Contrastive Disentangled Learning on Graph for Node Classification
CoRR(2023)
摘要
Contrastive learning methods have attracted considerable attention due to their remarkable success in analyzing graph-structured data. Inspired by the success of contrastive learning, we propose a novel framework for contrastive disentangled learning on graphs, employing a disentangled graph encoder and two carefully crafted self-supervision signals. Specifically, we introduce a disentangled graph encoder to enforce the framework to distinguish various latent factors corresponding to underlying semantic information and learn the disentangled node embeddings. Moreover, to overcome the heavy reliance on labels, we design two self-supervision signals, namely node specificity and channel independence, which capture informative knowledge without the need for labeled data, thereby guiding the automatic disentanglement of nodes. Finally, we perform node classification tasks on three citation networks by using the disentangled node embeddings, and the relevant analysis is provided. Experimental results validate the effectiveness of the proposed framework compared with various baselines.
更多查看译文
关键词
graph,classification
AI 理解论文
溯源树
样例
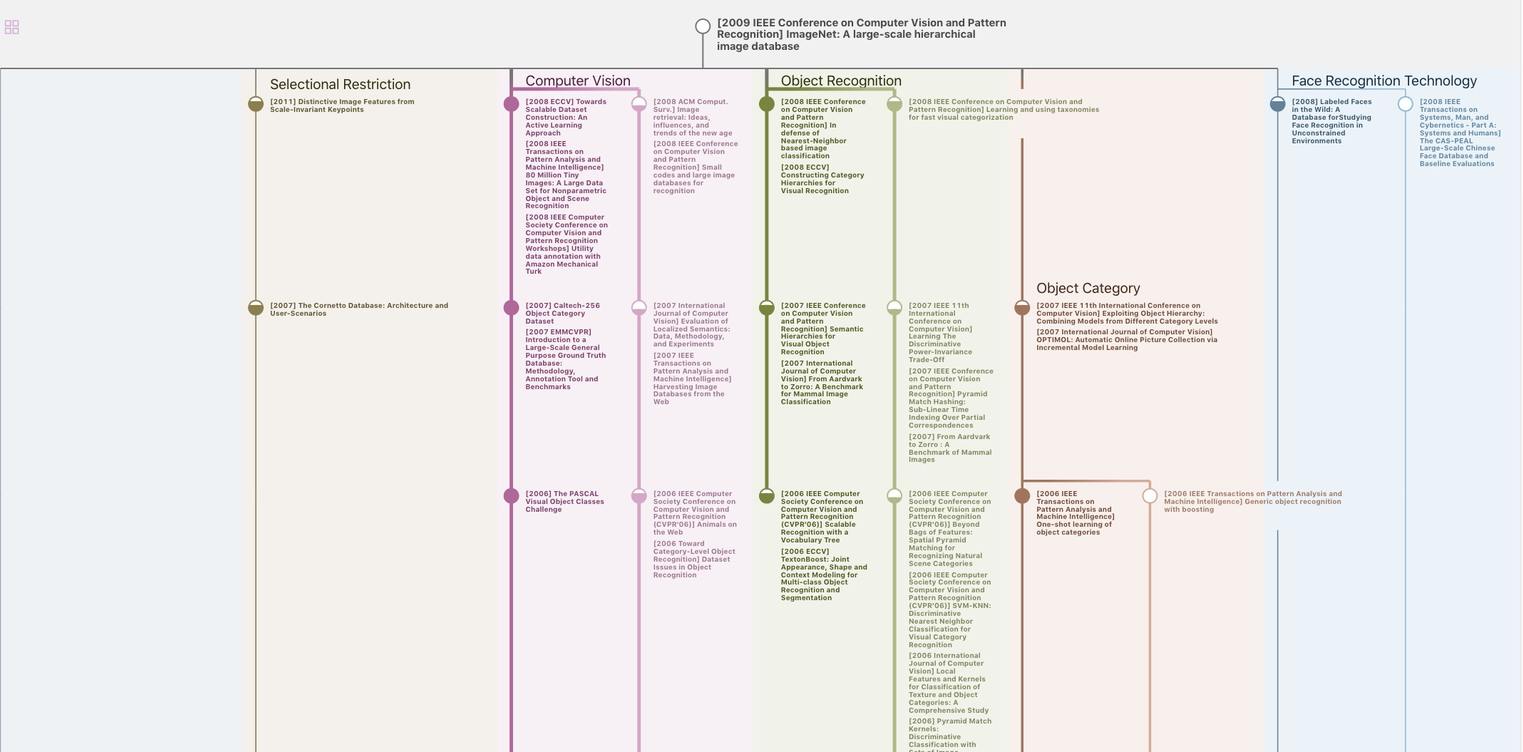
生成溯源树,研究论文发展脉络
Chat Paper
正在生成论文摘要