C2F2NeUS: Cascade Cost Frustum Fusion for High Fidelity and Generalizable Neural Surface Reconstruction
CoRR(2023)
摘要
There is an emerging effort to combine the two popular technical paths, i.e., the multi-view stereo (MVS) and neural implicit surface (NIS), in scene reconstruction from sparse views. In this paper, we introduce a novel integration scheme that combines the multi-view stereo with neural signed distance function representations, which potentially overcomes the limitations of both methods. MVS uses per-view depth estimation and cross-view fusion to generate accurate surface, while NIS relies on a common coordinate volume. Based on this, we propose to construct per-view cost frustum for finer geometry estimation, and then fuse cross-view frustums and estimate the implicit signed distance functions to tackle noise and hole issues. We further apply a cascade frustum fusion strategy to effectively captures global-local information and structural consistency. Finally, we apply cascade sampling and a pseudo-geometric loss to foster stronger integration between the two architectures. Extensive experiments demonstrate that our method reconstructs robust surfaces and outperforms existing state-of-the-art methods.
更多查看译文
关键词
generalizable neural surface reconstruction,cascade cost frustum fusion
AI 理解论文
溯源树
样例
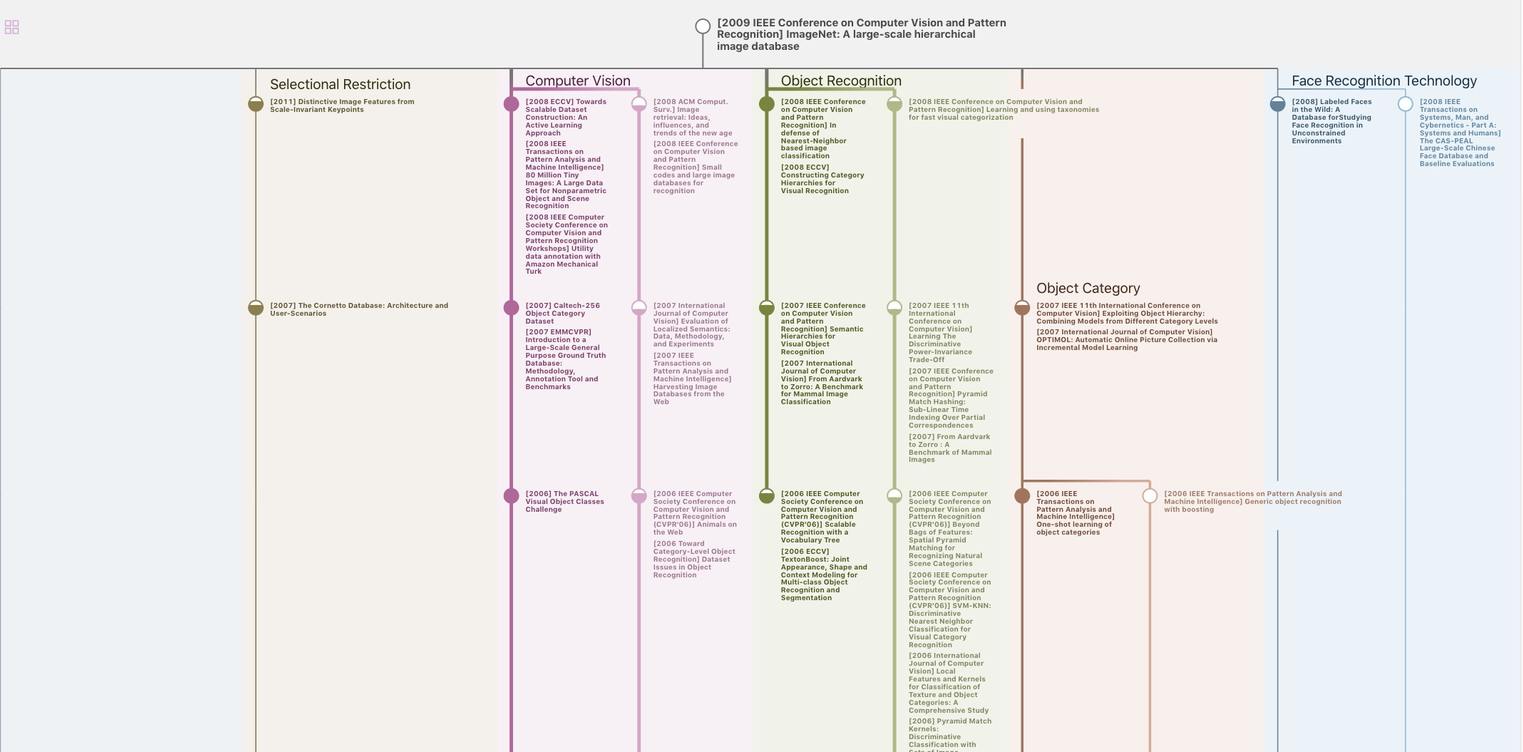
生成溯源树,研究论文发展脉络
Chat Paper
正在生成论文摘要