Adaptive Ordered Information Extraction with Deep Reinforcement Learning
conf_acl(2023)
摘要
Information extraction (IE) has been studied extensively. The existing methods always follow a fixed extraction order for complex IE tasks with multiple elements to be extracted in one instance such as event extraction. However, we conduct experiments on several complex IE datasets and observe that different extraction orders can significantly affect the extraction results for a great portion of instances, and the ratio of sentences that are sensitive to extraction orders increases dramatically with the complexity of the IE task. Therefore, this paper proposes a novel adaptive ordered IE paradigm to find the optimal element extraction order for different instances, so as to achieve the best extraction results. We also propose an reinforcement learning (RL) based framework to generate optimal extraction order for each instance dynamically. Additionally, we propose a co-training framework adapted to RL to mitigate the exposure bias during the extractor training phase. Extensive experiments conducted on several public datasets demonstrate that our proposed method can beat previous methods and effectively improve the performance of various IE tasks, especially for complex ones.
更多查看译文
关键词
extraction,reinforcement,information,learning
AI 理解论文
溯源树
样例
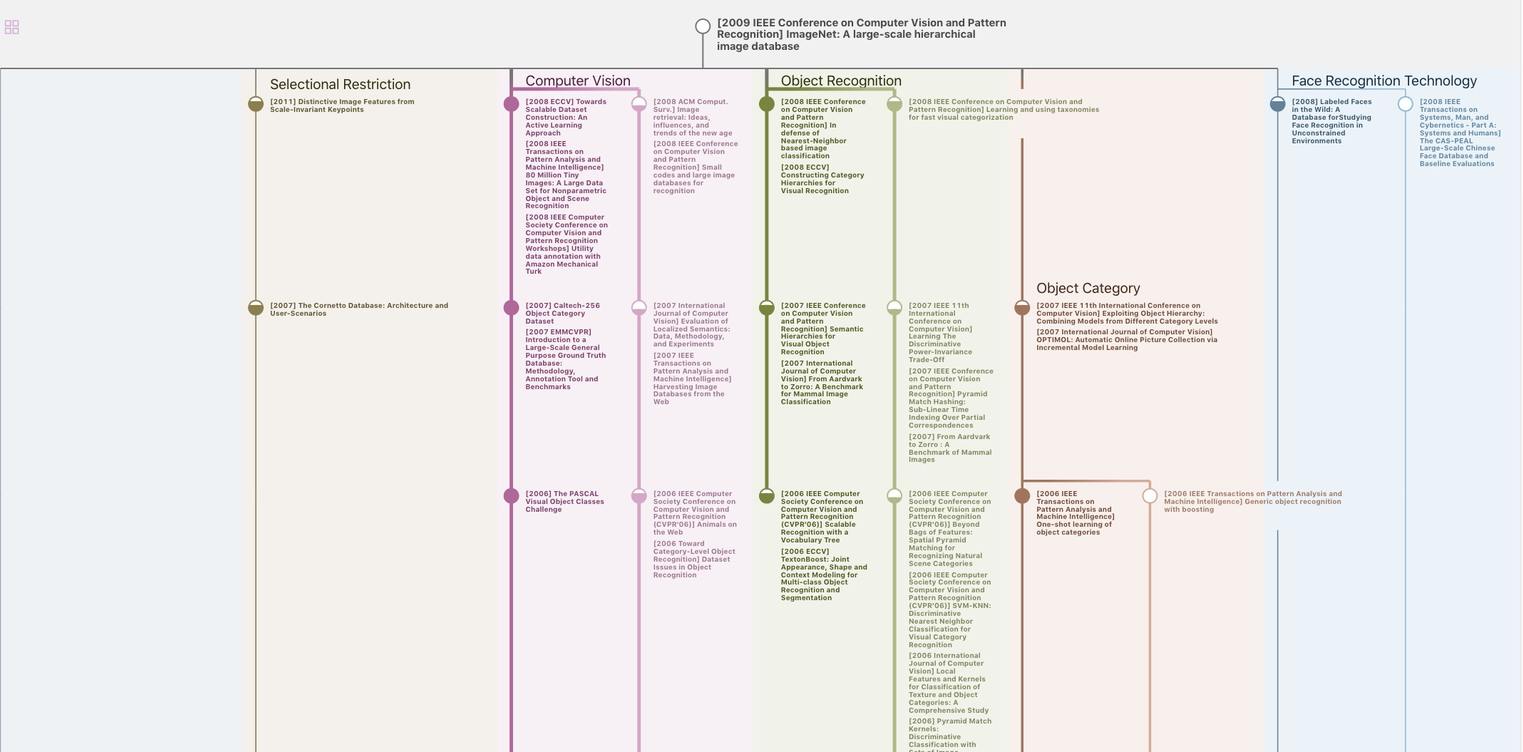
生成溯源树,研究论文发展脉络
Chat Paper
正在生成论文摘要