Simple and Fast Group Robustness by Automatic Feature Reweighting
CoRR(2023)
摘要
A major challenge to out-of-distribution generalization is reliance on spurious features -- patterns that are predictive of the class label in the training data distribution, but not causally related to the target. Standard methods for reducing the reliance on spurious features typically assume that we know what the spurious feature is, which is rarely true in the real world. Methods that attempt to alleviate this limitation are complex, hard to tune, and lead to a significant computational overhead compared to standard training. In this paper, we propose Automatic Feature Reweighting (AFR), an extremely simple and fast method for updating the model to reduce the reliance on spurious features. AFR retrains the last layer of a standard ERM-trained base model with a weighted loss that emphasizes the examples where the ERM model predicts poorly, automatically upweighting the minority group without group labels. With this simple procedure, we improve upon the best reported results among competing methods trained without spurious attributes on several vision and natural language classification benchmarks, using only a fraction of their compute.
更多查看译文
关键词
fast group robustness,automatic feature reweighting
AI 理解论文
溯源树
样例
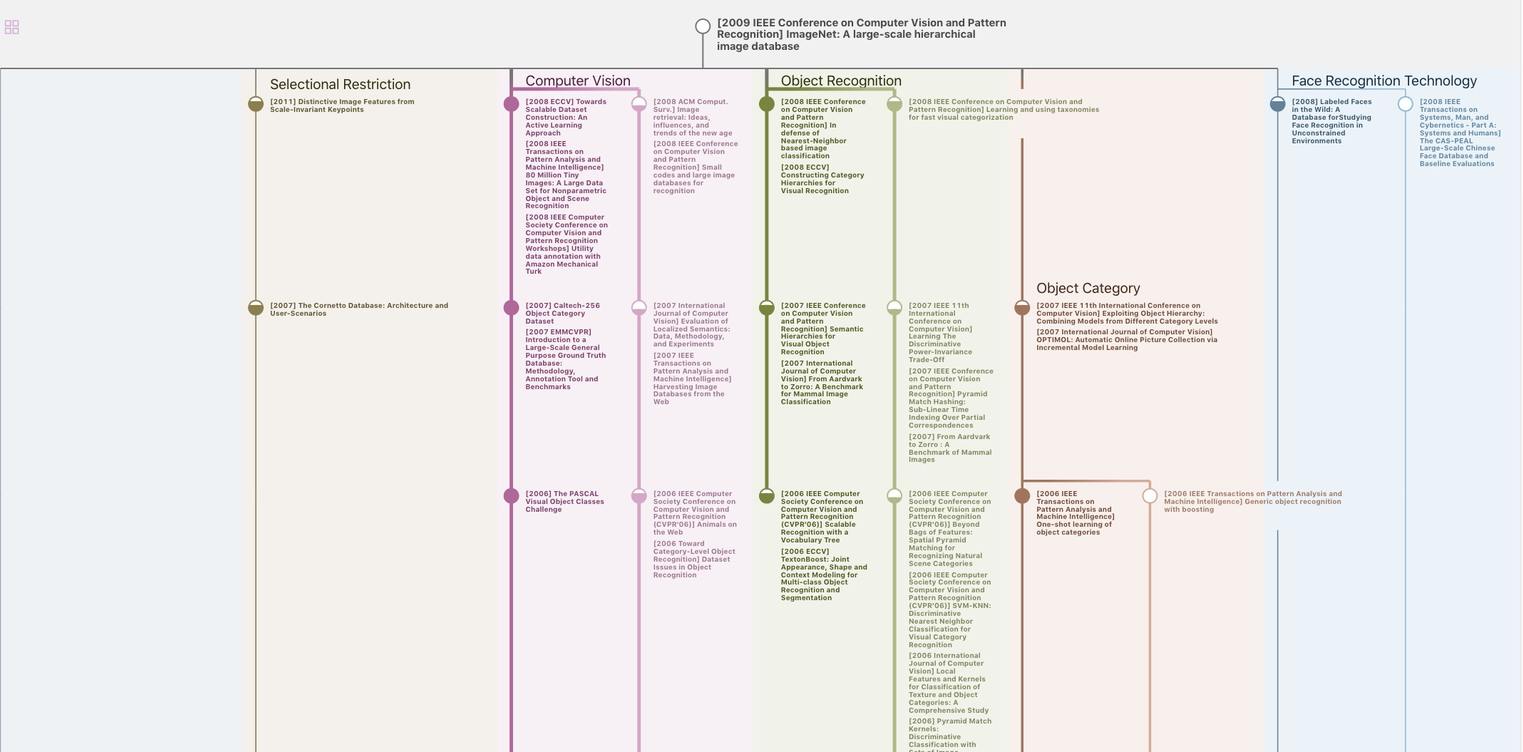
生成溯源树,研究论文发展脉络
Chat Paper
正在生成论文摘要