Deep pairwise learning for user preferences via dual graph attention model in location-based social networks.
Expert Syst. Appl.(2023)
摘要
The rapid growth of location-based social networks (LBSN) has generated massive multi-mode entities such as users, locations and social topics, as well as multi-relationships among them. Learning deep user preferences over point of interests (POIs) and social topics in LBSN has attracted more and more attentions. However, most of the previous studies have failed to incorporate the severely sparse and multi-relational data of heterogeneous LBSN, which plays a vital role to enhance the prediction performance. Toward this challenge, in this paper, we propose a unified pairwise relationship prediction framework based on graph neural networks combined with dual attention mechanism model, dubbed GE2AT, which has fully considered to incorporate three types of user behavioral relations to better capture user preference over different entities. Specifically, we first model LBSN as one user social graph only including social relations, and other two bipartite graphs involved user-location check-in relations and user-social topics following relations respectively. Then, we propose dual graph attention networks to jointly learn deep latent representations of different entities by aggregating features from neighbors with different importance weights. After that, we adopt two multi-layer perceptron (MLP) to output the pairwise preferences of users over POIs and social topics. In addition, to alleviate data sparsity problem, on the basis of MLPs output results, we further leverage social relationships as social-aware influence for similar user preferences so as to enhance the pairwise prediction performance. Extensive experiments on two real-world datasets have demonstrated that our proposed framework GE2AT significantly outperform the state-of-the-art baselines on both prediction tasks for user preferences on POIs and social topics.
更多查看译文
关键词
dual graph attention model,deep pairwise,networks,user preferences,location-based
AI 理解论文
溯源树
样例
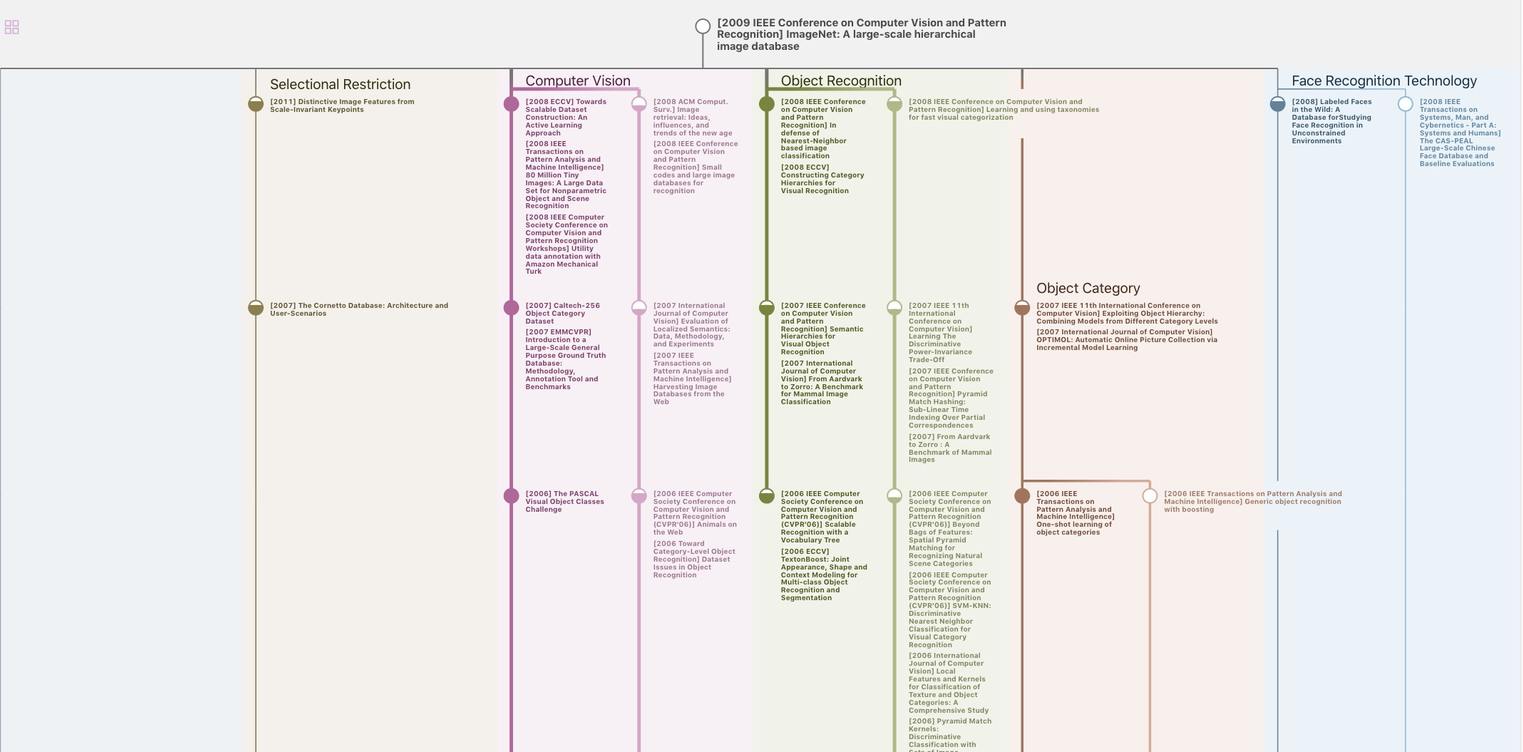
生成溯源树,研究论文发展脉络
Chat Paper
正在生成论文摘要