GRM: Generative Relevance Modeling Using Relevance-Aware Sample Estimation for Document Retrieval
CoRR(2023)
摘要
Recent studies show that Generative Relevance Feedback (GRF), using text generated by Large Language Models (LLMs), can enhance the effectiveness of query expansion. However, LLMs can generate irrelevant information that harms retrieval effectiveness. To address this, we propose Generative Relevance Modeling (GRM) that uses Relevance-Aware Sample Estimation (RASE) for more accurate weighting of expansion terms. Specifically, we identify similar real documents for each generated document and use a neural re-ranker to estimate their relevance. Experiments on three standard document ranking benchmarks show that GRM improves MAP by 6-9% and R@1k by 2-4%, surpassing previous methods.
更多查看译文
关键词
generative relevance modeling,retrieval,relevance-aware
AI 理解论文
溯源树
样例
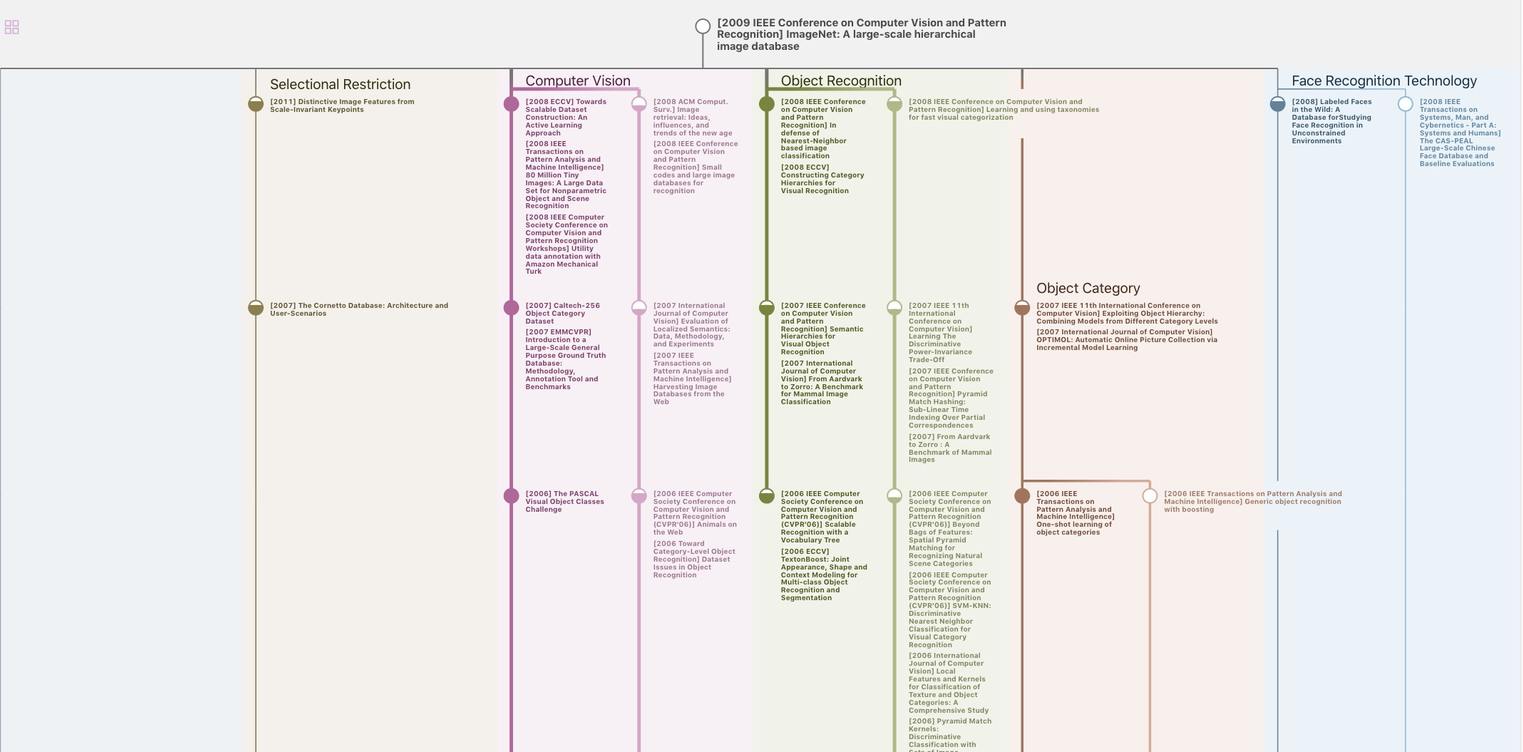
生成溯源树,研究论文发展脉络
Chat Paper
正在生成论文摘要