Consumer-grade UAV solid-state LiDAR accurately quantifies topography in a vegetated fluvial environment
EARTH SURFACE PROCESSES AND LANDFORMS(2023)
摘要
Unoccupied aerial vehicles (UAVs) with passive optical sensors have become popular for reconstructing topography using Structure from Motion (SfM) photogrammetry. Advances in UAV payloads and the advent of solid-state LiDAR have enabled consumer-grade active remote sensing equipment to become more widely available, potentially providing opportunities to overcome some challenges associated with SfM photogrammetry, such as vegetation penetration and shadowing, that can occur when processing UAV-acquired images. We evaluate the application of a DJI Zenmuse L1 solid-state LiDAR sensor on a Matrice 300 RTK UAV to generate digital elevation models (DEMs). To assess flying height (60-80 m) and speed parameters (5-10 ms(-1)) on accuracy, four point clouds were acquired at a test site. These point clouds were used to develop a processing workflow to georeference, filter and classify the point clouds to produce a raster DEM product. A dense control network showed that there was no significant difference in georeferencing from differing flying height or speed. Building on the test results, a 3 km reach of the River Feshie was surveyed, collecting over 755 million UAV LiDAR points. The Multiscale Curvature Classification algorithm was found to be the most suitable classifier of ground topography. GNSS check points showed a mean vertical residual of -0.015 m on unvegetated gravel bars. Multiscale Model to Model Cloud Comparison (M3C2) residuals compared UAV LiDAR and Terrestrial Laser Scanner point clouds for seven sample sites demonstrating a close match with marginally zero residuals. Solid-state LiDAR was effective at penetrating sparse canopy-type vegetation but was less penetrable through dense ground-hugging vegetation (e.g. heather, thick grass). Whilst UAV solid-state LiDAR needs to be supplemented with bathymetric mapping to produce wet-dry DEMs, by itself, it offers advantages to comparable geomatics technologies for kilometre-scale surveys. Ten best practice recommendations will assist users of UAV solid-state LiDAR to produce bare earth DEMs.
更多查看译文
关键词
accuracy,fluvial remote sensing,ground classification,solid-state LiDAR,topography,vegetation
AI 理解论文
溯源树
样例
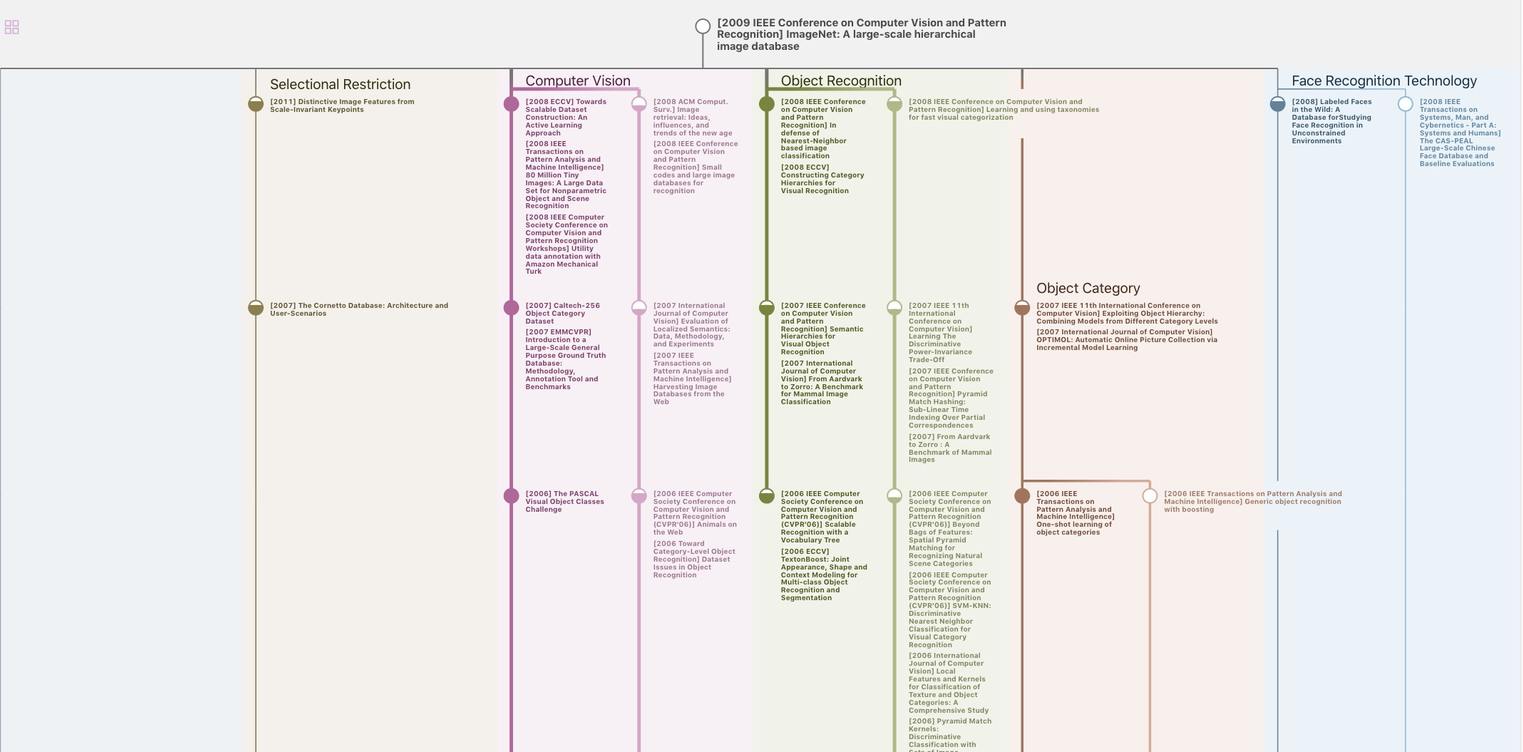
生成溯源树,研究论文发展脉络
Chat Paper
正在生成论文摘要