Uncertainty Quantification via Spatial-Temporal Tweedie Model for Zero-inflated and Long-tail Travel Demand Prediction
PROCEEDINGS OF THE 32ND ACM INTERNATIONAL CONFERENCE ON INFORMATION AND KNOWLEDGE MANAGEMENT, CIKM 2023(2023)
摘要
Understanding Origin-Destination (O-D) travel demand is crucial for transportation management. However, traditional spatial-temporal deep learning models grapple with addressing the sparse and long-tail characteristics in high-resolution O-D matrices and quantifying prediction uncertainty. This dilemma arises from the numerous zeros and over-dispersed demand patterns within these matrices, which challenge the Gaussian assumption inherent to deterministic deep learning models. To address these challenges, we propose a novel approach: the Spatial-Temporal Tweedie Graph Neural Network (STTD). The STTD introduces the Tweedie distribution as a compelling alternative to the traditional 'zero-inflated' model and leverages spatial and temporal embeddings to parameterize travel demand distributions. Our evaluations using real-world datasets highlight STTD's superiority in providing accurate predictions and precise confidence intervals, particularly in high-resolution scenarios. GitHub code is available online(1).
更多查看译文
关键词
Spatial-temporal Sparse Data,Uncertainty Quantification,Graph Neural Networks,Travel Demand Prediction
AI 理解论文
溯源树
样例
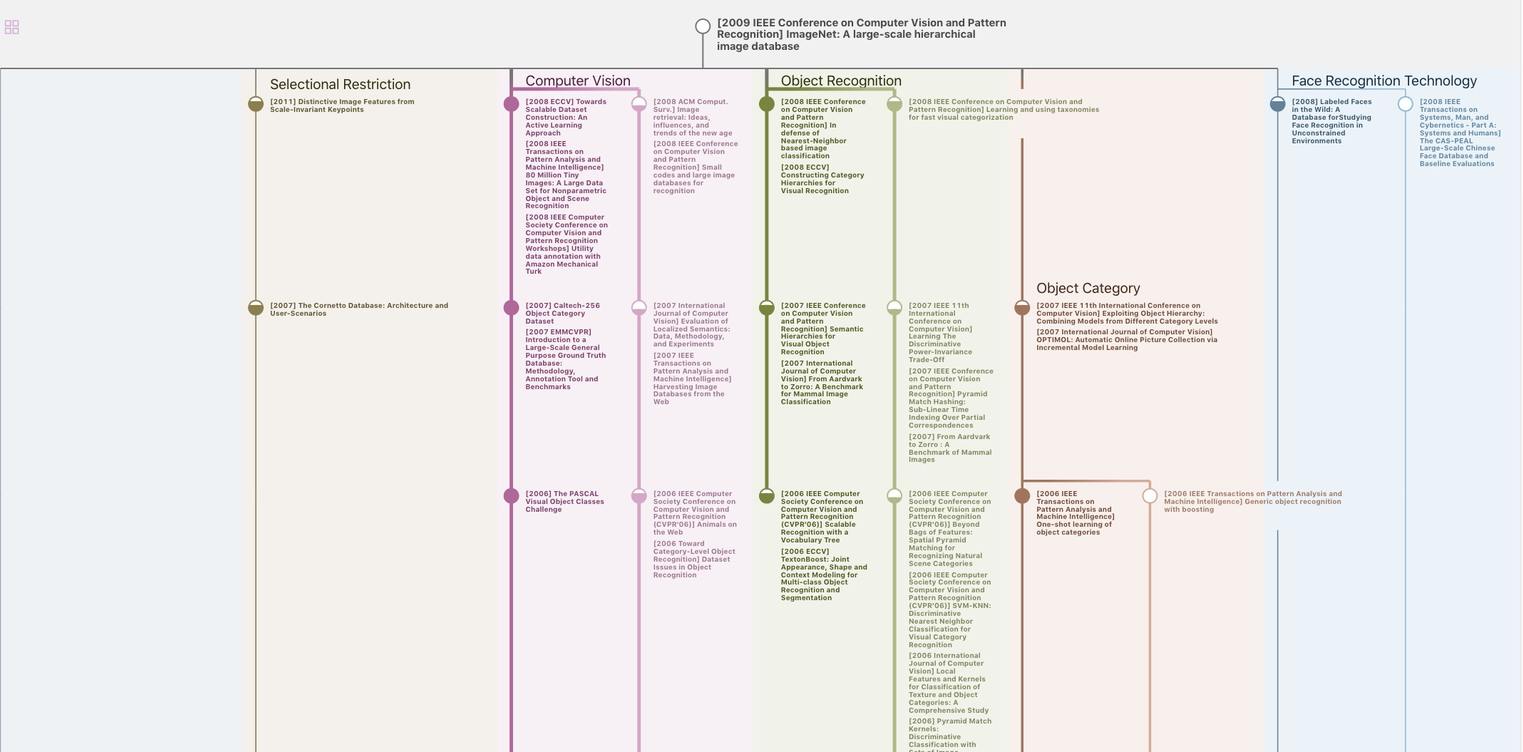
生成溯源树,研究论文发展脉络
Chat Paper
正在生成论文摘要