Mitigating the class imbalance effect in Sleep Apnea Classification
2023 International Conference on Recent Advances in Electrical, Electronics & Digital Healthcare Technologies (REEDCON)(2023)
摘要
The prediction of Sleep Apnea and its classification into Apnea and Hypopnea in people with sleep apnea syndrome has not been thoroughly investigated. The primary source of detecting apnea episodes is polysomnography, which is resource intensive and inconvenient. Hence, simpler alternative methods are the requirement. Already proposed techniques usually focus on detecting the sleep apnea events and then giving a count in the form Apnea-Hypopnea Index (AHI). This study focuses on classifying individual sleep apnea events into Apnea and Hypopnea using a minimal number of features that can be easily recorded. A well-known, gold-standard dataset of 25 patients was used for this study. Compared with different classical machine learning algorithms and ensemble methods, this study proposes a 15-layer sequential deep neural network learning model. Using this model, Sensitivity and Specificity of 0.67 and 0.55, respectively, were achieved using 5 input features. While using only 3 input features gave the Sensitivity and Specificity scores of 0.71 and 0.59, respectively, following 24x1 cross validation. The proposed model is better than the existing machine learning methods.
更多查看译文
关键词
Sleep Apnea,Hypopnea,Deep Learning,Machine Learning,Imbalance
AI 理解论文
溯源树
样例
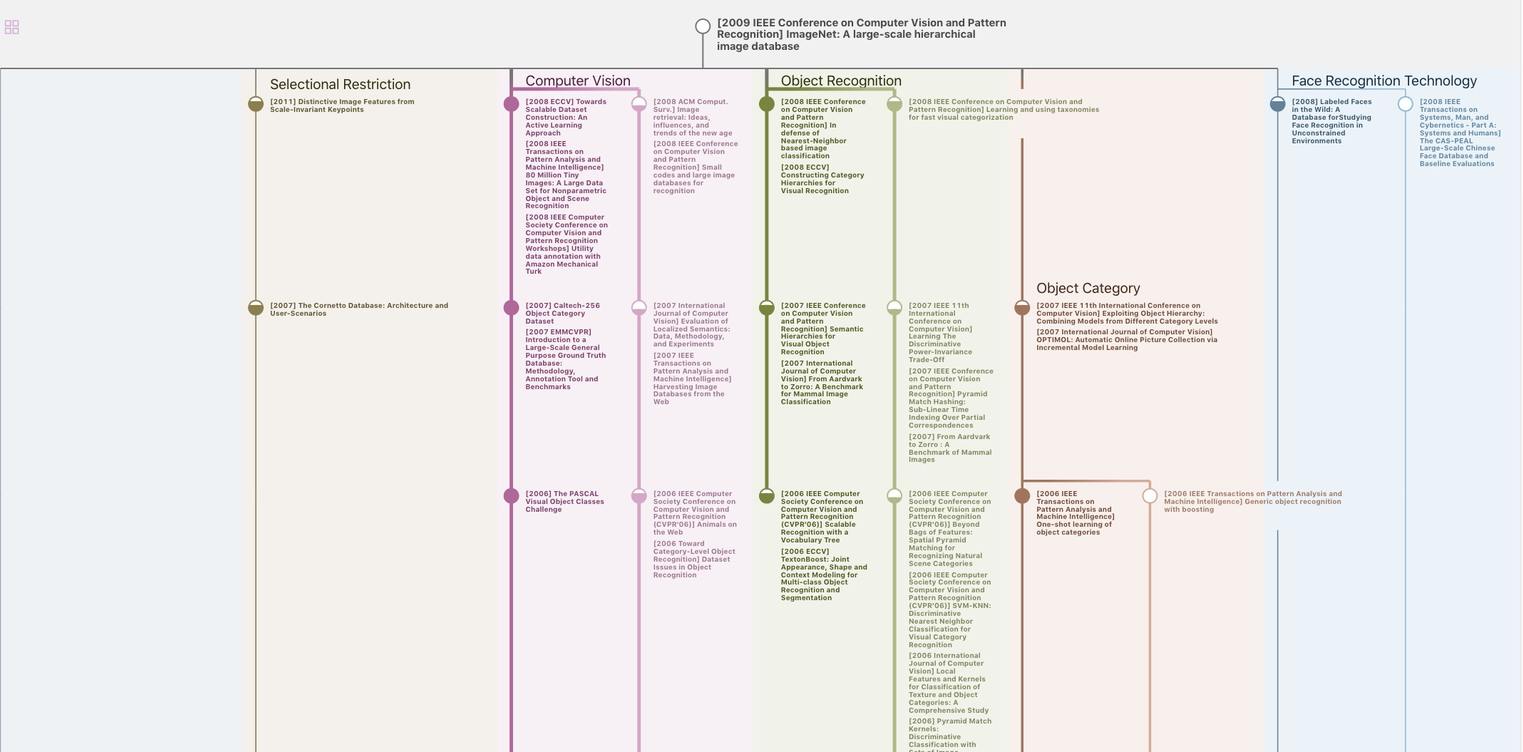
生成溯源树,研究论文发展脉络
Chat Paper
正在生成论文摘要