Diffusion model based data generation for partial differential equations
CoRR(2023)
摘要
In a preliminary attempt to address the problem of data scarcity in physics-based machine learning, we introduce a novel methodology for data generation in physics-based simulations. Our motivation is to overcome the limitations posed by the limited availability of numerical data. To achieve this, we leverage a diffusion model that allows us to generate synthetic data samples and test them for two canonical cases: (a) the steady 2-D Poisson equation, and (b) the forced unsteady 2-D Navier-Stokes (NS) {vorticity-transport} equation in a confined box. By comparing the generated data samples against outputs from classical solvers, we assess their accuracy and examine their adherence to the underlying physics laws. In this way, we emphasize the importance of not only satisfying visual and statistical comparisons with solver data but also ensuring the generated data's conformity to physics laws, thus enabling their effective utilization in downstream tasks.
更多查看译文
关键词
partial differential equations,data generation
AI 理解论文
溯源树
样例
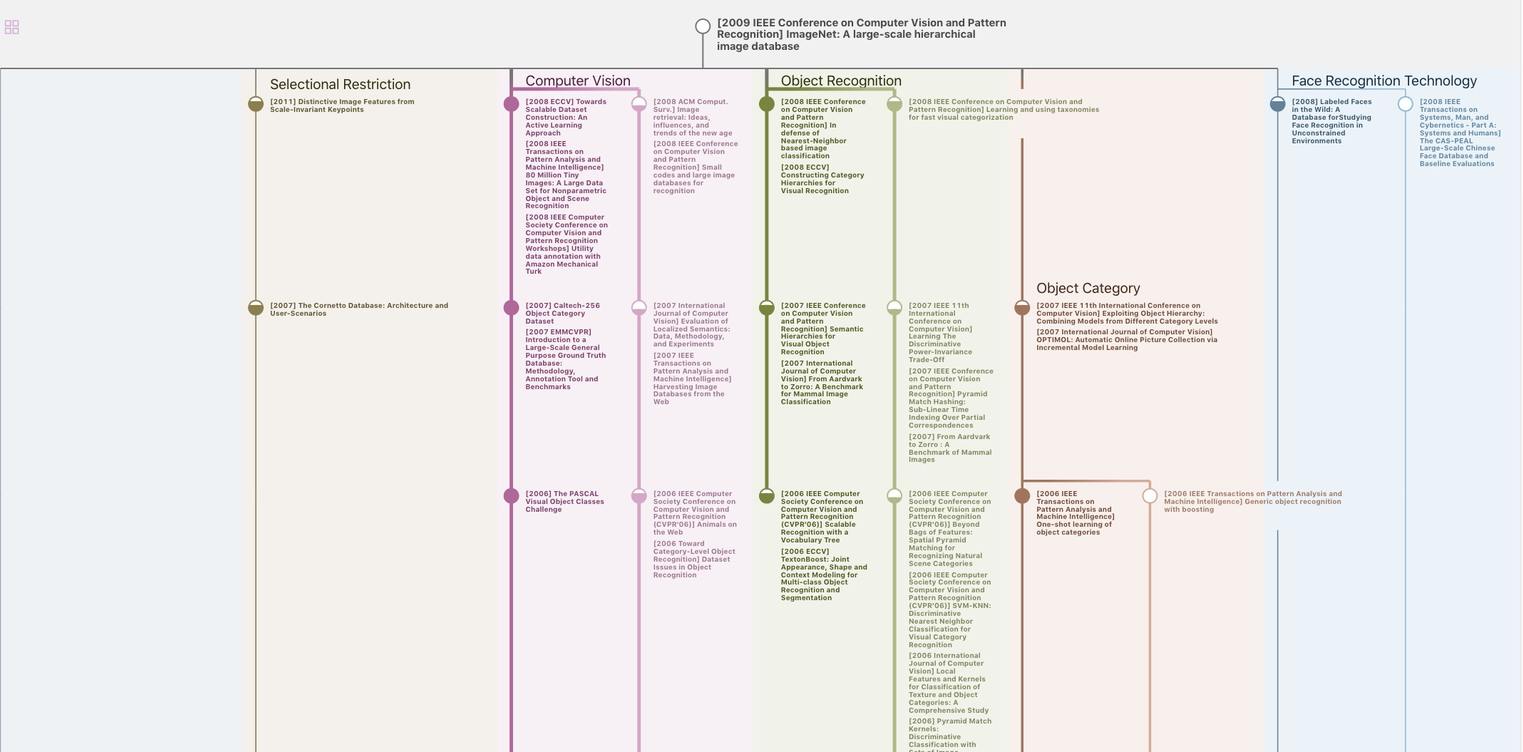
生成溯源树,研究论文发展脉络
Chat Paper
正在生成论文摘要