ClinicalGPT: Large Language Models Finetuned with Diverse Medical Data and Comprehensive Evaluation
CoRR(2023)
摘要
Large language models have exhibited exceptional performance on various Natural Language Processing (NLP) tasks, leveraging techniques such as the pre-training, and instruction fine-tuning. Despite these advances, their effectiveness in medical applications is limited, due to challenges such as factual inaccuracies, reasoning abilities, and lack grounding in real-world experience. In this study, we present ClinicalGPT, a language model explicitly designed and optimized for clinical scenarios. By incorporating extensive and diverse real-world data, such as medical records, domain-specific knowledge, and multi-round dialogue consultations in the training process, ClinicalGPT is better prepared to handle multiple clinical task. Furthermore, we introduce a comprehensive evaluation framework that includes medical knowledge question-answering, medical exams, patient consultations, and diagnostic analysis of medical records. Our results demonstrate that ClinicalGPT significantly outperforms other models in these tasks, highlighting the effectiveness of our approach in adapting large language models to the critical domain of healthcare.
更多查看译文
关键词
large language models,language models,diverse medical data
AI 理解论文
溯源树
样例
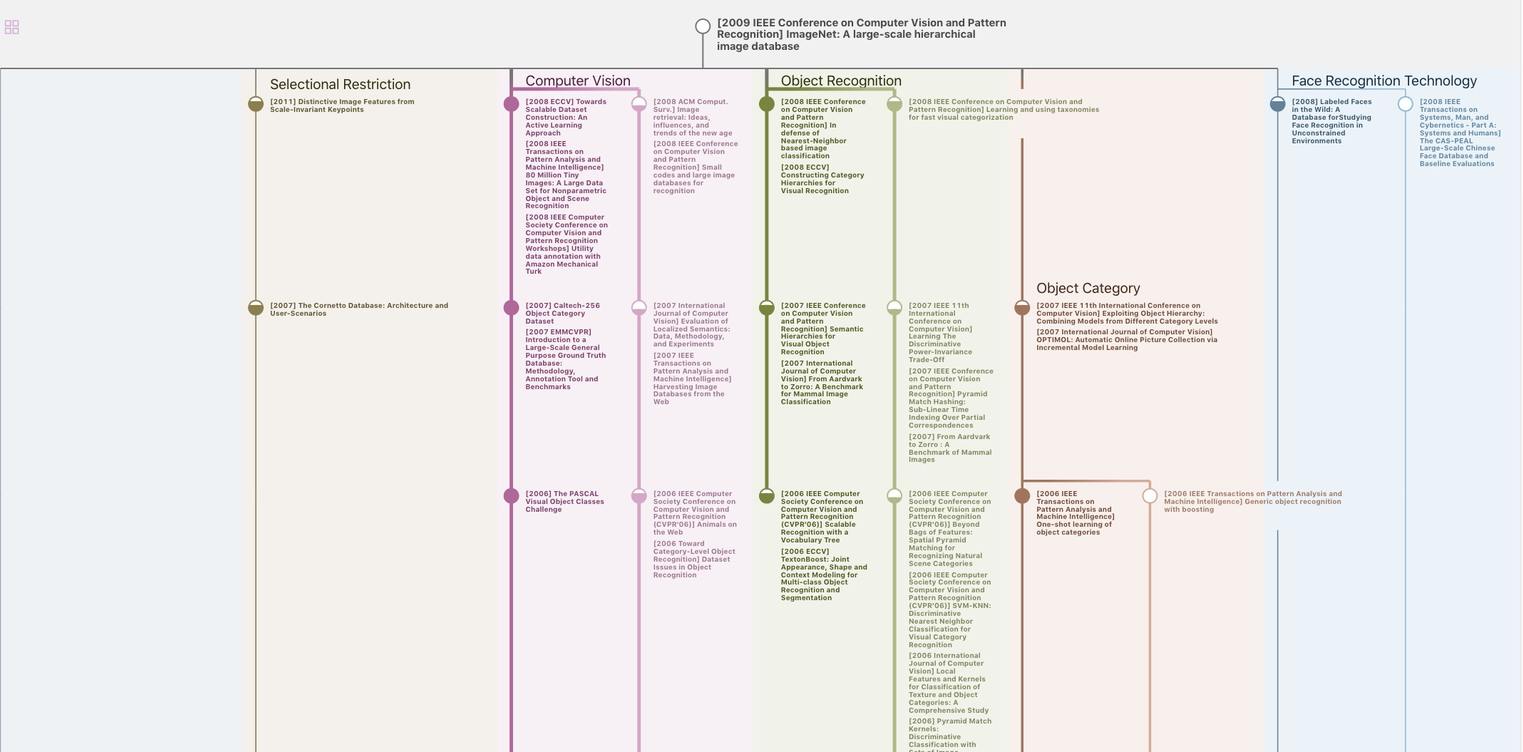
生成溯源树,研究论文发展脉络
Chat Paper
正在生成论文摘要