Automated Machine Learning for Remaining Useful Life Predictions
CoRR(2023)
摘要
Being able to predict the remaining useful life (RUL) of an engineering system is an important task in prognostics and health management. Recently, data-driven approaches to RUL predictions are becoming prevalent over model-based approaches since no underlying physical knowledge of the engineering system is required. Yet, this just replaces required expertise of the underlying physics with machine learning (ML) expertise, which is often also not available. Automated machine learning (AutoML) promises to build end-to-end ML pipelines automatically enabling domain experts without ML expertise to create their own models. This paper introduces AutoRUL, an AutoML-driven end-to-end approach for automatic RUL predictions. AutoRUL combines fine-tuned standard regression methods to an ensemble with high predictive power. By evaluating the proposed method on eight real-world and synthetic datasets against state-of-the-art hand-crafted models, we show that AutoML provides a viable alternative to hand-crafted data-driven RUL predictions. Consequently, creating RUL predictions can be made more accessible for domain experts using AutoML by eliminating ML expertise from data-driven model construction.
更多查看译文
关键词
Remaining Useful Life,Automated Machine Learning,data-driven,RUL,AutoML,PHM,ML
AI 理解论文
溯源树
样例
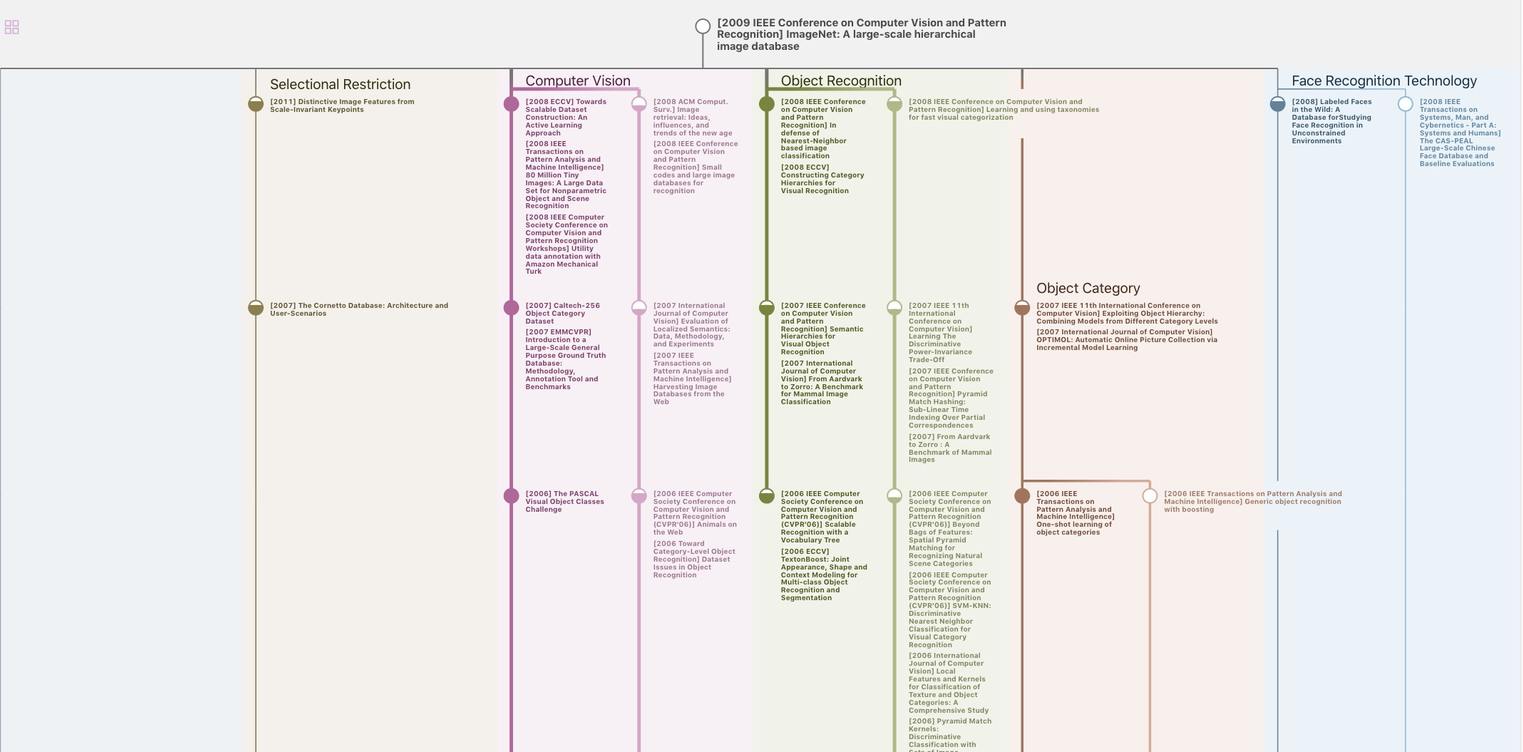
生成溯源树,研究论文发展脉络
Chat Paper
正在生成论文摘要