Learning-Augmented Decentralized Online Convex Optimization in Networks
CoRR(2023)
摘要
This paper studies decentralized online convex optimization in a networked multi-agent system and proposes a novel algorithm, Learning-Augmented Decentralized Online optimization (LADO), for individual agents to select actions only based on local online information. LADO leverages a baseline policy to safeguard online actions for worst-case robustness guarantees, while staying close to the machine learning (ML) policy for average performance improvement. In stark contrast with the existing learning-augmented online algorithms that focus on centralized settings, LADO achieves strong robustness guarantees in a decentralized setting. We also prove the average cost bound for LADO, revealing the tradeoff between average performance and worst-case robustness and demonstrating the advantage of training the ML policy by explicitly considering the robustness requirement.
更多查看译文
关键词
networks,optimization,decentralized,learning-augmented
AI 理解论文
溯源树
样例
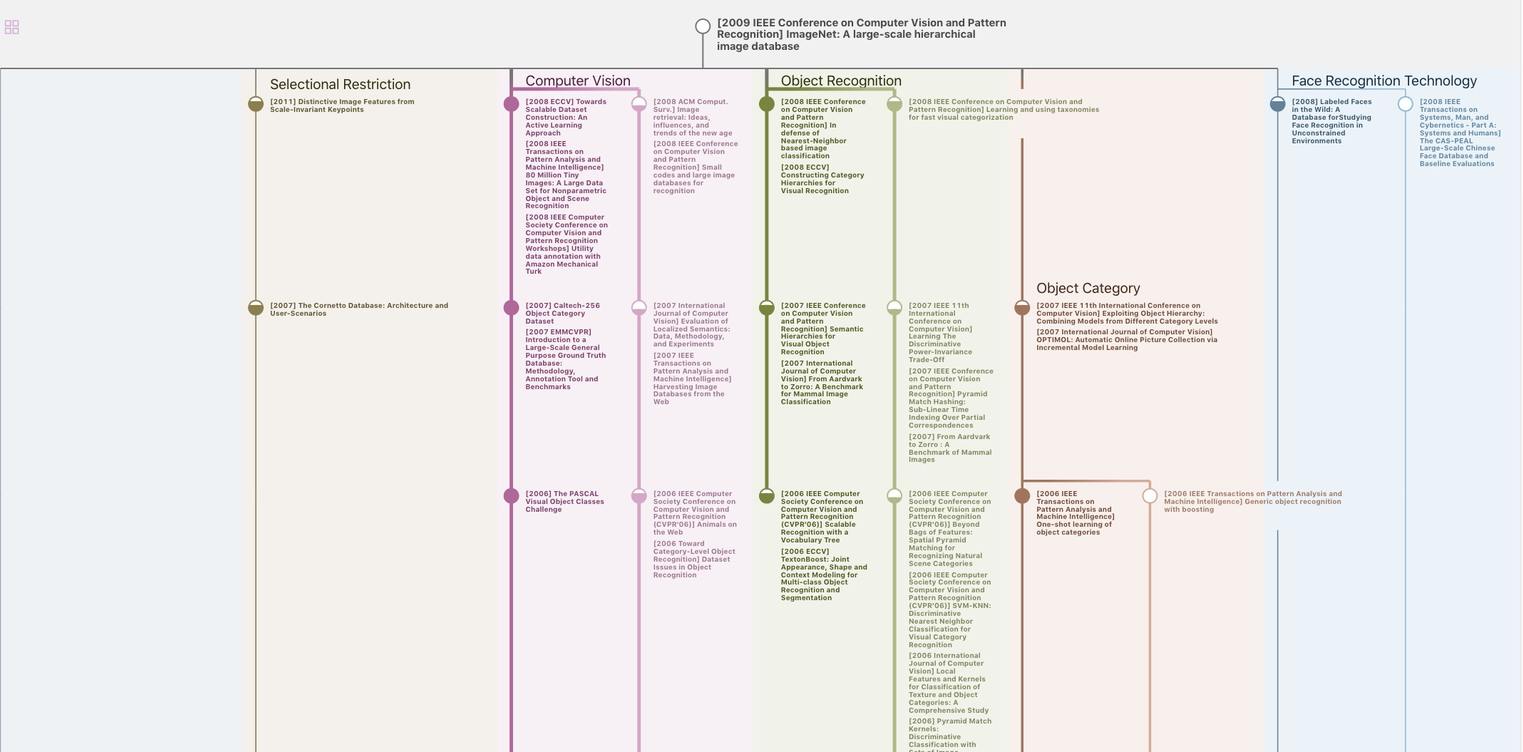
生成溯源树,研究论文发展脉络
Chat Paper
正在生成论文摘要