Data Mining and Machine Learning over HPC Approach Enhancing Antibody Conformations Prediction
Acs Symposium Series(2023)
摘要
The objective of this chapter is to demonstrate that the combined use of data mining and machine learning (ML) in a high-performance computing (HPC) environment offers a significant contribution to the process of computing the effects of antibody stress under the effect of production parameters, determining monoclonal antibodies (mAbs) stress conditions, and predicting the values of parameters that influence the loss of function or structure of antibodies. Indeed, there is a relationship between the structure, activity, and loss of function and the stress undergone by the mAb during the manufacturing process. Structural deformation can lead to loss of function of the mAb and be one of the most probable causes of antibodies bio-resistance. In this chapter, we propose a new molecular dynamics calculation architecture integrating AI and data mining in an HPC environment to determine the conformations most prone to the aggregation of mAbs. From there, we speculate on the use of ML models to predict these conformations as well as the link between aggregation and loss of function. Finally, we determine how the loss of function can promote conditions for antibiotic resistance. The concrete use cases study is focused on Pembrolizumab, an antibody that is well documented in the scientific literature.
更多查看译文
关键词
antibody,machine learning,prediction
AI 理解论文
溯源树
样例
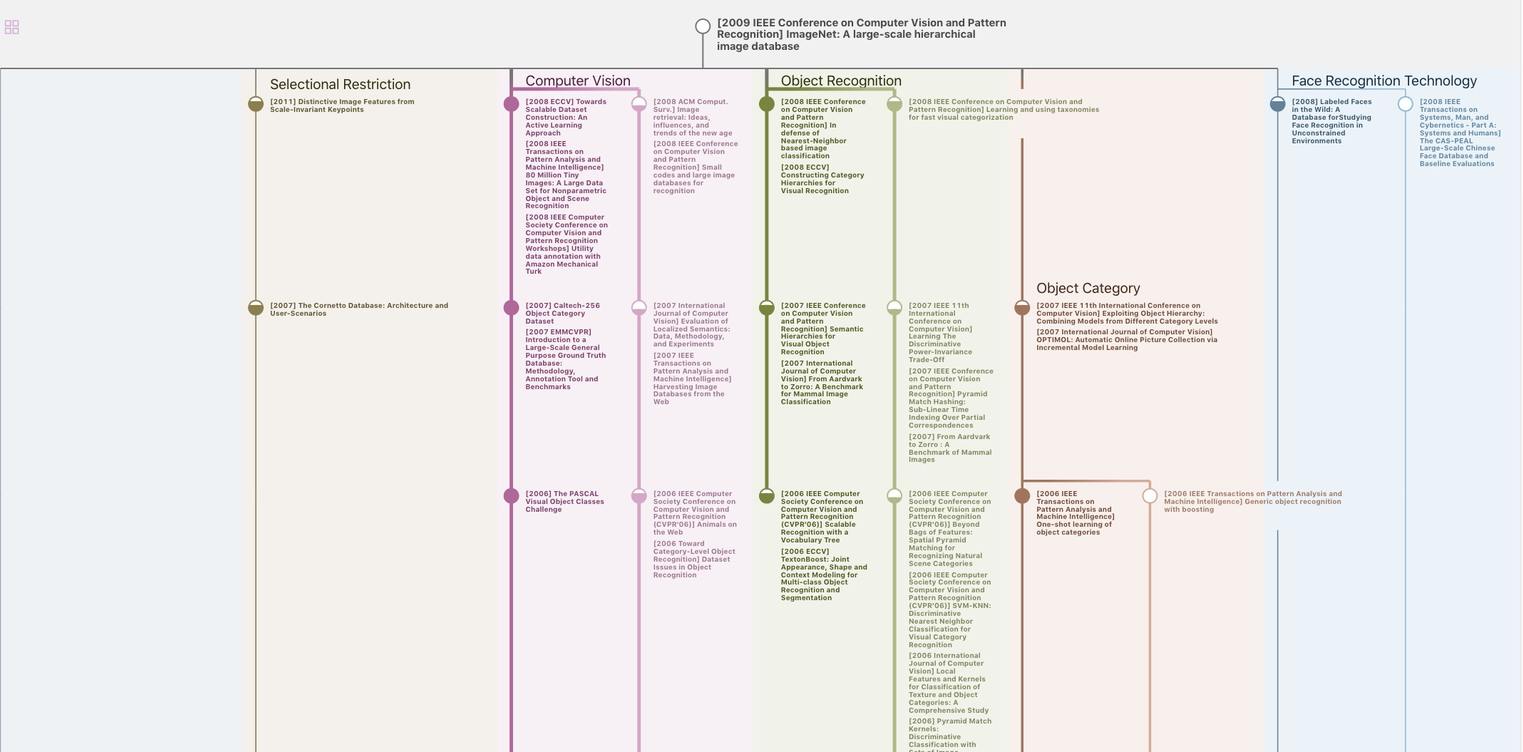
生成溯源树,研究论文发展脉络
Chat Paper
正在生成论文摘要