Forecasting Fine-Grained Urban Flows Via Spatio-Temporal Contrastive Self-Supervision
IEEE Transactions on Knowledge and Data Engineering(2023)
摘要
As a critical task of the urban traffic services, fine-grained urban flow inference (FUFI) benefits in many fields including intelligent transportation management, urban planning, public safety. FUFI is a technique that focuses on inferring fine-grained urban flows depending solely on observed coarse-grained data. However, existing methods always require massive learnable parameters and the complex network structures. To reduce these defects, we formulate a contrastive self-supervision method to predict fine-grained urban flows taking into account all correlated spatial and temporal contrastive patterns. Through several well-designed self-supervised tasks, uncomplicated networks have a strong ability to capture high-level representations from flow data. Then, a fine-tuning network combining with three pre-training encoder networks is proposed. We conduct experiments to evaluate our model and compare with other state-of-the-art methods by using two real-world datasets. All the empirical results not only show the superiority of our model against other comparative models, but also demonstrate its effectiveness in the resource-limited environment.
更多查看译文
关键词
urban,fine-grained,spatio-temporal,self-supervision
AI 理解论文
溯源树
样例
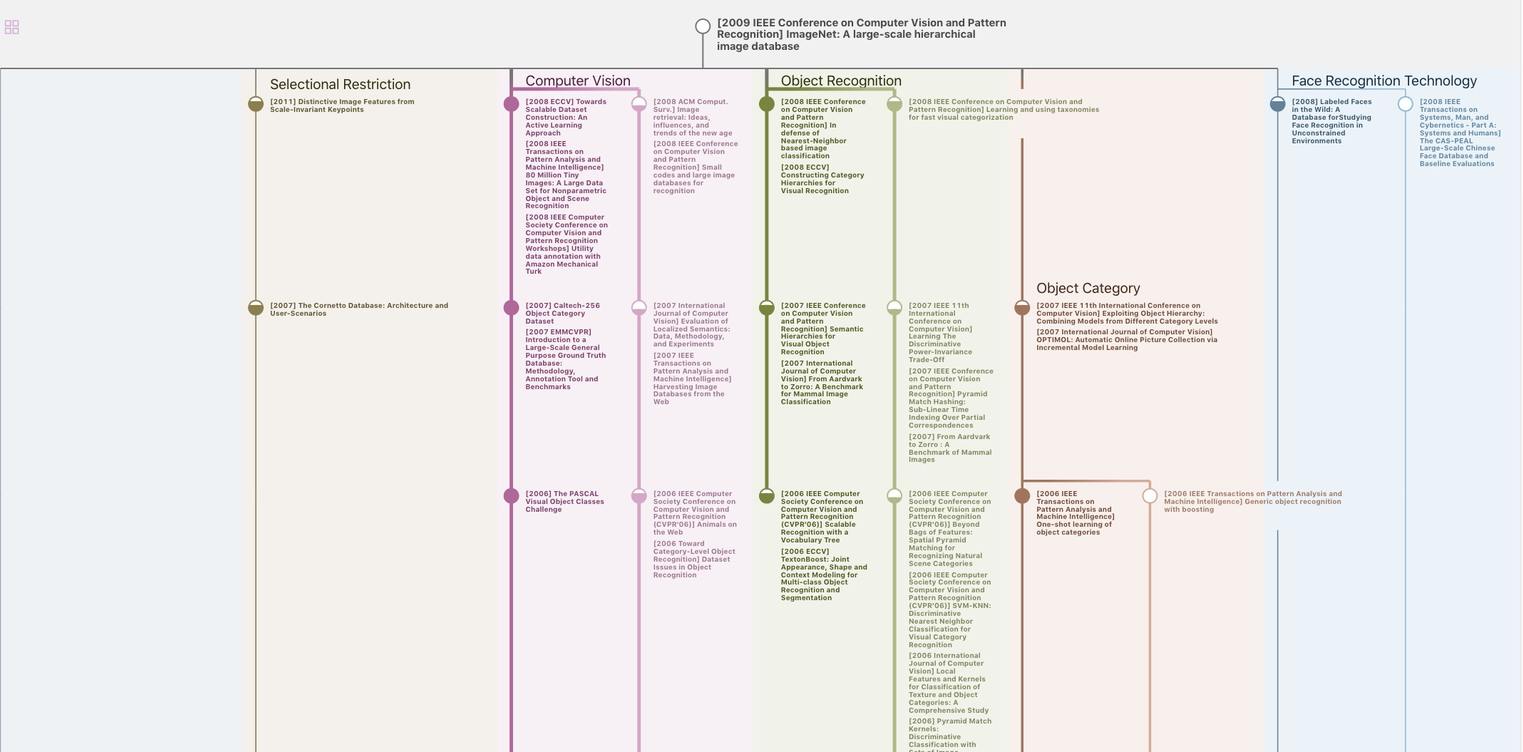
生成溯源树,研究论文发展脉络
Chat Paper
正在生成论文摘要