Tuber Root Growth In Silico Phenotyping Using Adaptive Neuro-Fuzzy Capacitive Resistivity Imaging System
2023 8th International Conference on Business and Industrial Research (ICBIR)(2023)
摘要
The study of plant root growth monitoring is critical for ensuring the sustainability of food production, particularly for tuber and other agricultural crops. Although capacitive resistivity imaging has been researched for non-destructive root tomography, image characterization accuracy remains relatively low. Thus, this study developed an intelligent root radius prediction method by utilizing an adaptive neuro-fuzzy inference system (ANFIS). The capacitive resistivity imaging (CRI) system and root specimen were modeled and simulated using CADFEKO. There were 100 data pairs employed that were divided for training, testing, and checking. From four input variables such as the voltage differences from electrode pairs C1, C2, D1, and D2, and a single output which is the root radius, 81 rules were constructed. Using grid partition method, different membership functions were applied to identify the one with the best performance in predicting the root radii based on the model evaluation metrics. The results shows that the triangular membership function (trimf) has the best performance with 0.9998, 8.85e-
9
, and 0.9999 values for the R2, MSE, and R, respectively. Furthermore, the comparison of the actual and predicted output shows a huge accuracy in result which proves that the developed root radius prediction approach is suitable for CRI system root size prediction.
更多查看译文
关键词
capacitive resistivity imaging,digital agriculture,neuro-fuzzy,nondestructive root imaging,root phenotyping
AI 理解论文
溯源树
样例
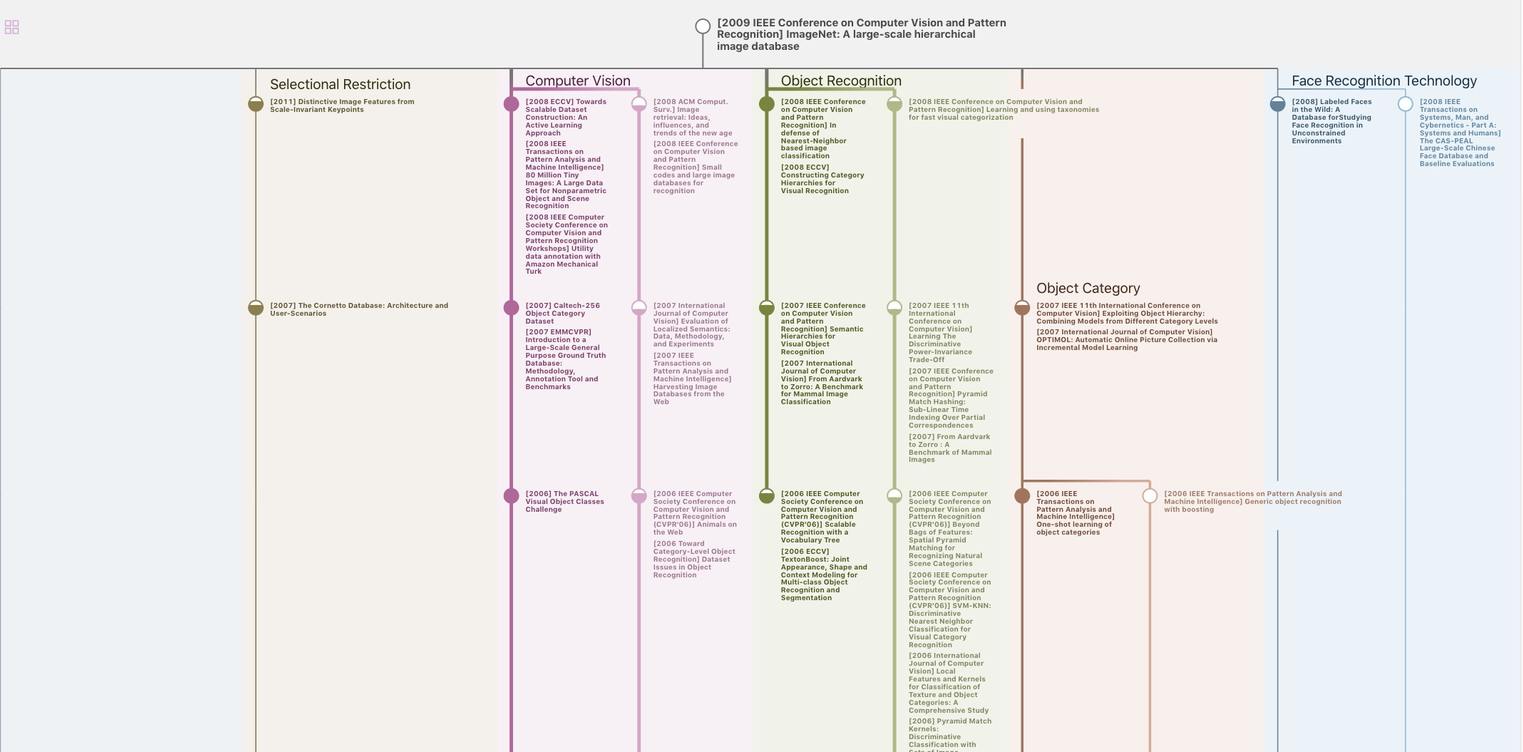
生成溯源树,研究论文发展脉络
Chat Paper
正在生成论文摘要