Fully automatic transfer and measurement system for structural superlubric materials
NATURE COMMUNICATIONS(2023)
摘要
Structural superlubricity, a state of nearly zero friction and no wear between two contact surfaces under relative sliding, holds immense potential for research and application prospects in micro-electro-mechanical systems devices, mechanical engineering, and energy resources. A critical step towards the practical application of structural superlubricity is the mass transfer and high throughput performance evaluation. Limited by the yield rate of material preparation, existing automated systems, such as roll printing or massive stamping, are inadequate for this task. In this paper, a machine learning-assisted system is proposed to realize fully automated selective transfer and tribological performance measurement for structural superlubricity materials. Specifically, the system has a judgment accuracy of over 98% for the selection of micro-scale graphite flakes with structural superlubricity properties and complete the 100 graphite flakes assembly array to form various pre-designed patterns within 100 mins, which is 15 times faster than manual operation. Besides, the system is capable of automatically measuring the tribological performance of over 100 selected flakes on Si3N4, delivering statistical results for new interface which is beyond the reach of traditional methods. With its high accuracy, efficiency, and robustness, this machine learning-assisted system promotes the fundamental research and practical application of structural superlubricity. A critical step to enable practical structural superlubricity (SSL) applications is to enable high throughput to both fabrication and performance evaluation. Here, the authors demonstrate an automated system for efficient and multiple SSL materials transfer and tribological measurement.
更多查看译文
关键词
structural superlubric materials,measurement system,automatic transfer
AI 理解论文
溯源树
样例
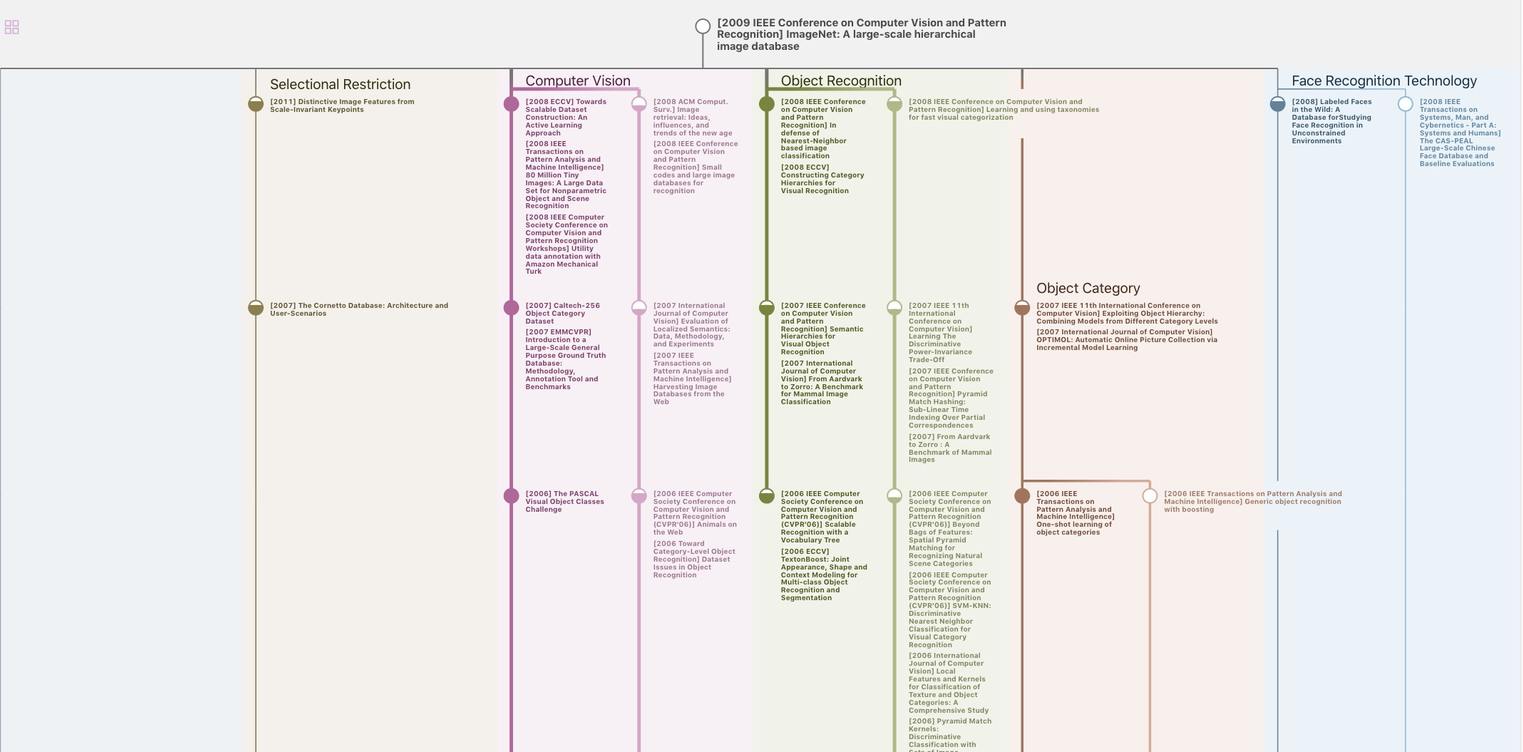
生成溯源树,研究论文发展脉络
Chat Paper
正在生成论文摘要