Predicting Two-Photon Absorption Cross Sections with Experimental Accuracy Using Only Four Molecular Features Revealed by Interpretable Machine Learning
crossref(2022)
摘要
Two-photon absorption has wide applications in bioimaging, photodynamic therapy, and three-dimensional printing. De-signing molecules with a large two-photon absorption cross section (TPACS) is thus highly desirable for advancing these technologies. Here we used machine learning to analyze a TPACS dataset of ca. one thousand molecules collected from literature reports. We found that the length of the conjugated structure is the most important feature to determine the TPACS in a power law of ~1.8 order. The effect of donor and acceptor substitutions and structural coplanarity on the TPACS can be adequately addressed by another three features obtained by empirical rules. Combining these four molecular features with the experimental wavelength and solvent used in the measurement, we derived an interpretable model to predict molecular TPACS with an accuracy comparable to that of experimental measurements and common theoretical calculations. Our approach not only provides insight into the factors that are critical to TPACS but, as we demonstrate, also allows high-throughput screening of new TPA molecules.
更多查看译文
AI 理解论文
溯源树
样例
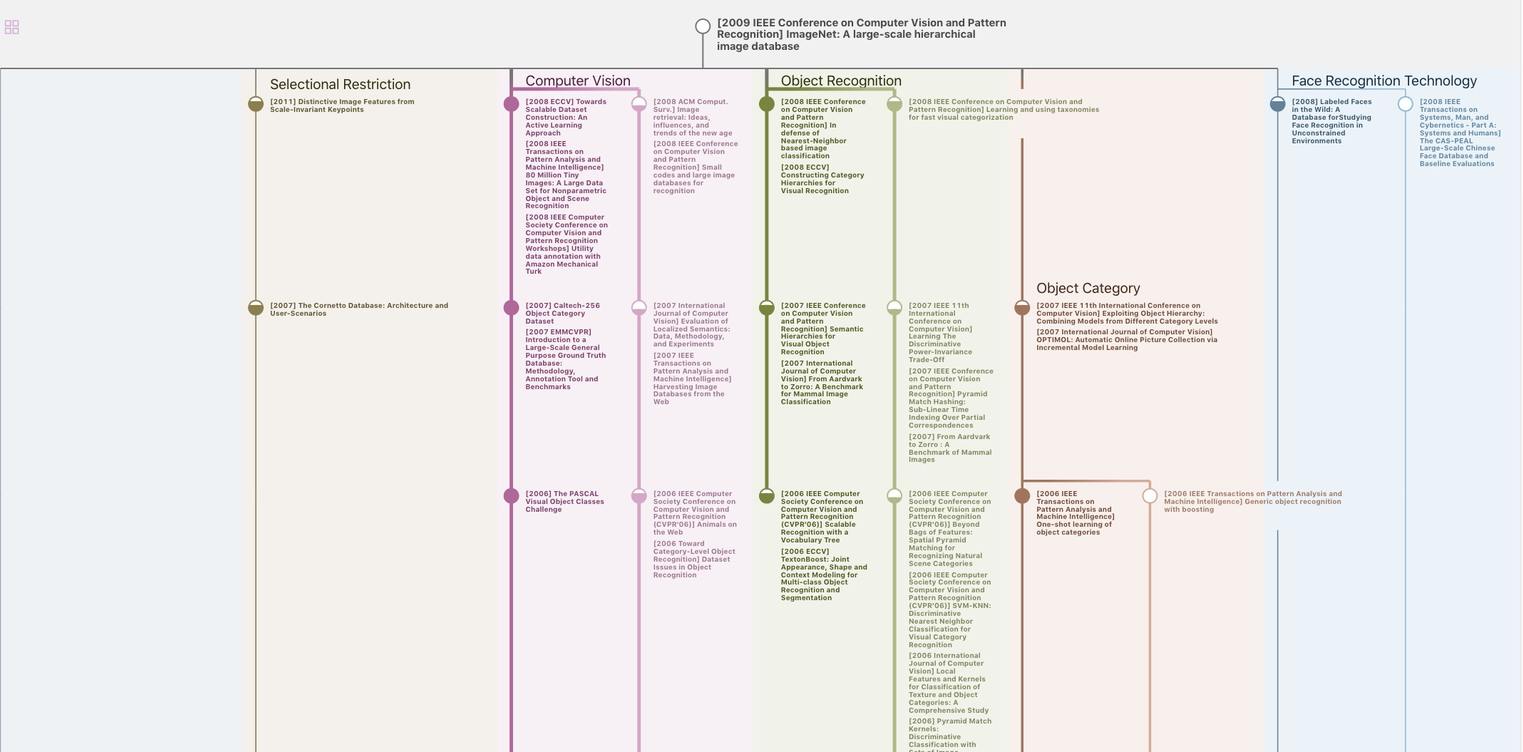
生成溯源树,研究论文发展脉络
Chat Paper
正在生成论文摘要