Annotation cost efficient active learning for content based image retrieval
IGARSS 2023 - 2023 IEEE INTERNATIONAL GEOSCIENCE AND REMOTE SENSING SYMPOSIUM(2023)
摘要
Deep metric learning (DML) based methods have been found very effective for content-based image retrieval (CBIR) in remote sensing (RS). For accurately learning the model parameters of deep neural networks, most of the DML methods require a high number of annotated training images, which can be costly to gather. To address this problem, in this paper we present an annotation cost efficient active learning (AL) method (denoted as ANNEAL). The proposed method aims to iteratively enrich the training set by annotating the most informative image pairs as similar or dissimilar, while accurately modelling a deep metric space. This is achieved by two consecutive steps. In the first step the pairwise image similarity is modelled based on the available training set. Then, in the second step the most uncertain and diverse (i.e., informative) image pairs are selected to be annotated. Unlike the existing AL methods for CBIR, at each AL iteration of ANNEAL a human expert is asked to annotate the most informative image pairs as similar/dissimilar. This significantly reduces the annotation cost compared to annotating images with land-use/land cover class labels. Experimental results show the effectiveness of our method. The code of ANNEAL is publicly available at https://git.tu-berlin.de/rsim/ANNEAL.
更多查看译文
关键词
Active learning,content based image retrieval,deep metric learning,remote sensing
AI 理解论文
溯源树
样例
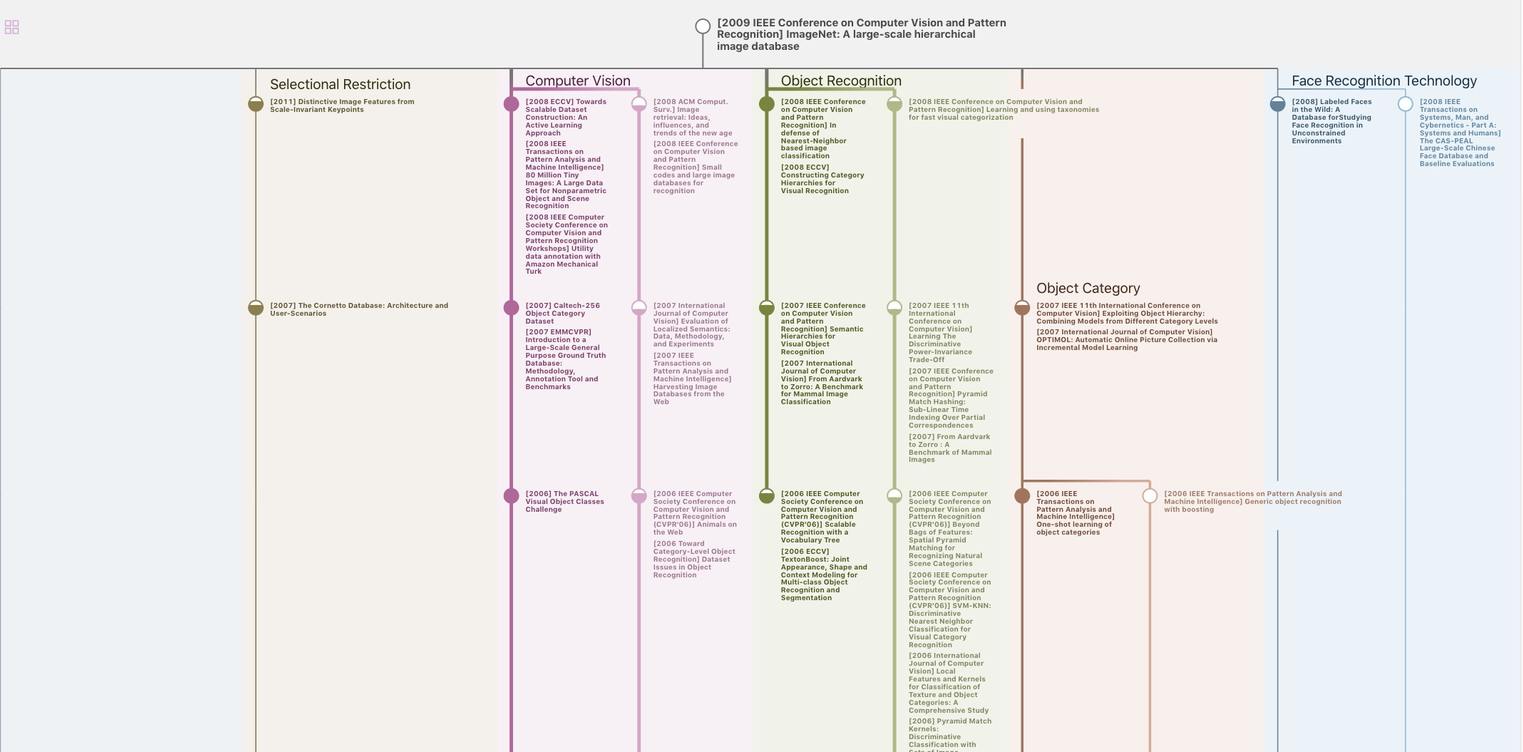
生成溯源树,研究论文发展脉络
Chat Paper
正在生成论文摘要