DuTa-VC: A Duration-aware Typical-to-atypical Voice Conversion Approach with Diffusion Probabilistic Model
arXiv (Cornell University)(2023)
摘要
We present a novel typical-to-atypical voice conversion approach (DuTa-VC), which (i) can be trained with nonparallel data (ii) first introduces diffusion probabilistic model (iii) preserves the target speaker identity (iv) is aware of the phoneme duration of the target speaker. DuTa-VC consists of three parts: an encoder transforms the source mel-spectrogram into a duration-modified speaker-independent mel-spectrogram, a decoder performs the reverse diffusion to generate the target mel-spectrogram, and a vocoder is applied to reconstruct the waveform. Objective evaluations conducted on the UASpeech show that DuTa-VC is able to capture severity characteristics of dysarthric speech, reserves speaker identity, and significantly improves dysarthric speech recognition as a data augmentation. Subjective evaluations by two expert speech pathologists validate that DuTa-VC can preserve the severity and type of dysarthria of the target speakers in the synthesized speech.
更多查看译文
关键词
conversion,diffusion,duration-aware,typical-to-atypical
AI 理解论文
溯源树
样例
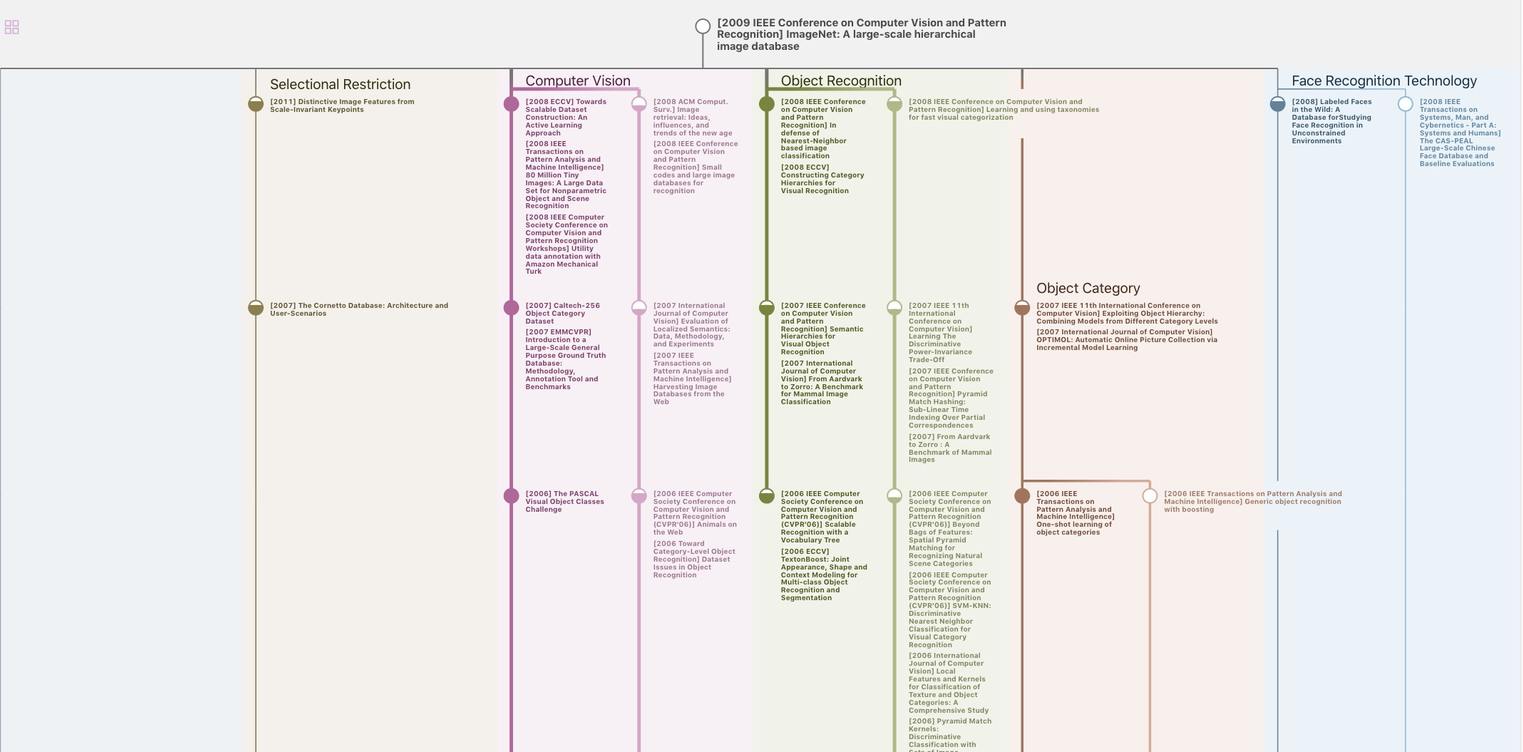
生成溯源树,研究论文发展脉络
Chat Paper
正在生成论文摘要