Lightweight Attribute Localizing Models for Pedestrian Attribute Recognition
CoRR(2023)
摘要
Pedestrian Attribute Recognition (PAR) deals with the problem of identifying features in a pedestrian image. It has found interesting applications in person retrieval, suspect re-identification and soft biometrics. In the past few years, several Deep Neural Networks (DNNs) have been designed to solve the task; however, the developed DNNs predominantly suffer from over-parameterization and high computational complexity. These problems hinder them from being exploited in resource-constrained embedded devices with limited memory and computational capacity. By reducing a network's layers using effective compression techniques, such as tensor decomposition, neural network compression is an effective method to tackle these problems. We propose novel Lightweight Attribute Localizing Models (LWALM) for Pedestrian Attribute Recognition (PAR). LWALM is a compressed neural network obtained after effective layer-wise compression of the Attribute Localization Model (ALM) using the Canonical Polyadic Decomposition with Error Preserving Correction (CPD-EPC) algorithm.
更多查看译文
关键词
lightweight attribute localizing models,pedestrian attribute,recognition
AI 理解论文
溯源树
样例
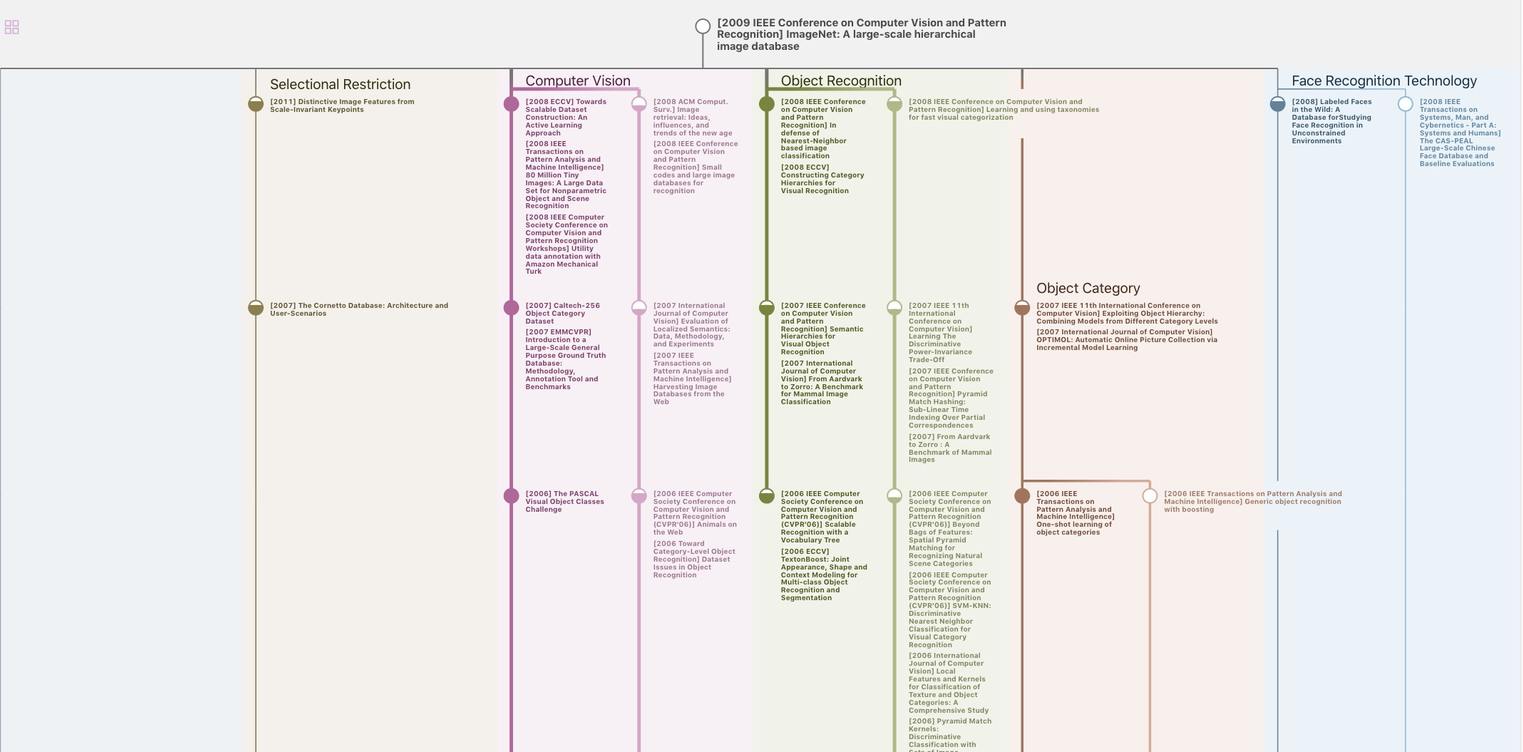
生成溯源树,研究论文发展脉络
Chat Paper
正在生成论文摘要