UniMC: A Unified Framework for Long-Term Memory Conversation via Relevance Representation Learning
CoRR(2023)
摘要
Open-domain long-term memory conversation can establish long-term intimacy with humans, and the key is the ability to understand and memorize long-term dialogue history information. Existing works integrate multiple models for modelling through a pipeline, which ignores the coupling between different stages. In this paper, we propose a Unified framework for Long-term Memory Conversations (UniMC), which increases the connection between different stages by learning relevance representation. Specifically, we decompose the main task into three subtasks based on probability graphs: 1) conversation summarization, 2) memory retrieval, 3) memory-augmented generation. Each subtask involves learning a representation for calculating the relevance between the query and memory, which is modelled by inserting a special token at the beginning of the decoder input. The relevance representation learning strengthens the connection across subtasks through parameter sharing and joint training. Extensive experimental results show that the proposed method consistently improves over strong baselines and yields better dialogue consistency and engagingness.
更多查看译文
关键词
relevance representation learning,memory,long-term
AI 理解论文
溯源树
样例
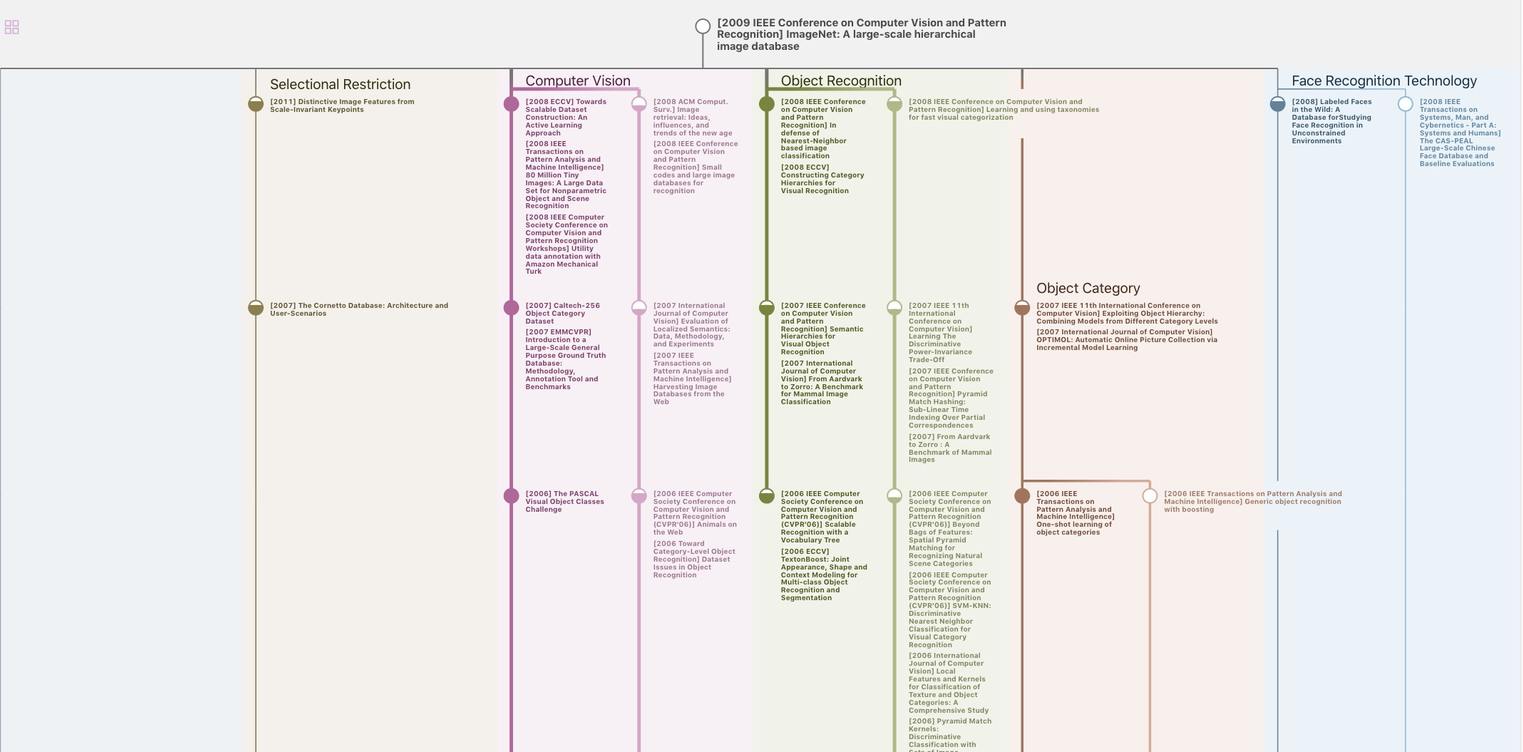
生成溯源树,研究论文发展脉络
Chat Paper
正在生成论文摘要