellent agreement
found across a broad range of thermal quench conditions.
更多查看译文
关键词
runaway electron seed,deep learning,deep learning model,physics-informed
AI 理解论文
溯源树
样例
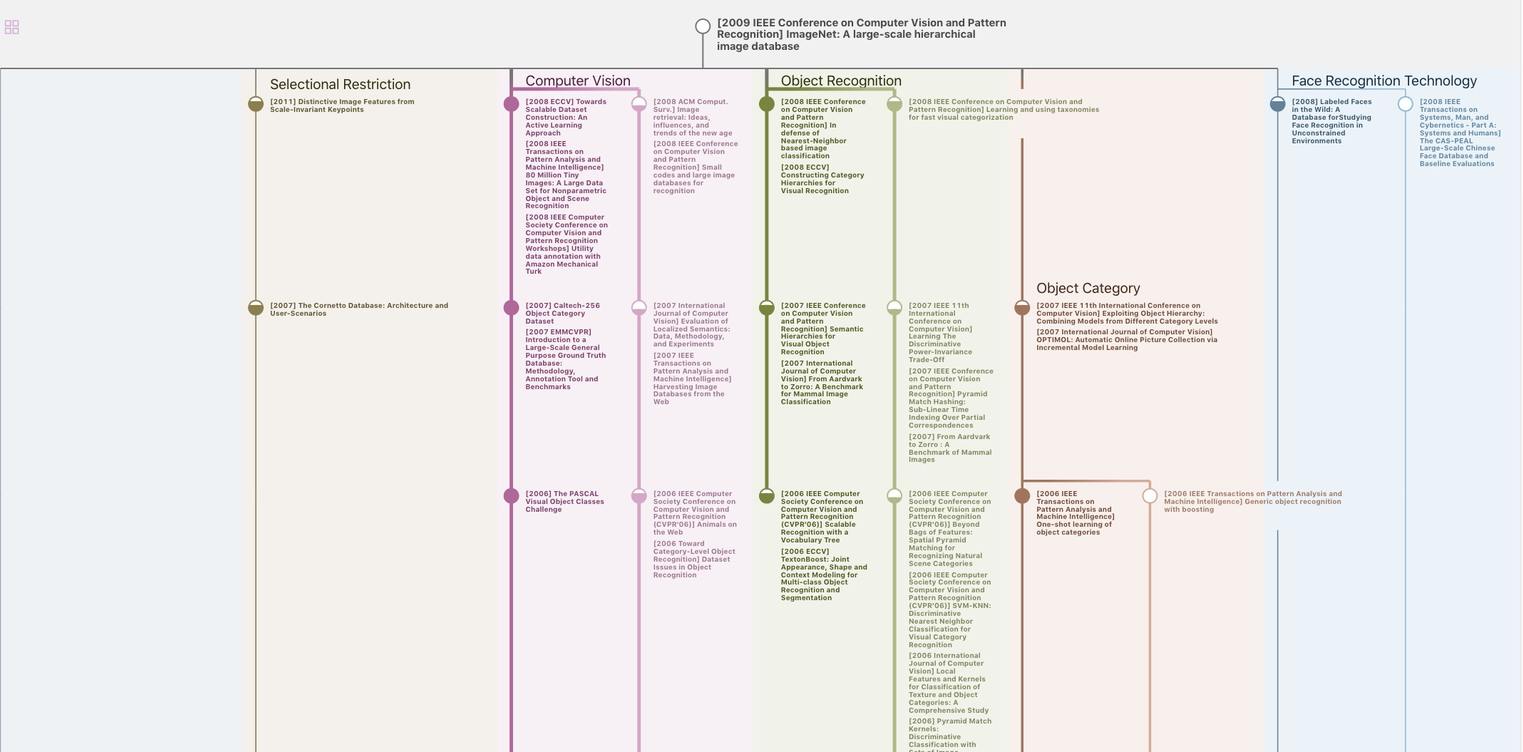
生成溯源树,研究论文发展脉络
Chat Paper
正在生成论文摘要