GIMLET: A Unified Graph-Text Model for Instruction-Based Molecule\n Zero-Shot Learning
NeurIPS(2023)
摘要
Molecule property prediction has gained significant attention in recent years. The main bottleneck is the label insufficiency caused by expensive lab experiments. In order to alleviate this issue and to better leverage textual knowledge for tasks, this study investigates the feasibility of employing natural language instructions to accomplish molecule-related tasks in a zero-shot setting. We discover that existing molecule-text models perform poorly in this setting due to inadequate treatment of instructions and limited capacity for graphs. To overcome these issues, we propose GIMLET, which unifies language models for both graph and text data. By adopting generalized position embedding, our model is extended to encode both graph structures and instruction text without additional graph encoding modules. GIMLET also decouples encoding of the graph from tasks instructions in the attention mechanism, enhancing the generalization of graph features across novel tasks. We construct a dataset consisting of more than two thousand molecule tasks with corresponding instructions derived from task descriptions. We pretrain GIMLET on the molecule tasks along with instructions, enabling the model to transfer effectively to a broad range of tasks. Experimental results demonstrate that GIMLET significantly outperforms molecule-text baselines in instruction-based zero-shot learning, even achieving closed results to supervised GNN models on tasks such as toxcast and muv.
更多查看译文
关键词
learning,graph-text,instruction-based,zero-shot
AI 理解论文
溯源树
样例
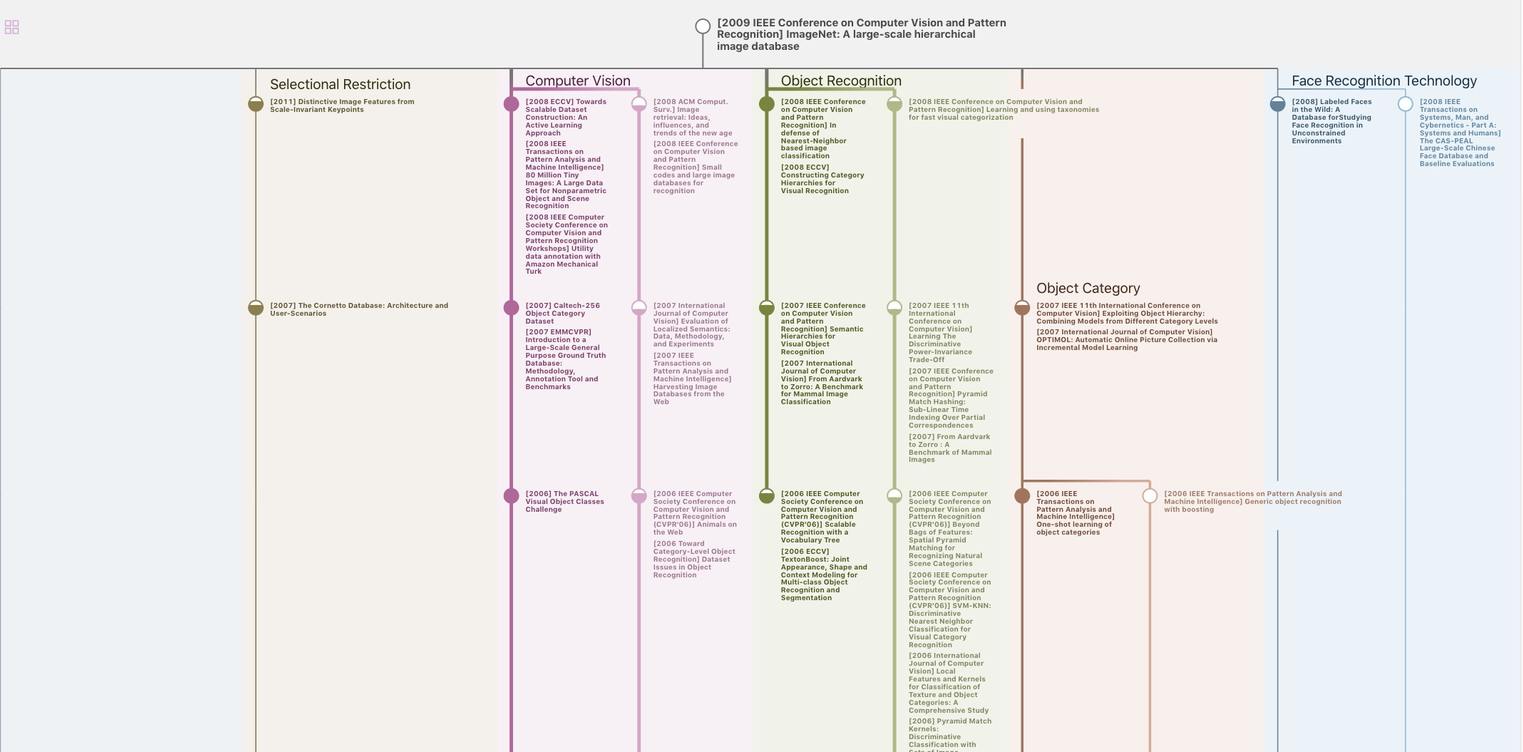
生成溯源树,研究论文发展脉络
Chat Paper
正在生成论文摘要