Subtypes of Early-Onset Restrictive Food Intake Disorder in Children: A Latent Class Analysis
Research Square (Research Square)(2022)
摘要
Abstract Rationale. The two most frequent early-onset restrictive food intake disorders are early-onset anorexia nervosa (EOAN) and avoidant/restrictive food intake disorders (ARFID). Although the core symptoms of EOAN(i.e., fear of gaining weight and disturbed body image) are not present in ARFID, these symptoms are difficult to assess during the initial phase of hospitalisation. Objectives: (i) To identify restrictive food intake disorder subtypes in children using latent class analysis (LCA) based on information available at admission to hospital, and (ii) to determine the agreement between the subtypes identified using LCA and the final diagnosis: EOAN or ARFID. Method: We retrospectively included 97 children under 13 years old with severe eating disorders (DSM-5) at their first hospitalisation in a specialized French paediatric unit. LCA was based on clinical information, growth chart analyses and socio-demographic parameters available at admission. We then compared the probabilities of latent class membership with the diagnosis (EOAN or ARFID) made at the end of the hospitalisation. Results: The most parsimonious LCA model was a 2-class solution. Children diagnosed with EOAN at the end of hospitalisation had a 100% probability of belonging to class 1 while children diagnosed with ARFID had an 8% probability of belonging to class 1 based on parameters available at admission. Discussion: Our results indicate that clinical and socio-demographic characteristics other than the core symptoms of EOAN may be discriminating for a differential diagnosis.
更多查看译文
关键词
restrictive food intake disorder,food intake,latent class analysis,early-onset
AI 理解论文
溯源树
样例
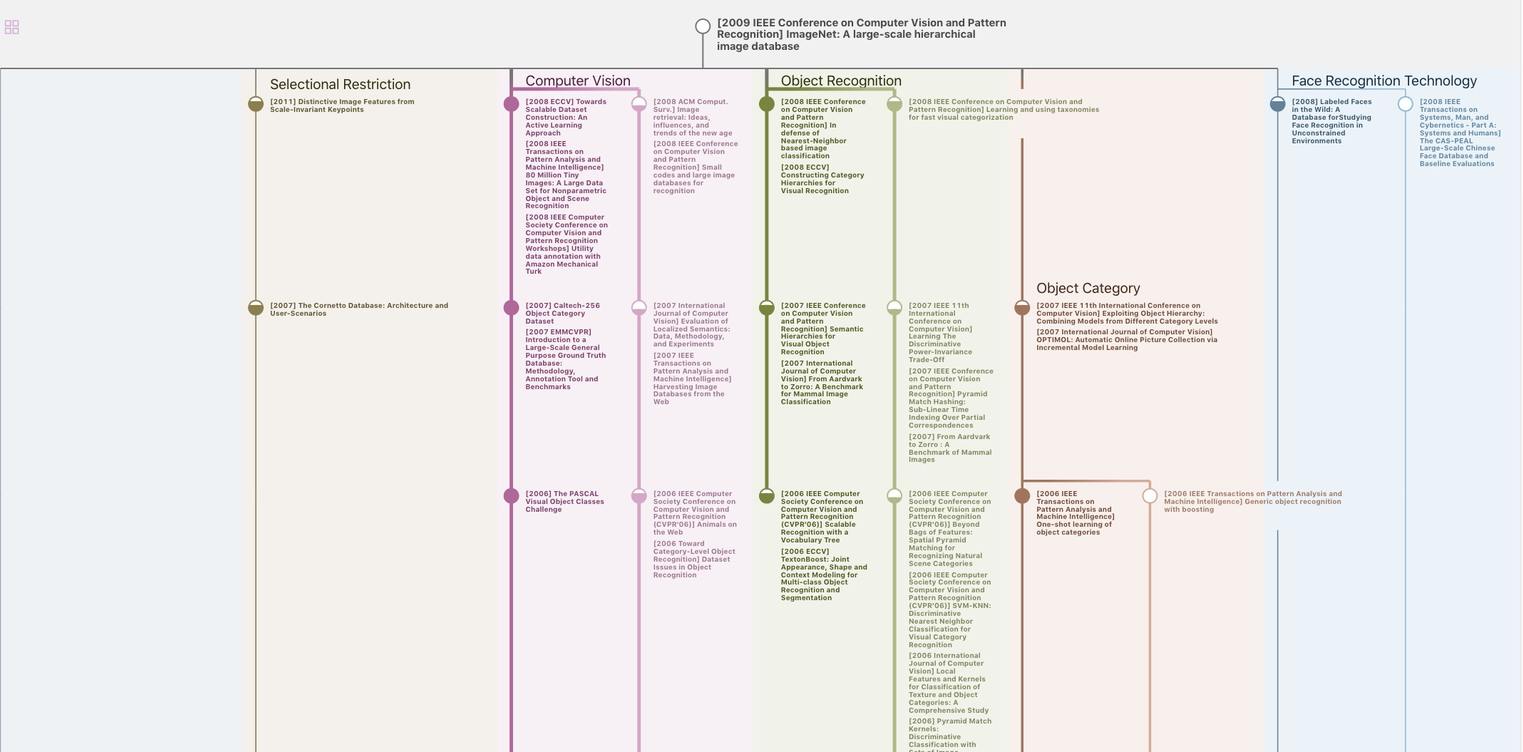
生成溯源树,研究论文发展脉络
Chat Paper
正在生成论文摘要