Artificial neural network encoding of molecular wavefunctions for quantum computing
DIGITAL DISCOVERY(2023)
摘要
Artificial neural networks (ANNs) for material modeling have received significant interest. We recently reported an adaptation of ANNs based on Boltzmann machine (BM) architectures to an ansatz of the multiconfigurational many-electron wavefunction, denoted as a neural-network quantum state (NQS), for quantum chemistry calculations [Yang et al., J. Chem. Theory Comput., 2020, 16, 3513-3529]. Here, this study presents its extended formalism to a quantum algorithm that enables the preparation of the NQS through quantum gates. The descriptors of the ANN model, which are chosen as the occupancies of electronic configurations, are quantum-mechanically represented by qubits. Our algorithm may thus bring potential advantages over classical sampling-based computation employed in previous studies. The NQS can be accurately formed using quantum-native procedures. Still, the training of the model in terms of energy minimization is efficiently performed on a classical computer; thus, our approach is a class of variational quantum eigensolvers. The BM models are related to the Gibbs distribution, and our preparation procedures exploit techniques of quantum phase estimation but with no Hamiltonian evolution. The proposed algorithm is assessed by implementing it on a quantum computer simulator. Illustrative molecular calculations at the complete-active-space configuration interaction level of theory are displayed, confirming consistency with the accuracy of our previous classical approaches. Molecular wavefunction encoded with artificial neural networks (BM2, BM3, and RBM) is prepared by the quantum algorithm.
更多查看译文
关键词
molecular wavefunctions,artificial neural network encoding,quantum,artificial neural network,neural network
AI 理解论文
溯源树
样例
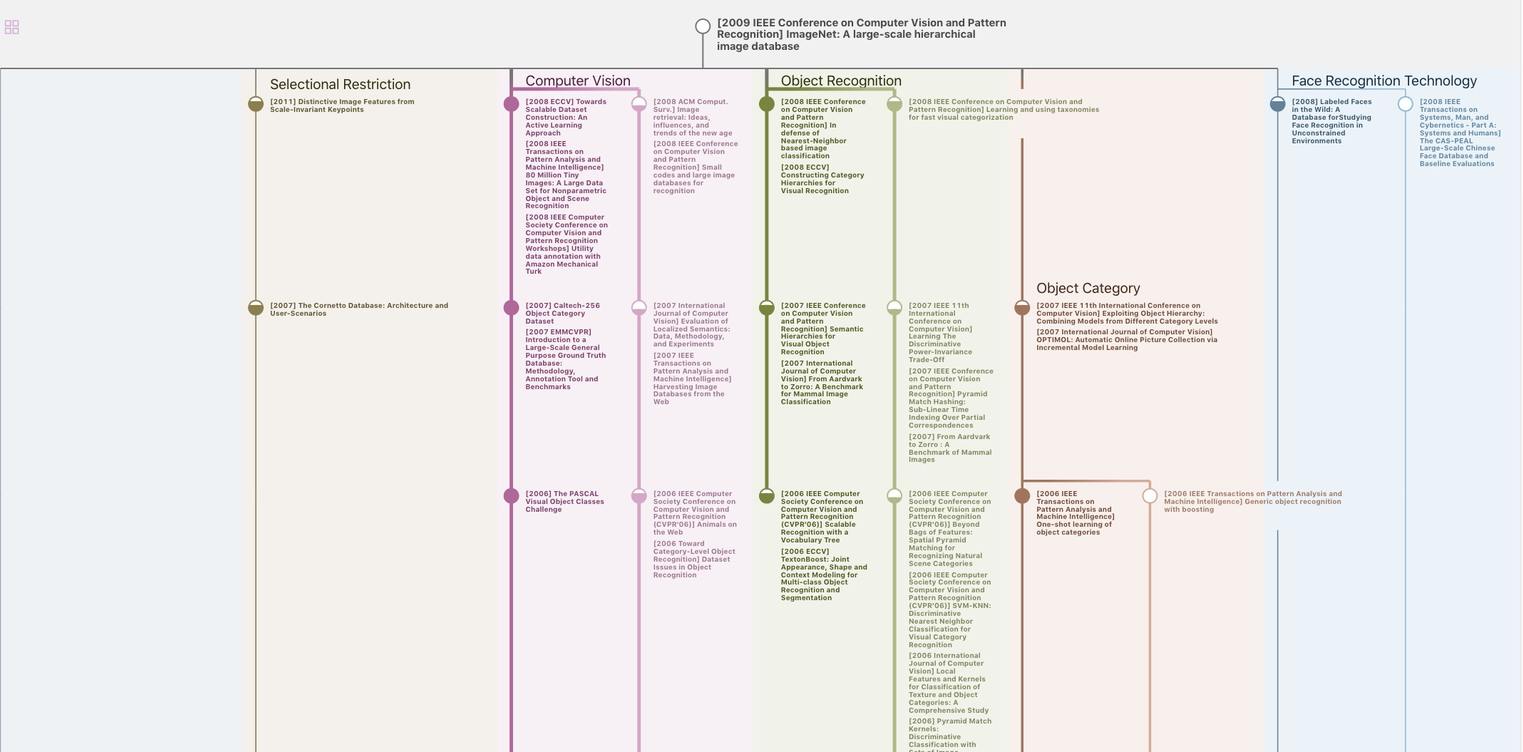
生成溯源树,研究论文发展脉络
Chat Paper
正在生成论文摘要