229. Using machine learning (ML) models to predict risk of venous thromboembolism (VTE) following spine surgery
The Spine Journal(2022)
摘要
BACKGROUND CONTEXT Venous thromboembolism (VTE), which includes deep vein thrombosis (DVT) and pulmonary embolisms (PE) is a potentially high-risk complication for patients undergoing spine surgery. Chemoprophylaxis cannot be administered to all patients due to its potentially serious complications, which can include epidural hematomas, wound related complications, risk of revision surgery, etc. Thus, it is imperative to establish a predictive model that can effectively determine VTE risk in patients who have undergone spine surgery to administer chemoprophylaxis appropriately. PURPOSE The aim of this study was to develop and apply a machine learning (ML) model for predicting VTE in spine surgery patients. STUDY DESIGN/SETTING Retrospective cohort study. OUTCOME MEASURES Outcome measures evaluated for each model include model accuracy, positive predictive value (PPV) and the risk factors used to determine VTE risk and incidence. METHODS A cohort of 63 patients was identified who had undergone spine surgery at a single center from 2015-2021. Thirty-one patients had a confirmed VTE while 32 randomly chosen patients had no VTE. A total of 113 attributes were defined and collected through chart review. Attribute categories included (eg, sex, BMI, insurance, etc), medications, labs (eg, CBC, BMP, coagulation panels), past medical history, operative history (eg, anesthesia time, estimated blood loss, complications) and postoperative history (eg, VTE diagnosis). The Waikato Environment for Knowledge Analysis (WEKA) software was used in creating and evaluating the ML models. Six classifier models were tested with 10-fold cross validation: ZeroR, OneR, Logistic, SimpleLogistic, J48 (C4.5) and RandomForest with VTE as the predicting output. Model accuracy was statistically analyzed using t-tests in WEKA. RESULTS Comparing the predictive ML models to the control model (ZeroR), all predictive models were significantly better than the control model at predicting VTE risk, based on the 113 attributes (p < 0.001). The RandomForest model had the highest accuracy of 88.89% with a PPV of 93.75%. The SimpleLogistic algorithm had an accuracy of 84.13%. This model defined risk attributes to include calcium and phosphate lab values, history of cardiac comorbidity, history of previous VTE, anesthesia time, SSRI use, antibiotic use and antihistamine use. The J48 model had an accuracy of 80.95% and it defined hemoglobin lab values, anesthesia time, beta blocker use, DA agonist use, history of cancer and Medicare use as potential VTE risk factors. CONCLUSIONS We created machine learning models to predict the occurrence of VTE with up to 89% accuracy. Several lesser-known risk factors were identified that increased the accuracy of our models. These models can be used to guide chemoprophylaxis in select patients at high risk for development of VTE postoperatively while avoiding the possible complications associated with anticoagulation in the low-risk patients. FDA DEVICE/DRUG STATUS This abstract does not discuss or include any applicable devices or drugs. Venous thromboembolism (VTE), which includes deep vein thrombosis (DVT) and pulmonary embolisms (PE) is a potentially high-risk complication for patients undergoing spine surgery. Chemoprophylaxis cannot be administered to all patients due to its potentially serious complications, which can include epidural hematomas, wound related complications, risk of revision surgery, etc. Thus, it is imperative to establish a predictive model that can effectively determine VTE risk in patients who have undergone spine surgery to administer chemoprophylaxis appropriately. The aim of this study was to develop and apply a machine learning (ML) model for predicting VTE in spine surgery patients. Retrospective cohort study. Outcome measures evaluated for each model include model accuracy, positive predictive value (PPV) and the risk factors used to determine VTE risk and incidence. A cohort of 63 patients was identified who had undergone spine surgery at a single center from 2015-2021. Thirty-one patients had a confirmed VTE while 32 randomly chosen patients had no VTE. A total of 113 attributes were defined and collected through chart review. Attribute categories included (eg, sex, BMI, insurance, etc), medications, labs (eg, CBC, BMP, coagulation panels), past medical history, operative history (eg, anesthesia time, estimated blood loss, complications) and postoperative history (eg, VTE diagnosis). The Waikato Environment for Knowledge Analysis (WEKA) software was used in creating and evaluating the ML models. Six classifier models were tested with 10-fold cross validation: ZeroR, OneR, Logistic, SimpleLogistic, J48 (C4.5) and RandomForest with VTE as the predicting output. Model accuracy was statistically analyzed using t-tests in WEKA. Comparing the predictive ML models to the control model (ZeroR), all predictive models were significantly better than the control model at predicting VTE risk, based on the 113 attributes (p < 0.001). The RandomForest model had the highest accuracy of 88.89% with a PPV of 93.75%. The SimpleLogistic algorithm had an accuracy of 84.13%. This model defined risk attributes to include calcium and phosphate lab values, history of cardiac comorbidity, history of previous VTE, anesthesia time, SSRI use, antibiotic use and antihistamine use. The J48 model had an accuracy of 80.95% and it defined hemoglobin lab values, anesthesia time, beta blocker use, DA agonist use, history of cancer and Medicare use as potential VTE risk factors. We created machine learning models to predict the occurrence of VTE with up to 89% accuracy. Several lesser-known risk factors were identified that increased the accuracy of our models. These models can be used to guide chemoprophylaxis in select patients at high risk for development of VTE postoperatively while avoiding the possible complications associated with anticoagulation in the low-risk patients.
