FuXi: a cascade machine learning forecasting system for 15-day global weather forecast
CoRR(2023)
摘要
Over the past few years, the rapid development of machine learning (ML) models for weather forecasting has led to state-of-the-art ML models that have superior performance compared to the European Centre for Medium-Range Weather Forecasts (ECMWF)’s high-resolution forecast (HRES), which is widely considered as the world’s best physics-based weather forecasting system. Specifically, ML models have outperformed HRES in 10-day forecasts with a spatial resolution of 0.25 ∘ . However, the challenge remains in mitigating the accumulation of forecast errors for longer effective forecasts, such as achieving comparable performance to the ECMWF ensemble in 15-day forecasts. Despite various efforts to reduce accumulation errors, such as implementing autoregressive multi-time step loss, relying on a single model has been found to be insufficient for achieving optimal performance in both short and long lead times. Therefore, we present FuXi, a cascaded ML weather forecasting system that provides 15-day global forecasts at a temporal resolution of 6 hours and a spatial resolution of 0.25 ∘ . FuXi is developed using 39 years of the ECMWF ERA5 reanalysis dataset. The performance evaluation demonstrates that FuXi has forecast performance comparable to ECMWF ensemble mean (EM) in 15-day forecasts. FuXi surpasses the skillful forecast lead time achieved by ECMWF HRES by extending the lead time for Z500 from 9.25 to 10.5 days and for T2M from 10 to 14.5 days. Moreover, the FuXi ensemble is created by perturbing initial conditions and model parameters, enabling it to provide forecast uncertainty and demonstrating promising results when compared to the ECMWF ensemble.
更多查看译文
关键词
forecasting system,cascade machine,machine learning
AI 理解论文
溯源树
样例
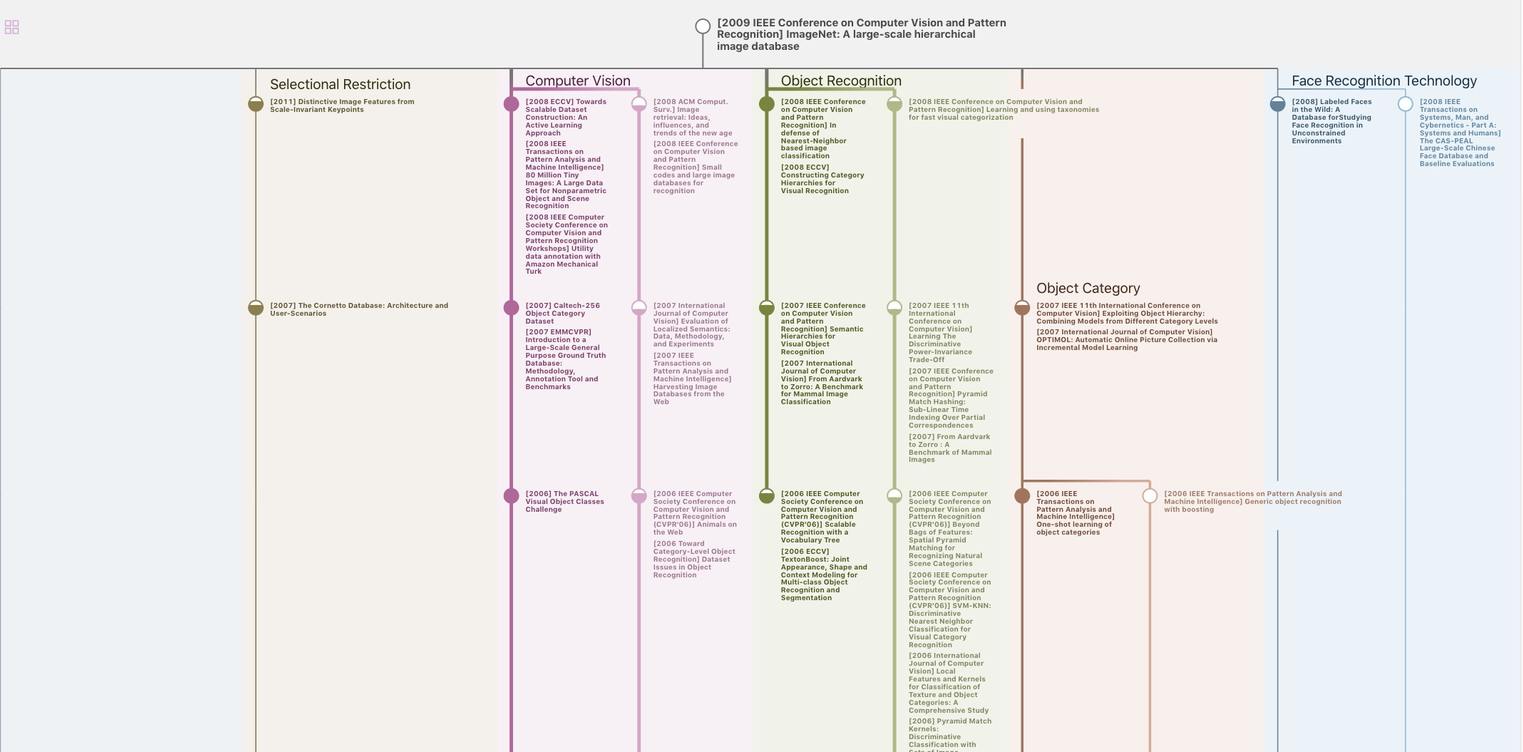
生成溯源树,研究论文发展脉络
Chat Paper
正在生成论文摘要