Development and Validation of a Novel Scoring System to Quantify the Severity of Incident Reports Using Machine Learning: An exploratory research study
Research Square (Research Square)(2022)
摘要
Abstract Incident reporting systems have been widely adopted to collect information about patient safety incidents. The value of incident reports often lies in the free-text section, but this is often inadequately processed. We developed a novel scoring system that uses machine learning to assess the severity of an incident using the semantic characteristics of text in incident reports. We retrospectively analyzed free-text data extracted from incident reports from between January 2012 and September 2021 at Nagoya University Hospital, Aichi, Japan. The sample was randomly allocated into a training dataset and validation dataset. Morphological analysis was used to segment terms using the training dataset. We calculated a severity term score, a severity report score and severity group score, by the report volume size. We then compared these with conventional severity classifications by patient safety experts and reporters. We allocated 96,082 incident reports into two groups. From the 48,041 reports in the training dataset, 1,802 severity term scores were calculated. There was a significant difference in severity report score between reports categorized as severe and not severe by experts (95% confidence interval [CI] − 0.83 to − 0.80, p < 0.001). Severity group scores were positively associated with severity ratings from experts and reporters, with correlation coefficients of 0.73 (95% CI 0.63–0.80, p < 0.001) and 0.79 (95% CI 0.71–0.85, p < 0.001) for all departments. Our severity scoring system using machine learning could contribute to improved organizational patient safety.
更多查看译文
关键词
incident reports,severity,novel scoring system,machine learning
AI 理解论文
溯源树
样例
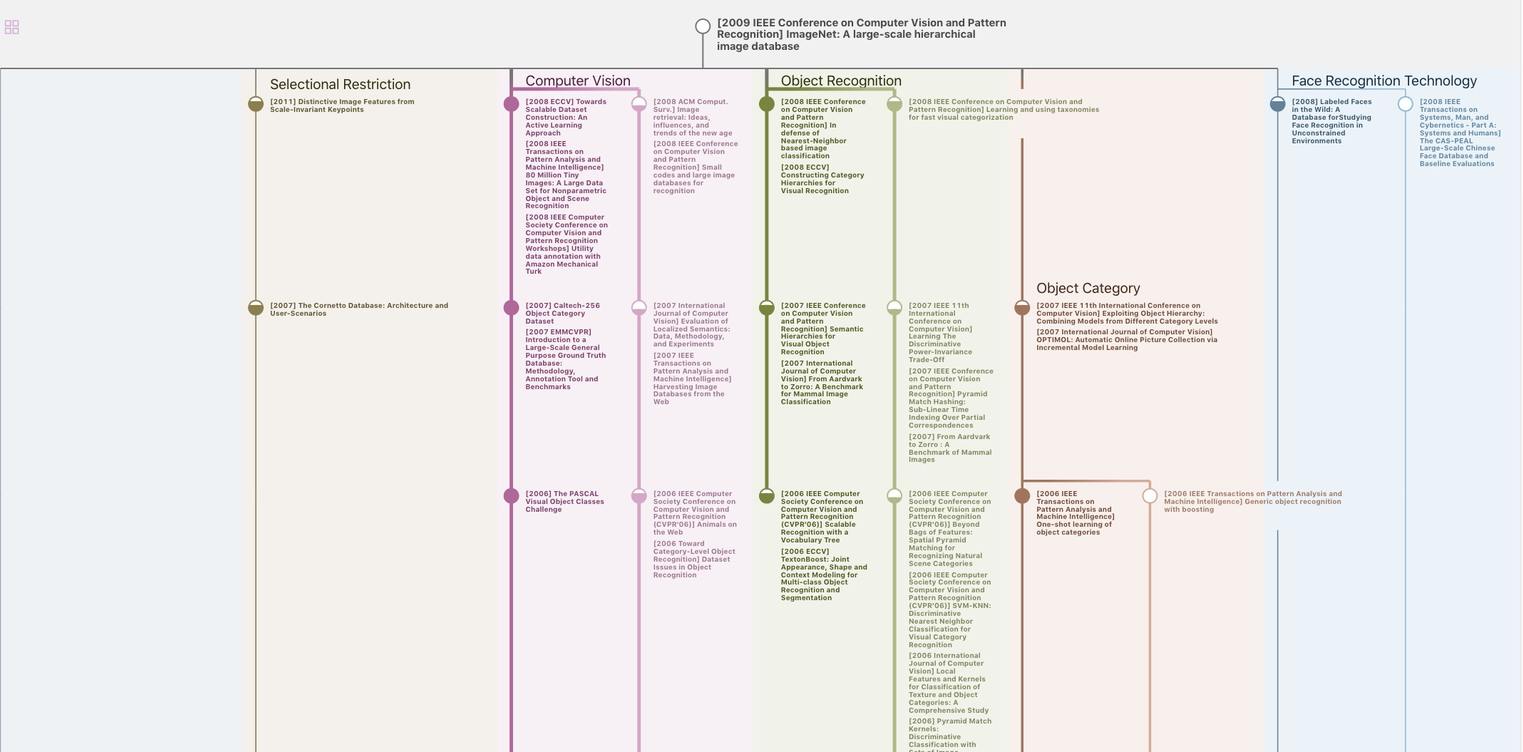
生成溯源树,研究论文发展脉络
Chat Paper
正在生成论文摘要