3D Keypoint Estimation Using Implicit Representation Learning
Comput. Graph. Forum(2023)
摘要
In this paper, we tackle the challenging problem of 3D keypoint estimation of general objects using a novel implicit representation. Previous works have demonstrated promising results for keypoint prediction through direct coordinate regression or heatmap-based inference. However, these methods are commonly studied for specific subjects, such as human bodies and faces, which possess fixed keypoint structures. They also suffer in several practical scenarios where explicit or complete geometry is not given, including images and partial point clouds. Inspired by the recent success of advanced implicit representation in reconstruction tasks, we explore the idea of using an implicit field to represent keypoints. Specifically, our key idea is employing spheres to represent 3D keypoints, thereby enabling the learnability of the corresponding signed distance field. Explicit keypoints can be extracted subsequently by our algorithm based on the Hough transform. Quantitative and qualitative evaluations also show the superiority of our representation in terms of prediction accuracy.
更多查看译文
关键词
3d
AI 理解论文
溯源树
样例
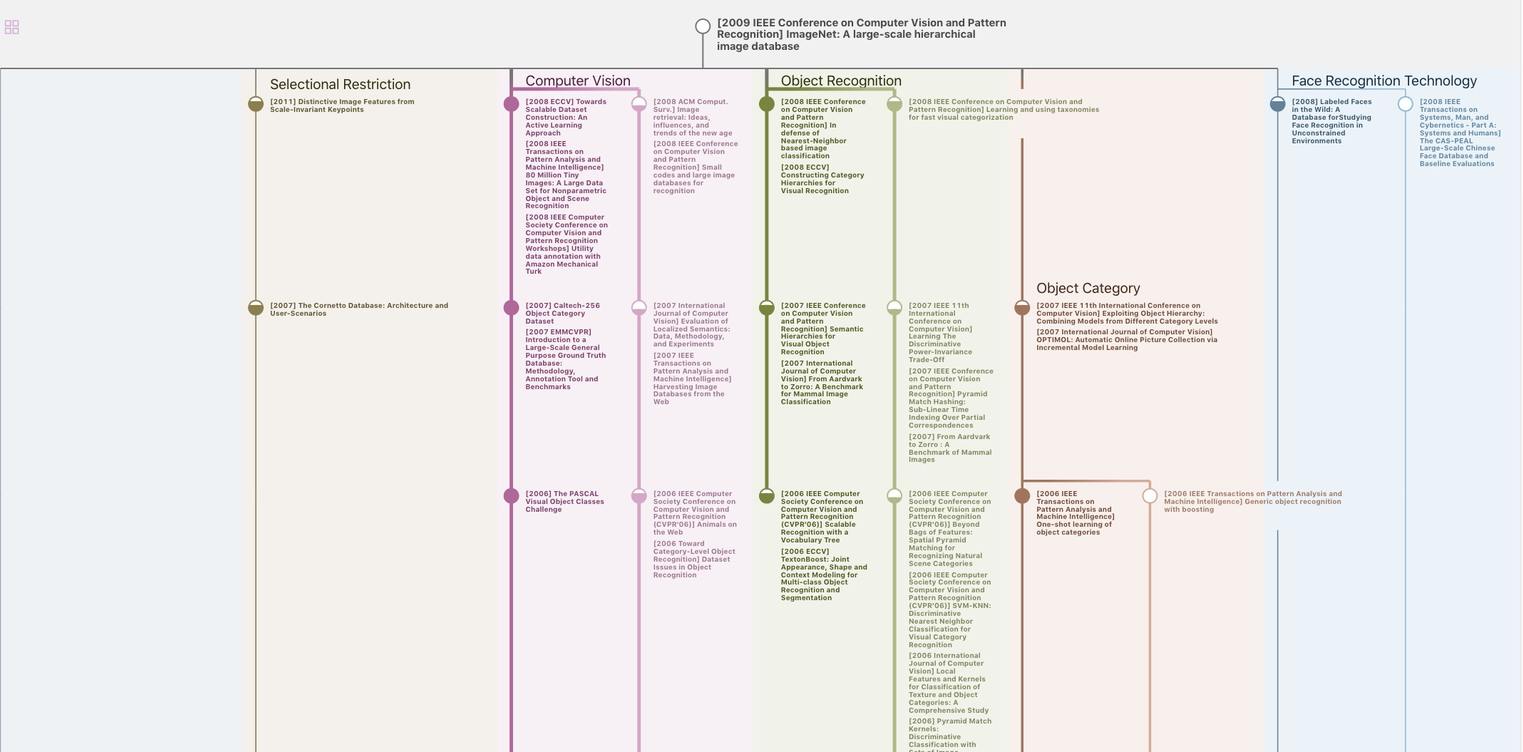
生成溯源树,研究论文发展脉络
Chat Paper
正在生成论文摘要