Deep Reinforcement Learning for Flipper Control of Tracked Robots
CoRR(2023)
摘要
The autonomous control of flippers plays an important role in enhancing the intelligent operation of tracked robots within complex environments. While existing methods mainly rely on hand-crafted control models, in this paper, we introduce a novel approach that leverages deep reinforcement learning (DRL) techniques for autonomous flipper control in complex terrains. Specifically, we propose a new DRL network named AT-D3QN, which ensures safe and smooth flipper control for tracked robots. It comprises two modules, a feature extraction and fusion module for extracting and integrating robot and environment state features, and a deep Q-Learning control generation module for incorporating expert knowledge to obtain a smooth and efficient control strategy. To train the network, a novel reward function is proposed, considering both learning efficiency and passing smoothness. A simulation environment is constructed using the Pymunk physics engine for training. We then directly apply the trained model to a more realistic Gazebo simulation for quantitative analysis. The consistently high performance of the proposed approach validates its superiority over manual teleoperation.
更多查看译文
关键词
deep reinforcement learning,flipper control
AI 理解论文
溯源树
样例
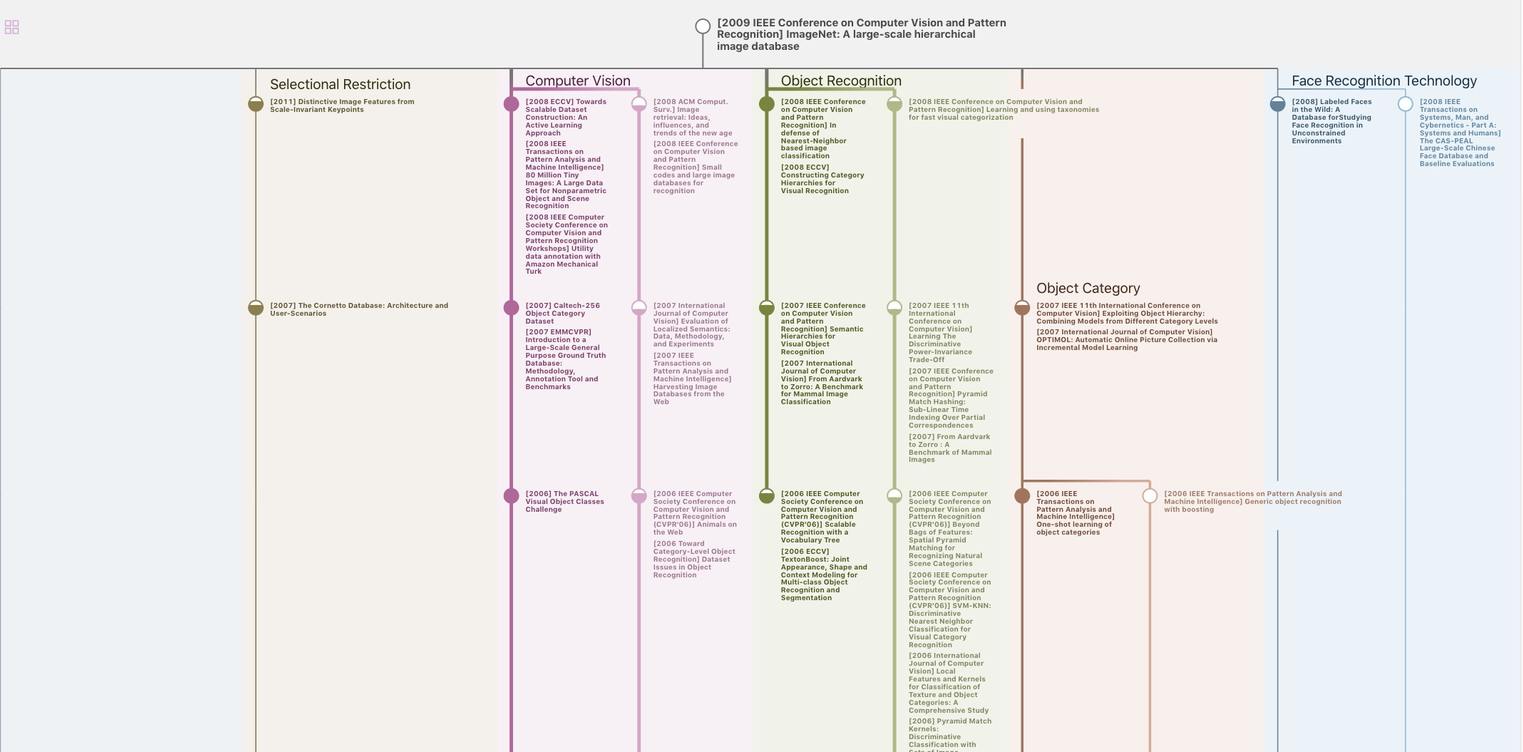
生成溯源树,研究论文发展脉络
Chat Paper
正在生成论文摘要