Sample Attackability in Natural Language Adversarial Attacks
CoRR(2023)
摘要
Adversarial attack research in natural language processing (NLP) has made significant progress in designing powerful attack methods and defence approaches. However, few efforts have sought to identify which source samples are the most attackable or robust, i.e. can we determine for an unseen target model, which samples are the most vulnerable to an adversarial attack. This work formally extends the definition of sample attackability/robustness for NLP attacks. Experiments on two popular NLP datasets, four state of the art models and four different NLP adversarial attack methods, demonstrate that sample uncertainty is insufficient for describing characteristics of attackable/robust samples and hence a deep learning based detector can perform much better at identifying the most attackable and robust samples for an unseen target model. Nevertheless, further analysis finds that there is little agreement in which samples are considered the most attackable/robust across different NLP attack methods, explaining a lack of portability of attackability detection methods across attack methods.
更多查看译文
关键词
natural language,sample
AI 理解论文
溯源树
样例
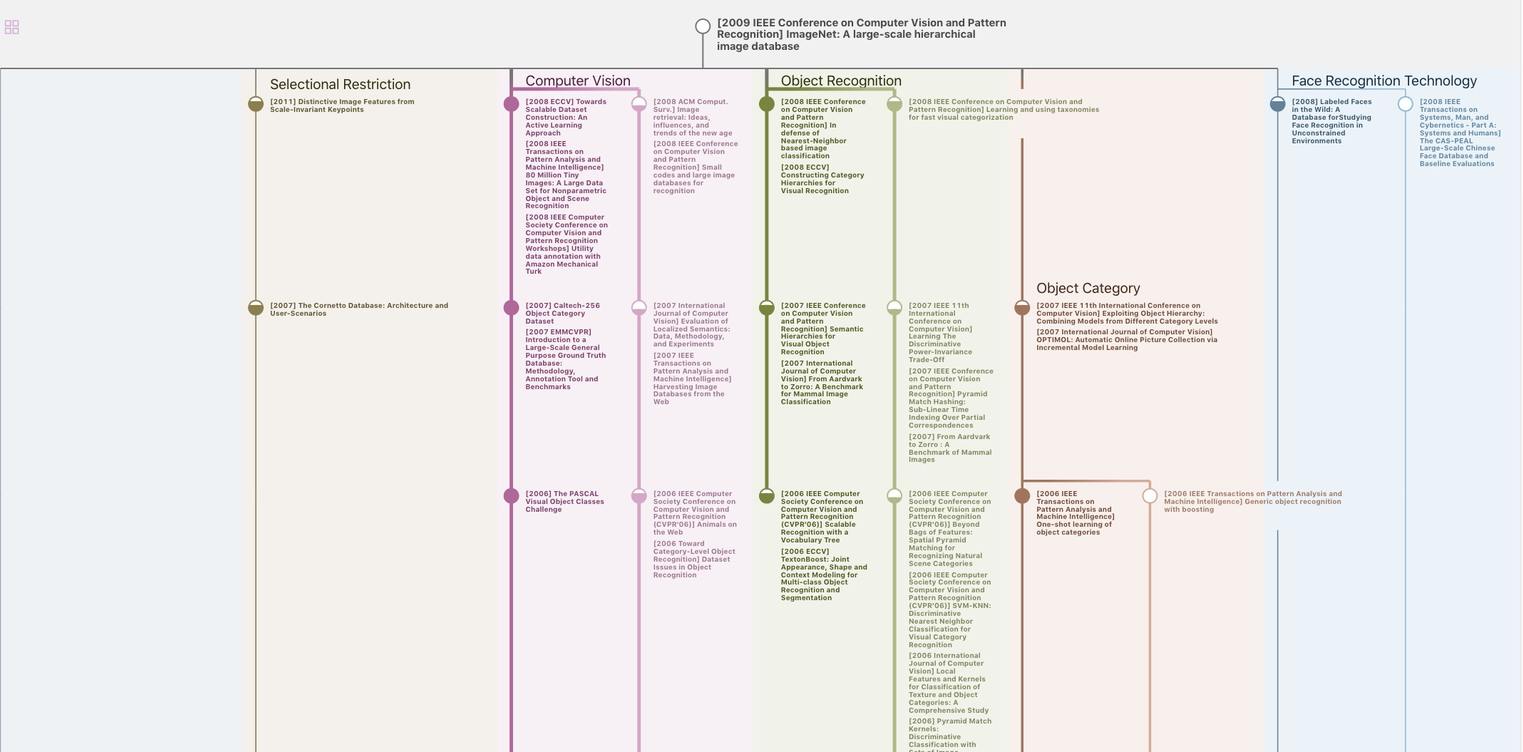
生成溯源树,研究论文发展脉络
Chat Paper
正在生成论文摘要