Metasurface-based Spectral Convolutional Neural Network for Matter Meta-imaging
CoRR(2023)
摘要
Convolutional neural networks (CNNs) are representative models of artificial neural networks (ANNs), that form the backbone of modern computer vision. However, the considerable power consumption and limited computing speed of electrical computing platforms restrict further development of CNNs. Optical neural networks are considered the next-generation physical implementations of ANNs to break the bottleneck. This study proposes a spectral convolutional neural network (SCNN) with the function of matter meta-imaging, namely identifying the composition of matter and mapping its distribution in space. This SCNN includes an optical convolutional layer (OCL) and a reconfigurable electrical backend. The OCL is implemented by integrating very large-scale, pixel-aligned metasurfaces on a CMOS image sensor, which accepts 3D raw datacubes of natural images, containing two-spatial and one-spectral dimensions, at megapixels directly as input to realize the matter meta-imaging. This unique optoelectronic framework empowers in-sensor optical analog computing at extremely high energy efficiency eliminating the need for coherent light sources and greatly reducing the computing load of the electrical backend. We employed the SCNN framework on several real-world complex tasks. It achieved accuracies of 96.4% and 100% for pathological diagnosis and real-time face anti-spoofing at video rate, respectively. The SCNN framework, with an unprecedented new function of substance identification, provides a feasible optoelectronic and integrated optical CNN implementation for edge devices or cellphones with limited computing capabilities, facilitating diverse applications, such as intelligent robotics, industrial automation, medical diagnosis, and astronomy.
更多查看译文
关键词
spectral convolutional neural network,neural network,metasurface-based,meta-imaging
AI 理解论文
溯源树
样例
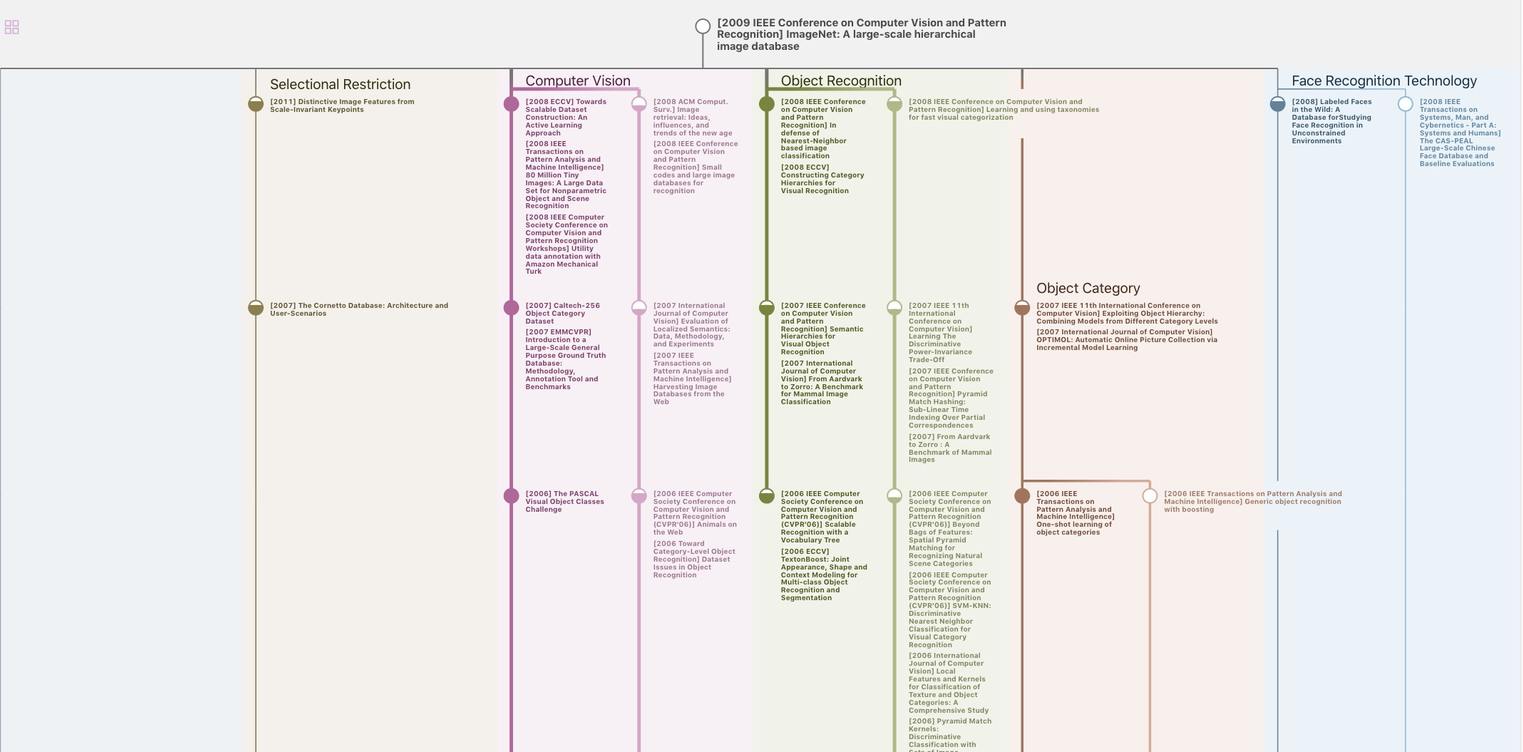
生成溯源树,研究论文发展脉络
Chat Paper
正在生成论文摘要