Towards Understanding What Code Language Models Learned
CoRR(2023)
摘要
Pre-trained language models are effective in a variety of natural language tasks, but it has been argued their capabilities fall short of fully learning meaning or understanding language. To understand the extent to which language models can learn some form of meaning, we investigate their ability to capture semantics of code beyond superficial frequency and co-occurrence. In contrast to previous research on probing models for linguistic features, we study pre-trained models in a setting that allows for objective and straightforward evaluation of a model's ability to learn semantics. In this paper, we examine whether such models capture the semantics of code, which is precisely and formally defined. Through experiments involving the manipulation of code fragments, we show that code pre-trained models of code learn a robust representation of the computational semantics of code that goes beyond superficial features of form alone
更多查看译文
关键词
code language models,understanding
AI 理解论文
溯源树
样例
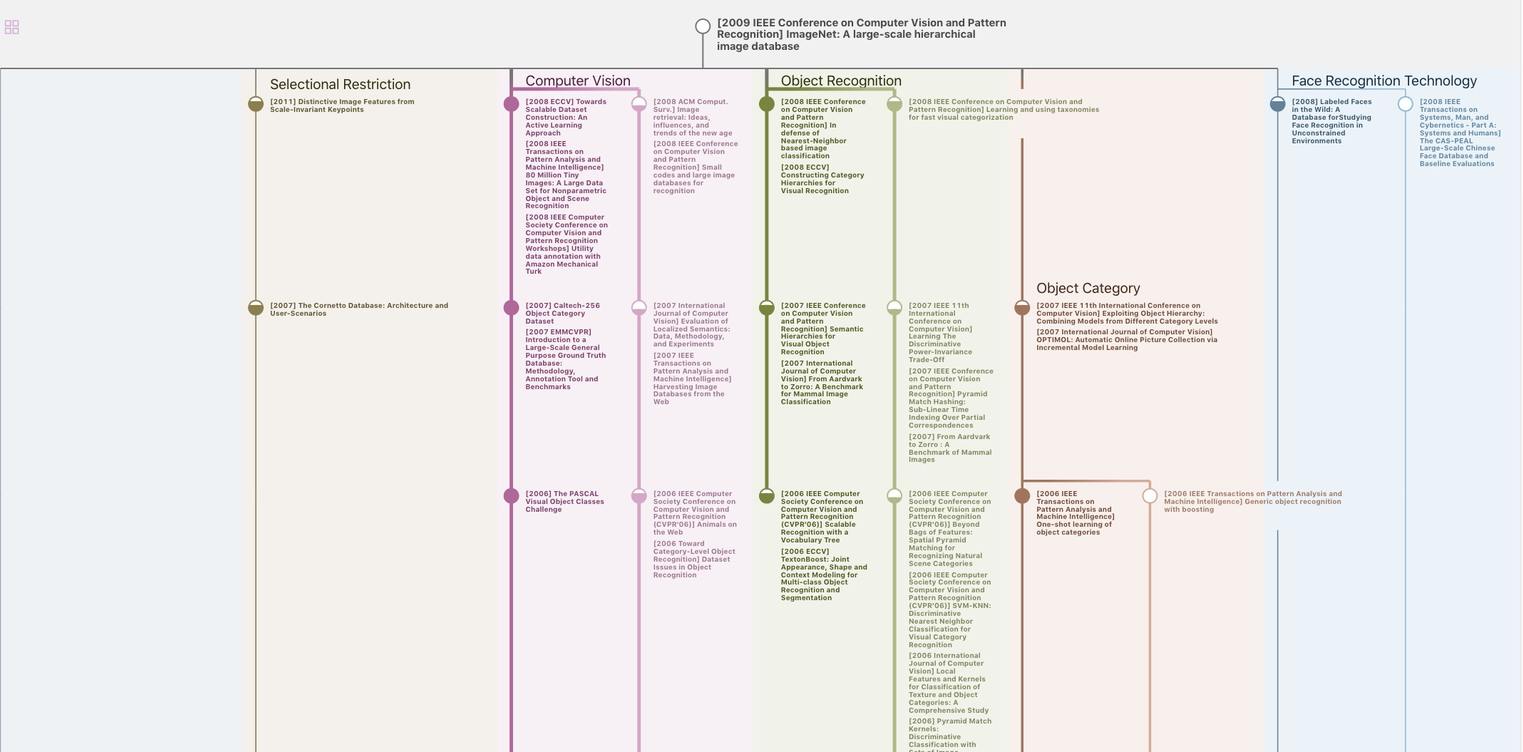
生成溯源树,研究论文发展脉络
Chat Paper
正在生成论文摘要