Machine-Learned Climate Model Corrections From a Global Storm-Resolving Model: Performance Across the Annual Cycle
JOURNAL OF ADVANCES IN MODELING EARTH SYSTEMS(2023)
摘要
One approach to improving the accuracy of a coarse-grid global climate model is to add machine-learned (ML) state-dependent corrections to the prognosed model tendencies, such that the climate model evolves more like a reference fine-grid global storm-resolving model (GSRM). Our past work demonstrating this approach was trained with short (40-day) simulations of GFDL's X-SHiELD GSRM with 3 km global horizontal grid spacing. Here, we extend this approach to span the full annual cycle by training and testing our ML using a new year-long GSRM simulation. Our corrective ML models are trained by learning the state-dependent tendencies of temperature and humidity and surface radiative fluxes needed to nudge a closely related 200 km grid coarse model, FV3GFS, to the GSRM evolution. Coarse-grid simulations adding these learned ML corrections run stably for multiple years. Compared to a no-ML baseline, the time-mean spatial pattern errors with respect to the fine-grid target are reduced by 6%-26% for land surface temperature and 9%-25% for land surface precipitation. The ML-corrected simulations develop other biases in climate and circulation that differ from, but have comparable amplitude to, the no-ML baseline simulation.
更多查看译文
关键词
climate modeling, machine learning, global storm-resolving model, coarse-graining
AI 理解论文
溯源树
样例
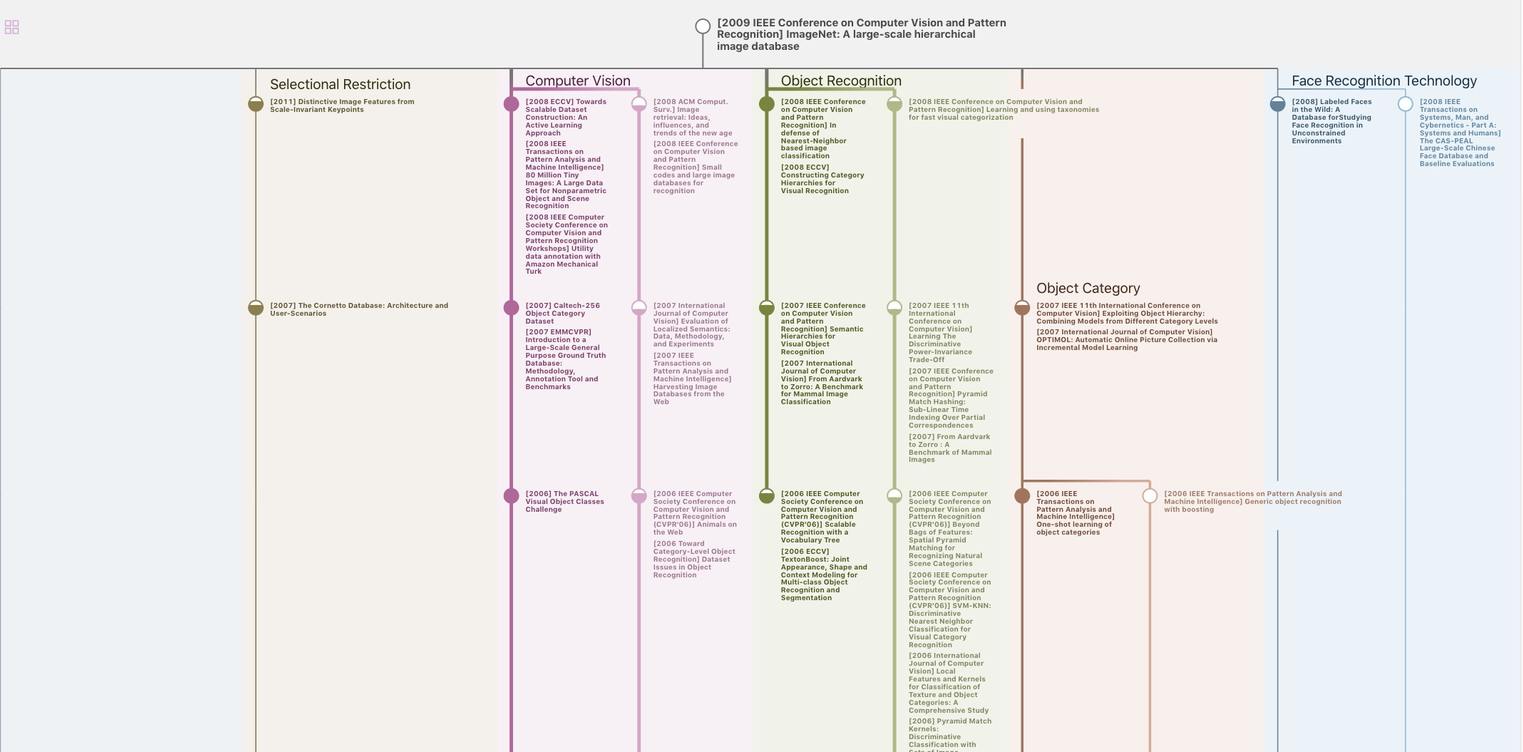
生成溯源树,研究论文发展脉络
Chat Paper
正在生成论文摘要