On the Validation of Gibbs Algorithms: Training Datasets, Test Datasets and their Aggregation
CoRR(2023)
摘要
The dependence on training data of the Gibbs algorithm (GA) is analytically characterized. By adopting the expected empirical risk as the performance metric, the sensitivity of the GA is obtained in closed form. In this case, sensitivity is the performance difference with respect to an arbitrary alternative algorithm. This description enables the development of explicit expressions involving the training errors and test errors of GAs trained with different datasets. Using these tools, dataset aggregation is studied and different figures of merit to evaluate the generalization capabilities of GAs are introduced. For particular sizes of such datasets and parameters of the GAs, a connection between Jeffrey’s divergence, training and test errors is established.
更多查看译文
关键词
gibbs algorithms,training datasets,test datasets,validation
AI 理解论文
溯源树
样例
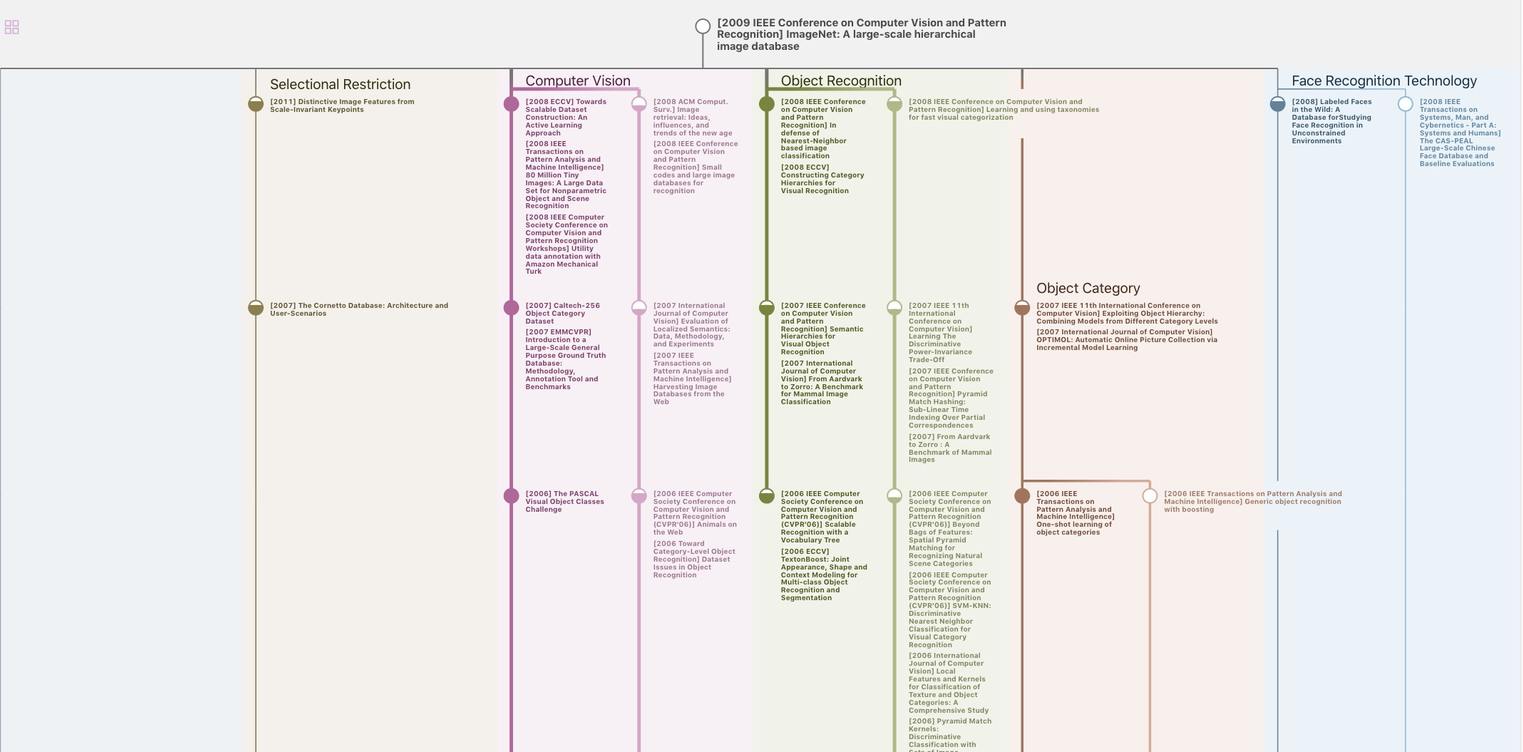
生成溯源树,研究论文发展脉络
Chat Paper
正在生成论文摘要