Training generative models from privatized data
CoRR(2023)
摘要
Local differential privacy (LDP) is a powerful method for privacy-preserving data collection. In this paper, we develop a framework for training Generative Adversarial Networks (GAN) on differentially privatized data. We show that entropic regularization of the Wasserstein distance -- a popular regularization method in the literature that has been often leveraged for its computational benefits -- can be used to denoise the data distribution when data is privatized by common additive noise mechanisms, such as Laplace and Gaussian. This combination uniquely enables the mitigation of both the regularization bias and the effects of privatization noise, thereby enhancing the overall efficacy of the model. We analyse the proposed method, provide sample complexity results and experimental evidence to support its efficacy.
更多查看译文
关键词
generative models,privatized data,training
AI 理解论文
溯源树
样例
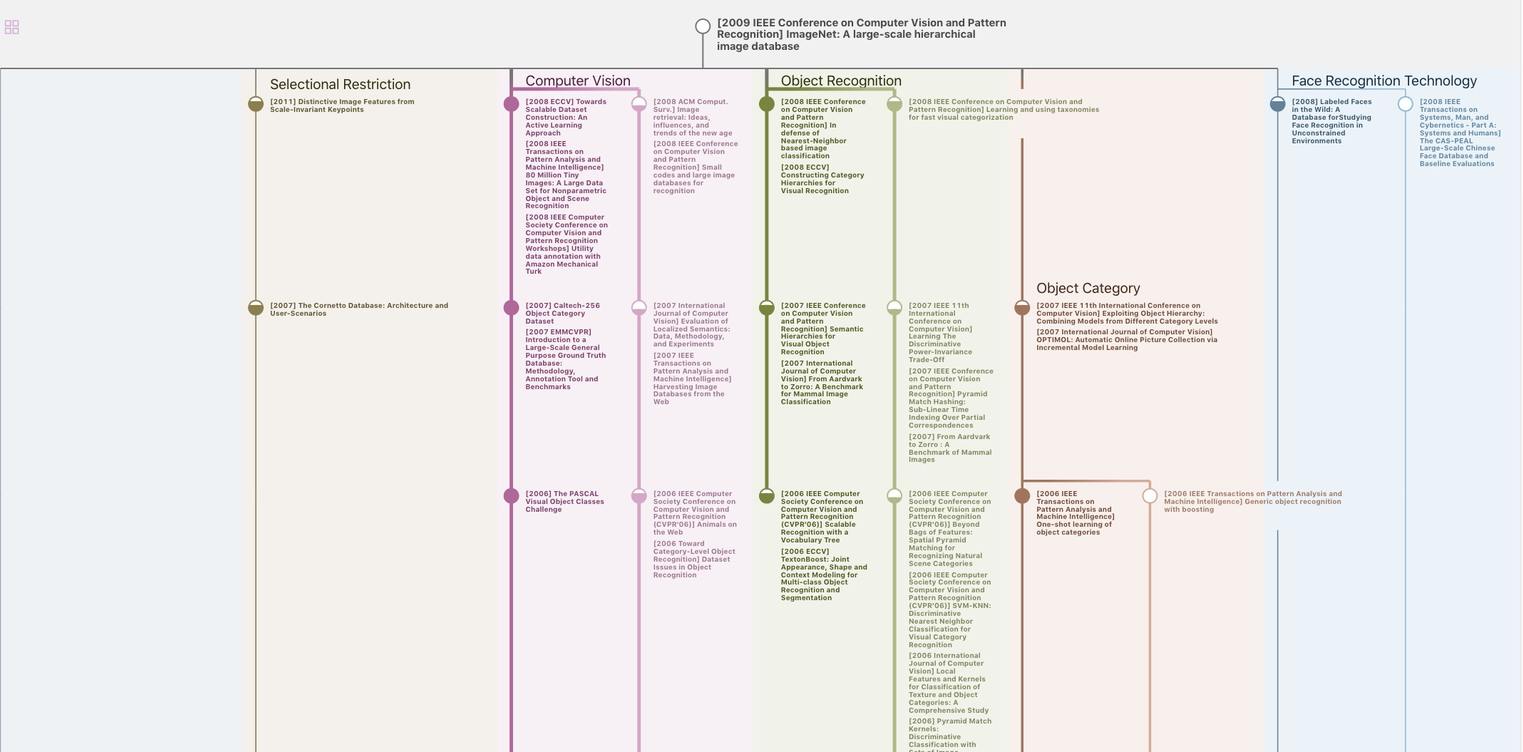
生成溯源树,研究论文发展脉络
Chat Paper
正在生成论文摘要