DP-BREM: Differentially-Private and Byzantine-Robust Federated Learning with Client Momentum
CoRR(2023)
摘要
Federated Learning (FL) allows multiple participating clients to train machine learning models collaboratively by keeping their datasets local and only exchanging the gradient or model updates with a coordinating server. Existing FL protocols were shown to be vulnerable to attacks that aim to compromise data privacy and/or model robustness. Recently proposed defenses focused on ensuring either privacy or robustness, but not both. In this paper, we focus on simultaneously achieving differential privacy (DP) and Byzantine robustness for cross-silo FL, based on the idea of learning from history. The robustness is achieved via client momentum, which averages the updates of each client over time, thus reduces the variance of the honest clients and exposes the small malicious perturbations of Byzantine clients that are undetectable in a single round but accumulate over time. In our initial solution DP-BREM, the DP property is achieved via adding noise to the aggregated momentum, and we account for the privacy cost from the momentum, which is different from the conventional DP-SGD that accounts for the privacy cost from gradient. Since DP-BREM assumes a trusted server (who can obtain clients' local models or updates), we further develop the final solution called DP-BREM+, which achieves the same DP and robustness properties as DP-BREM without a trusted server by utilizing secure aggregation techniques, where DP noise is securely and jointly generated by the clients. Our theoretical analysis on the convergence rate and experimental results under different DP guarantees and attack settings demonstrate that our proposed protocols achieve better privacy-utility tradeoff and stronger Byzantine robustness than several baseline methods.
更多查看译文
关键词
learning
AI 理解论文
溯源树
样例
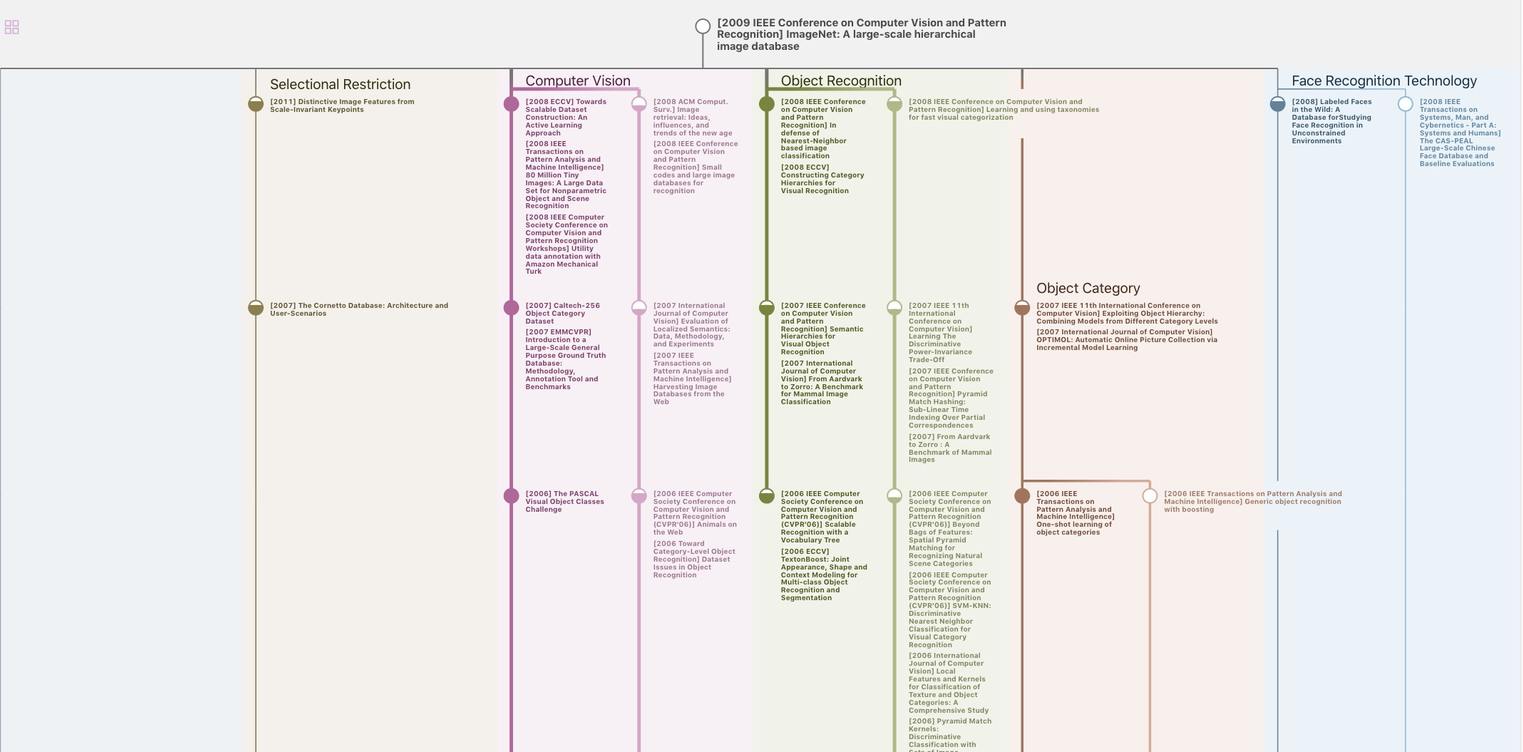
生成溯源树,研究论文发展脉络
Chat Paper
正在生成论文摘要