MiTFed: A Privacy Preserving Collaborative Network Attack Mitigation Framework Based on Federated Learning Using SDN and Blockchain
IEEE Transactions on Network Science and Engineering(2023)
摘要
Distributed denial-of-service (DDoS) attacks continue to grow at a rapid rate plaguing Internet Service Providers (ISPs) and individuals in a stealthy way. Thus, intrusion detection systems (IDSs) must evolve to cope with these increasingly sophisticated and challenging security threats. Traditional IDSs are prone to zero-day attacks since they are usually signature-based detection systems. The recent advent of machine learning and deep learning (ML/DL) techniques can help strengthen these IDSs. However, the lack of up-to-date labeled training datasets makes these ML/DL based IDSs inefficient. The privacy nature of these datasets and widespread emergence of adversarial attacks make it difficult for major organizations to share their sensitive data. Federated Learning (FL) is gaining momentum from both academia and industry as a new sub-field of ML that aims to train a global statistical model across multiple distributed users, referred to as collaborators, without sharing their private data. Due to its privacy-preserving nature, FL has the potential to enable privacy-aware learning between a large number of collaborators. This paper presents a novel framework, called MiTFed, that allows multiple software defined networks (SDN) domains (
$i.e.,$
collaborators) to collaboratively build a global intrusion detection model without sharing their sensitive datasets. In particular, MiTFed consists of: (1) a novel distributed architecture that allows multiple SDN based domains to securely collaborate in order to cope with sophisticated security threats while preserving the privacy of each SDN domain; (2) a novel Secure Multiparty Computation (SMPC) scheme to securely aggregate local model updates; and (3) a blockchain based scheme that uses Ethereum smart contracts to maintain the collaboration in a fully decentralized, trustworthy, flexible, and efficient manner. To the best of our knowledge, MiTFed is the first framework that leverages FL, blockchain and SDN technologies to mitigate the new emerging security threats in large scale. To evaluate MiTFed, we conduct several experiments using real-world network attacks; the experimental results using the well-known public network security dataset NSL-KDD show that MiTFed achieves efficiency and high accuracy in detecting the new emerging security threats in both binary and multi-class classification while preserving the privacy of collaborators, making it a promising framework to cope with the new emerging security threats in SDN.
更多查看译文
关键词
DDoS,IDS,Federated Learning,SDN,Blockchain,SMPC,Smart contracts
AI 理解论文
溯源树
样例
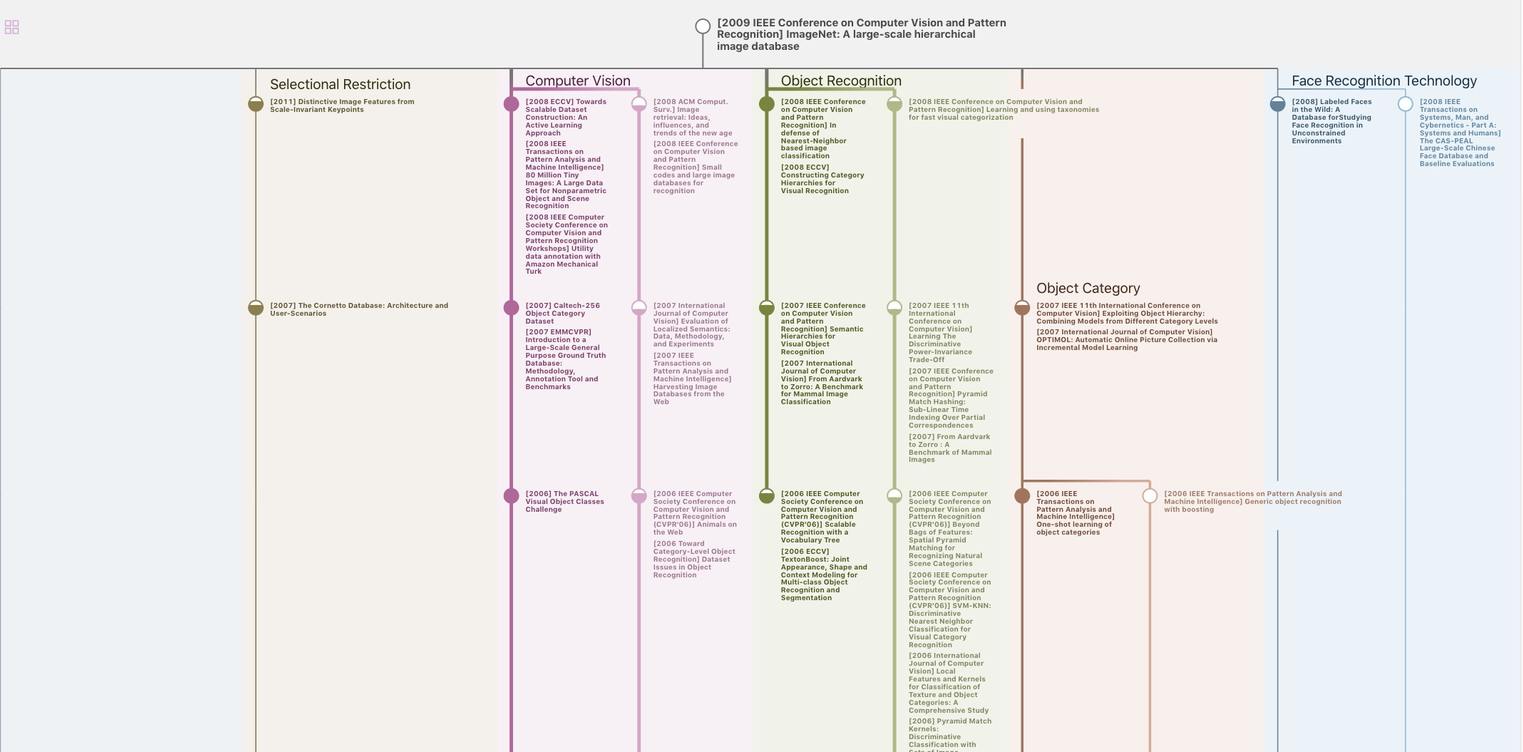
生成溯源树,研究论文发展脉络
Chat Paper
正在生成论文摘要