Measurement errors in Gaussian mixture models using high-dimensional air pollution constituents data
ISEE Conference Abstracts(2022)
摘要
BACKGROUND AND AIM: An active area of current interest in air pollution epidemiology is characterizing the impact of air pollution constituents on health outcomes. Statistical analysis of air pollution data suffers from bias due to measurement error of these exposures, and this bias is exacerbated when the exposures are high-dimensional. It is well known that exposure to fine particles (PM2.5) can cause adverse health effects. However, the independent effects of PM2.5 constituents have not been as well investigated. METHODS: In this work, we develop a novel approach to correcting for the bias due to measurement errors associated with multiple correlated air pollutants with a complex correlated error structure through the use of Gaussian mixture models under the main / external validation design framework. The clusters are corrected for measurement error and then analyzed in a Cox model to investigate the impacts of different air pollutant profiles on time to even outcomes, such as all-cause mortality and cancer incidence. RESULTS: Extensive simulation studies are carried out to investigate the performance of the proposed method, and to explore sensitivity to departures from Gaussian assumptions. The method is illustrated in a study of air pollutants on all-cause mortality in the Nurses’ Health Study, with exposures validated in the RIOPA and MESA studies.
更多查看译文
关键词
gaussian mixture models,pollution,measurement errors,air,high-dimensional
AI 理解论文
溯源树
样例
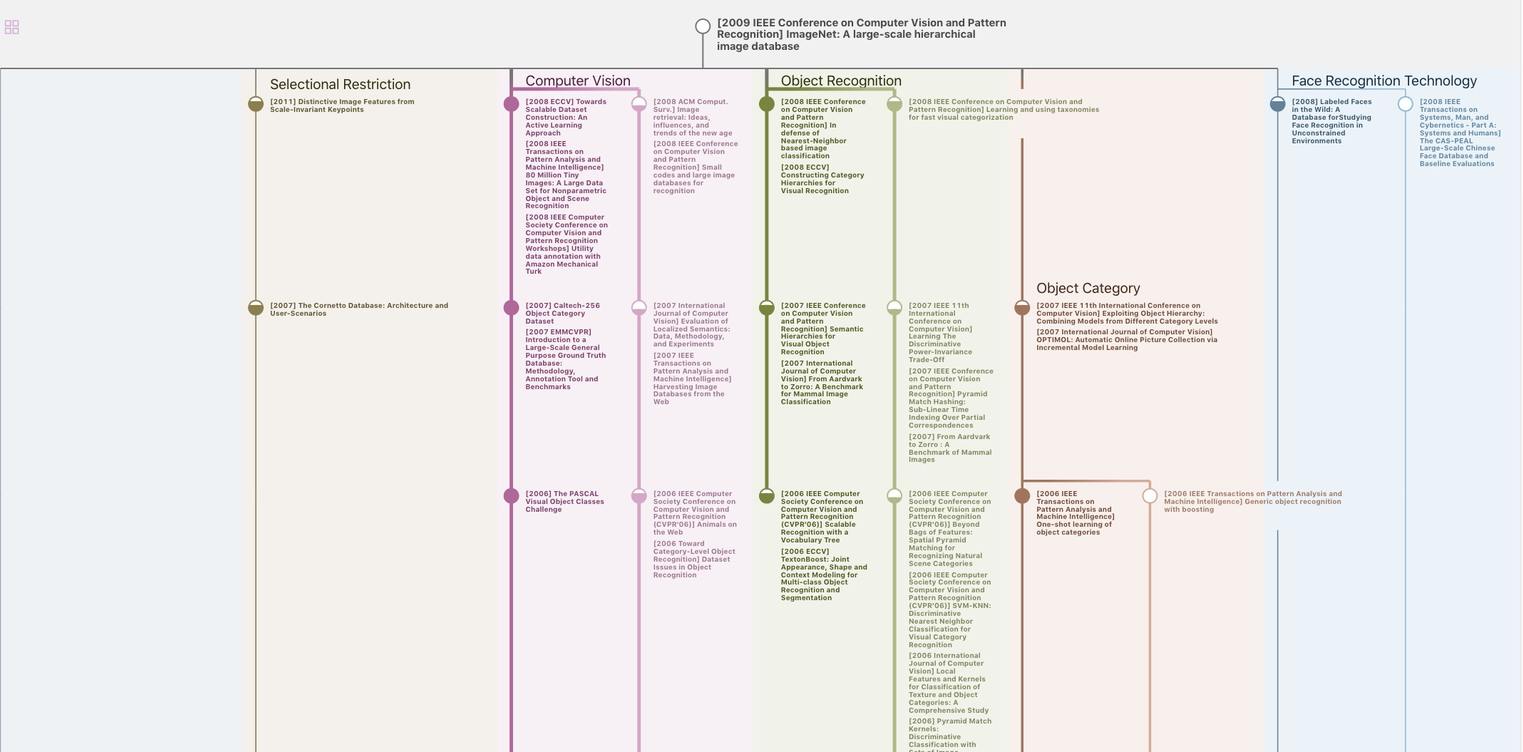
生成溯源树,研究论文发展脉络
Chat Paper
正在生成论文摘要