AVOIDDS: Aircraft Vision-based Intruder Detection Dataset and Simulator
arXiv (Cornell University)(2023)
摘要
Designing robust machine learning systems remains an open problem, and there is a need for benchmark problems that cover both environmental changes and evaluation on a downstream task. In this work, we introduce AVOIDDS, a realistic object detection benchmark for the vision-based aircraft detect-and-avoid problem. We provide a labeled dataset consisting of 72,000 photorealistic images of intruder aircraft with various lighting conditions, weather conditions, relative geometries, and geographic locations. We also provide an interface that evaluates trained models on slices of this dataset to identify changes in performance with respect to changing environmental conditions. Finally, we implement a fully-integrated, closed-loop simulator of the vision-based detect-and-avoid problem to evaluate trained models with respect to the downstream collision avoidance task. This benchmark will enable further research in the design of robust machine learning systems for use in safety-critical applications. The AVOIDDS dataset and code are publicly available at $\href{https://purl.stanford.edu/hj293cv5980}{purl.stanford.edu/hj293cv5980}$ and $\href{https://github.com/sisl/VisionBasedAircraftDAA}{github.com/sisl/VisionBasedAircraftDAA}$, respectively.
更多查看译文
关键词
intruder detection dataset,simulator,vision-based
AI 理解论文
溯源树
样例
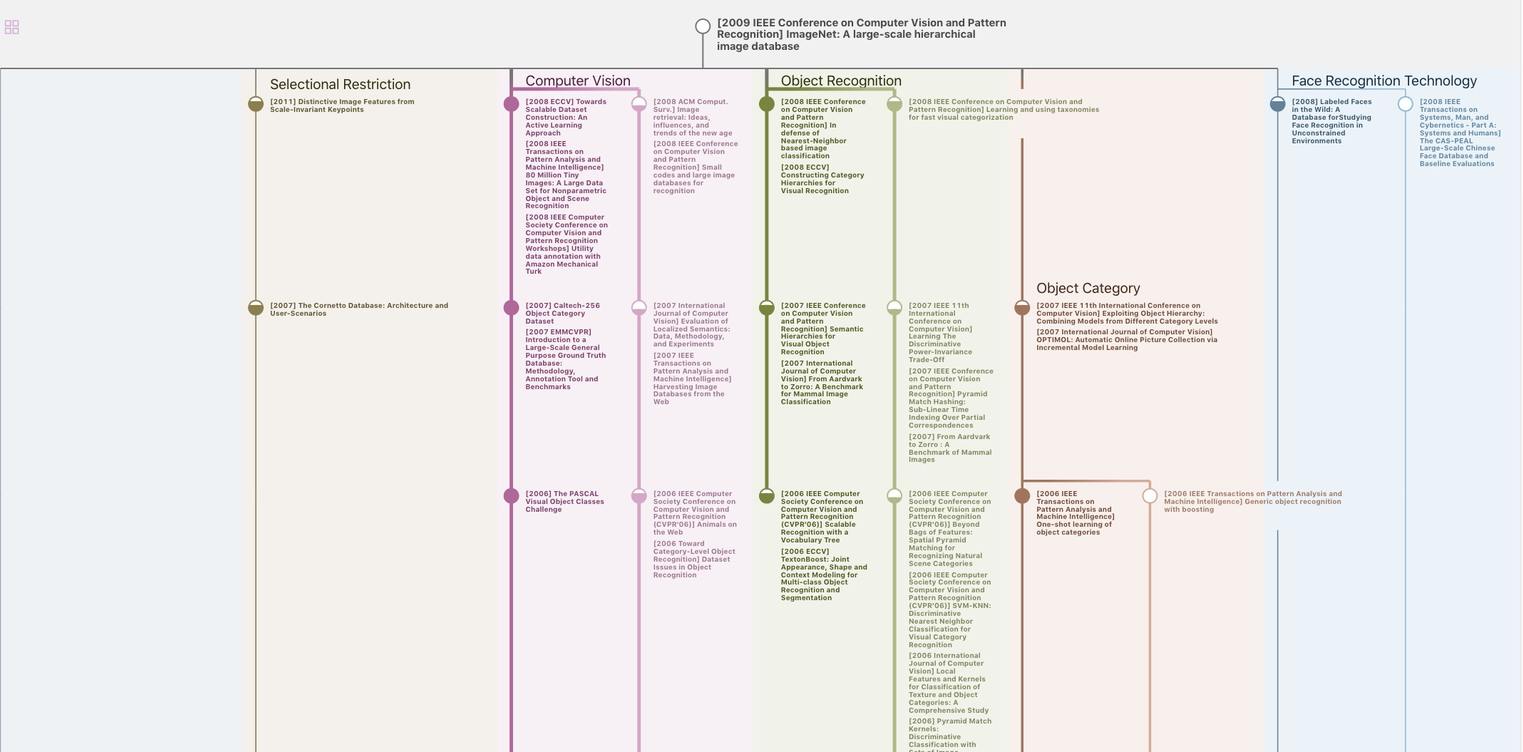
生成溯源树,研究论文发展脉络
Chat Paper
正在生成论文摘要