Cooperative Multi-Agent Learning for Navigation via Structured State Abstraction
CoRR(2023)
摘要
Cooperative multi-agent reinforcement learning (MARL) for navigation enables
agents to cooperate to achieve their navigation goals. Using emergent
communication, agents learn a communication protocol to coordinate and share
information that is needed to achieve their navigation tasks. In emergent
communication, symbols with no pre-specified usage rules are exchanged, in
which the meaning and syntax emerge through training. Learning a navigation
policy along with a communication protocol in a MARL environment is highly
complex due to the huge state space to be explored. To cope with this
complexity, this work proposes a novel neural network architecture, for jointly
learning an adaptive state space abstraction and a communication protocol among
agents participating in navigation tasks. The goal is to come up with an
adaptive abstractor that significantly reduces the size of the state space to
be explored, without degradation in the policy performance. Simulation results
show that the proposed method reaches a better policy, in terms of achievable
rewards, resulting in fewer training iterations compared to the case where raw
states or fixed state abstraction are used. Moreover, it is shown that a
communication protocol emerges during training which enables the agents to
learn better policies within fewer training iterations.
更多查看译文
关键词
Reinforcement learning,emergent communication,multiagent,state abstraction,structure,graph neural network,quadtree,adaptive
AI 理解论文
溯源树
样例
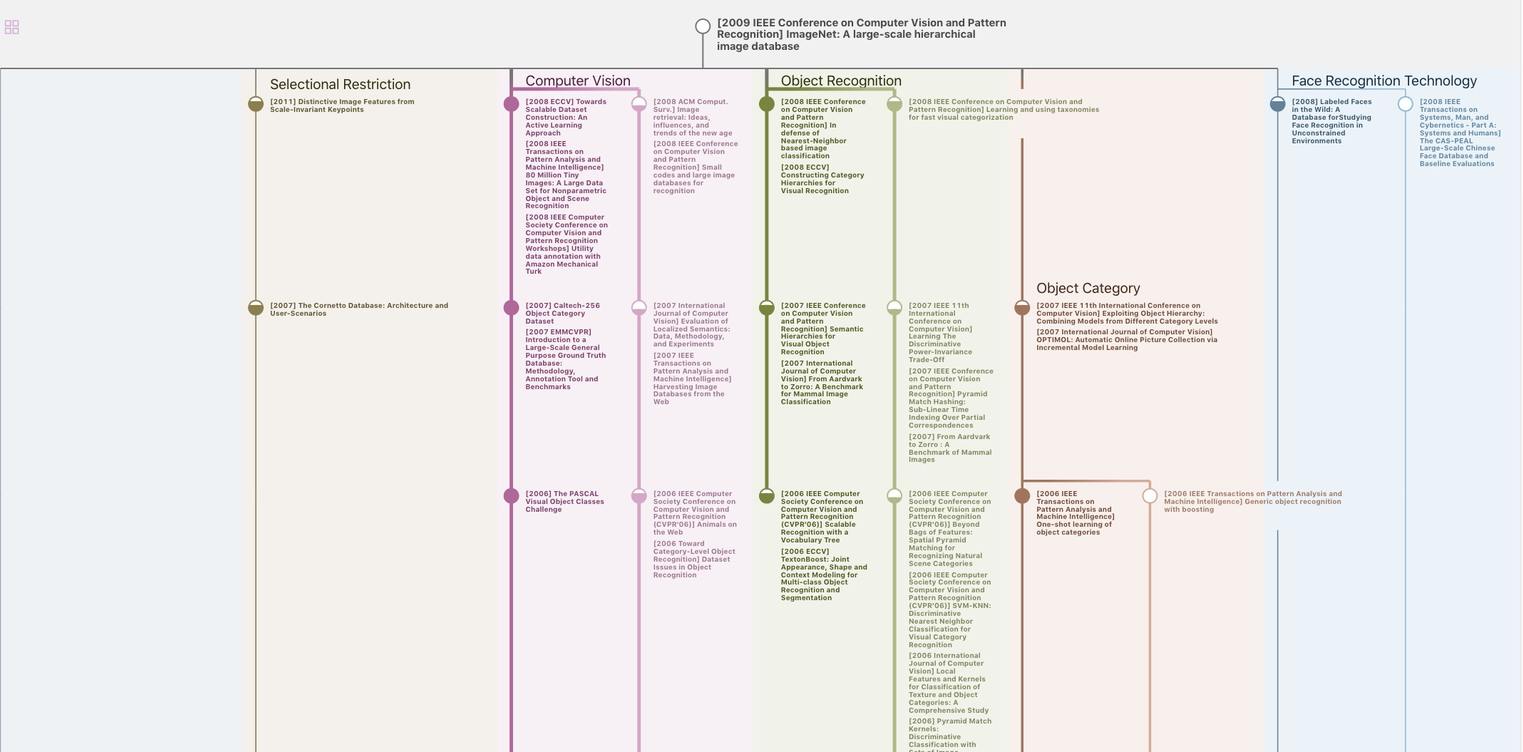
生成溯源树,研究论文发展脉络
Chat Paper
正在生成论文摘要