Bayesian vs Evolutionary Optimisation in Exploring Pareto Fronts for Materials Discovery
crossref(2022)
With advancements in automated experimental setups, material optimisation and discovery can scale to higher throughput with larger evaluation budgets. Two state-of-the-art algorithms with conceptually different multi-objective optimisation strategies (Bayesian and Evolutionary) are compared on synthetic and real-world datasets. Our results show that the Bayesian optimisation strategy, q-Noisy Expected Hypervolume Improvement (qNEHVI) is superior in finding solutions at the Pareto Front rapidly, and when considering hypervolume improvement as a performance indicator. On the other hand, the Evolutionary optimisation strategy, Unified Non-dominated Sorting Genetic Algorithm III (U-NSGA-III), can exploit the Pareto Front and propose a larger pool of optimal solutions, given sufficient evaluation budget, and thus may be a better choice for materials discovery problems where knowing the complete Pareto Front provides greater scientific value to understanding materials space. We discuss the limitations of using hypervolume as a performance indicator for optimisation strategies, alongside hypervolume-based strategies such as qNEHVI, which do not adequately explain the number of solutions at or near the Pareto Front. We also performed a comparison of both optimisation strategies at different batch sizes to consider throughput capabilities.
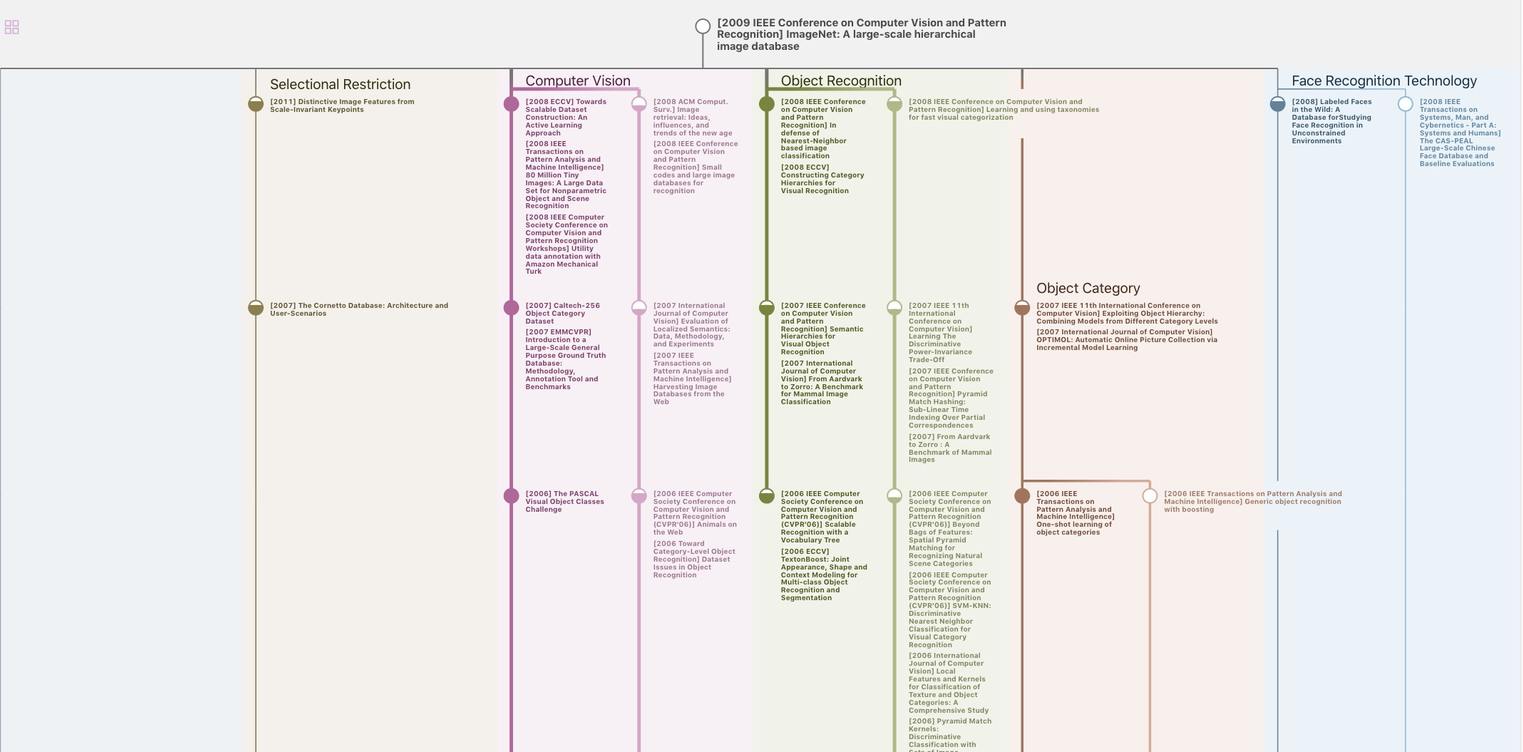