Workflow Scheduling in Serverless Edge Computing for the Industrial Internet of Things: A Learning Approach
IEEE Transactions on Industrial Informatics(2023)
摘要
Serverless edge computing is seen as a promising enabler to execute differentiated Industrial Internet of Things (IIoT) applications without managing the underlying servers and clusters. In IIoT serverless edge computing, IIoT workflow scheduling for cloud-edge collaborative processing is closely related to the service quality of users. However, serverless functions decomposed by IIoT applications are limited in their deployment at the edge due to the resource-constrained nature of edge infrastructures. In addition, the scheduling of complex IIoT applications supported by serverless computing is more challenging. Therefore, considering the limited function deployment and the complex dependencies of serverless workflows, we model the workflow application as directed acyclic graph and formulate the scheduling problem as a multiobjective optimization problem. A dueling double deep Q-network-based solution is proposed to make scheduling decisions under dynamically changing systems. Extensive simulation experiments are conducted to validate the superiority of the proposed scheme.
更多查看译文
关键词
Deep reinforcement learning (DRL),multi-objective optimization,serverless edge computing,workflow scheduling
AI 理解论文
溯源树
样例
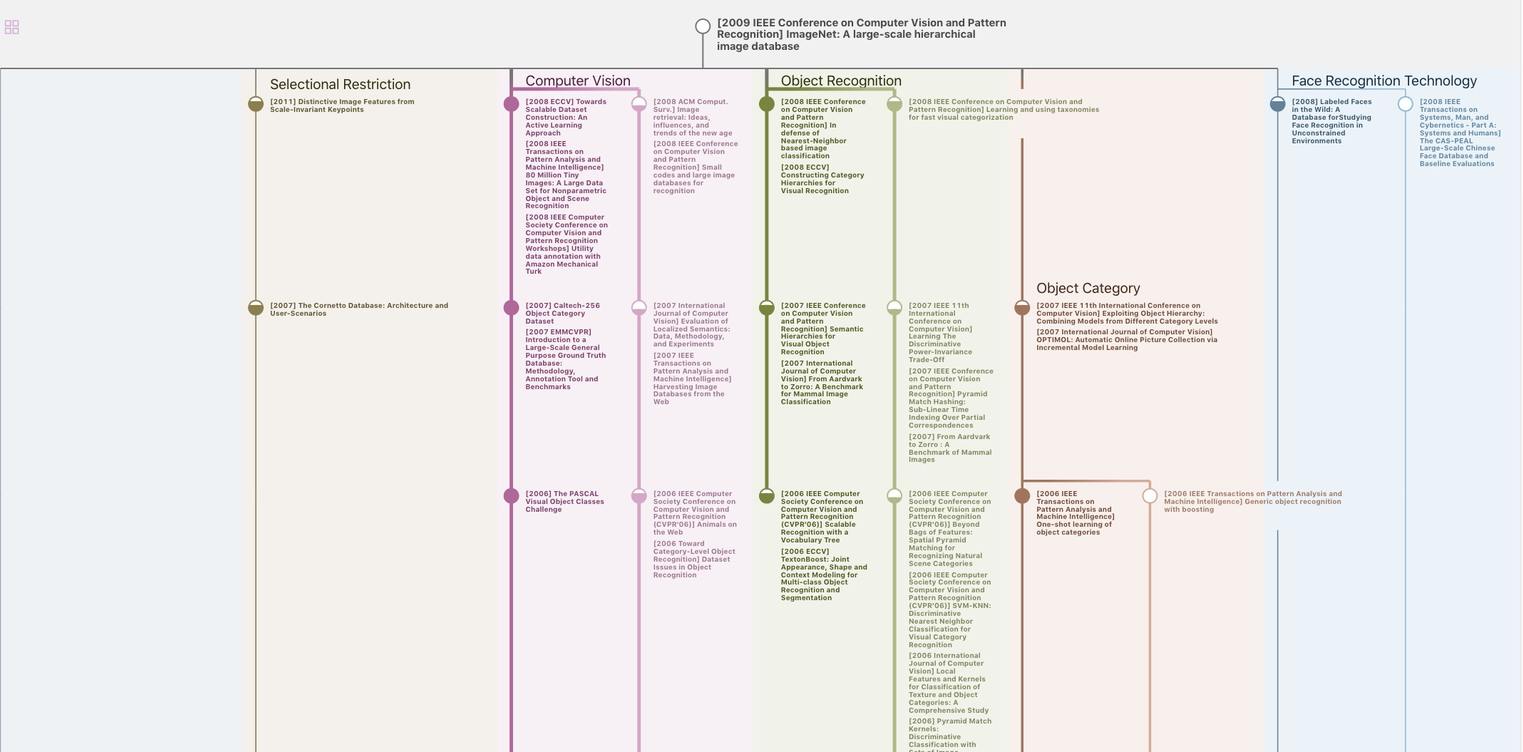
生成溯源树,研究论文发展脉络
Chat Paper
正在生成论文摘要