更多查看译文
关键词
venous thromboembolism,spine surgery,machine learning,models,ml
AI 理解论文
溯源树
样例
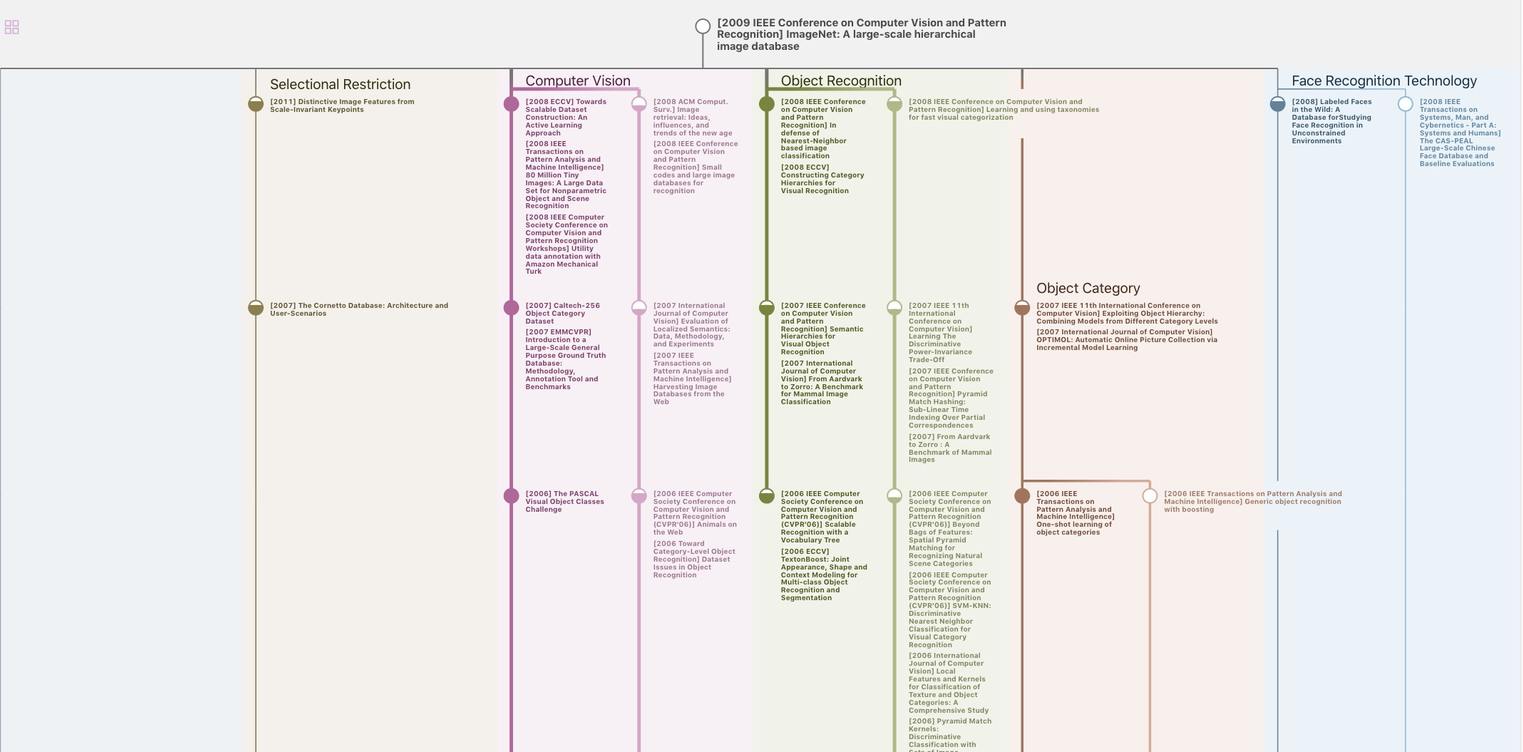
生成溯源树,研究论文发展脉络
Chat Paper
正在生成论文摘要