Simulating Temporally and Spatially Correlated Wind Speed Time Series by Spectral Representation Method
Complex System Modeling and Simulation(2023)
摘要
In this paper, it aims to model wind speed time series at multiple sites. The five-parameter Johnson distribution is deployed to relate the wind speed at each site to a Gaussian time series, and the resultant m- dimensional Gaussian stochastic vector process
$\boldsymbol{Z}(t)$
is employed to model the temporal-spatial correlation of wind speeds at
$m$
different sites.
$\ln$
general, it is computationally tedious to obtain the autocorrelation functions (ACFs) and cross-correlation functions (CCFs) of
$\boldsymbol{Z}(t)$
, which are different to those of wind speed times series. In order to circumvent this correlation distortion problem, the rank ACF and rank CCF are introduced to characterize the temporal-spatial correlation of wind speeds, whereby the ACFs and CCFs of
$\boldsymbol{Z}(t)$
can be analytically obtained.
$\text{Then}$
, Fourier transformation is implemented to establish the cross-spectral density matrix of
$\boldsymbol{Z}(t)$
, and an analytical approach is proposed to generate samples of wind speeds at
$m$
different sites. Finally, simulation experiments are performed to check the proposed methods, and the results verify that the five-parameter Johnson distribution can accurately match distribution functions of wind speeds, and the spectral representation method can well reproduce the temporal-spatial correlation of wind speeds.
更多查看译文
关键词
multivariate wind speed time series,rank autocorrelation function,rank cross-correlation function,cross-spectral density matrix,five-parameter johnson distribution
AI 理解论文
溯源树
样例
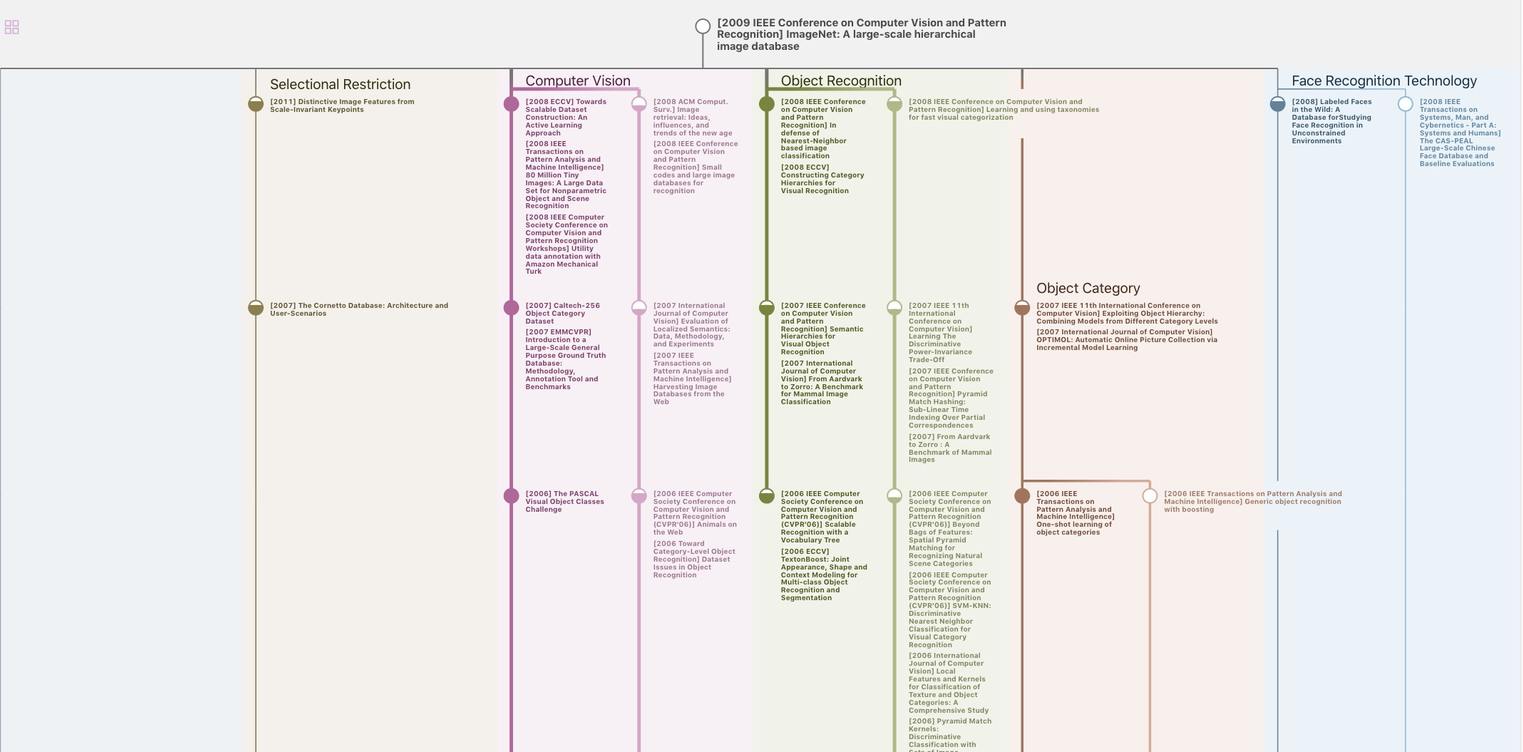
生成溯源树,研究论文发展脉络
Chat Paper
正在生成论文摘要