Sleep Apnea Event Detection Using EEG Band Entropy Features and Machine Learning
2023 International Conference on Recent Advances in Electrical, Electronics & Digital Healthcare Technologies (REEDCON)(2023)
摘要
Sleep is one of the essential biological components of human life comprising one-third of our complete lifespan. Sleep quality is affected by various breathing, cardiovascular, and movement disorders. Sleep Apnea is one of the significant undiagnosed sleep-breathing disorders that can cause various neural and cardiovascular disorders in the long term. Among all polysomnography signals, Electroencephalogram (EEG) is majorly affected by sleep-related issues. Thus, EEG is a vital biomedical signal used for the detection of sleep apnea. In this work, Shannon entropy and log-energy entropy-based automated sleep apnea classification method is proposed to distinguish apnea and normal events in an apneic patient. The EEG signal is first preprocessed by filtering out the undesired frequency components using the IIR Butterworth bandpass filter and then disbanded into five frequency sub-bands (Delta, Theta, Alpha, Sigma, and Beta). EEG band entropies are calculated as features on each sub-band for the classification of sleep apnea and normal events. St. Vincent’s sleep apnea database is used to evaluate the performance of the proposed technique. The weighted KNN classifier gives the best result with the highest accuracy of 98.90%, sensitivity of 97.80%, and specificity of 100%. The proposed method offers better results in terms of sensitivity, specificity, and accuracy using a lesser number of features as compared to some of the latest published work on the same database.
更多查看译文
关键词
Sleep Apnea,Band filtering,EEG,IIR Butterworth bandpass filter,Log Energy Entropy,Shannon Entropy,Weighted KNN classifier
AI 理解论文
溯源树
样例
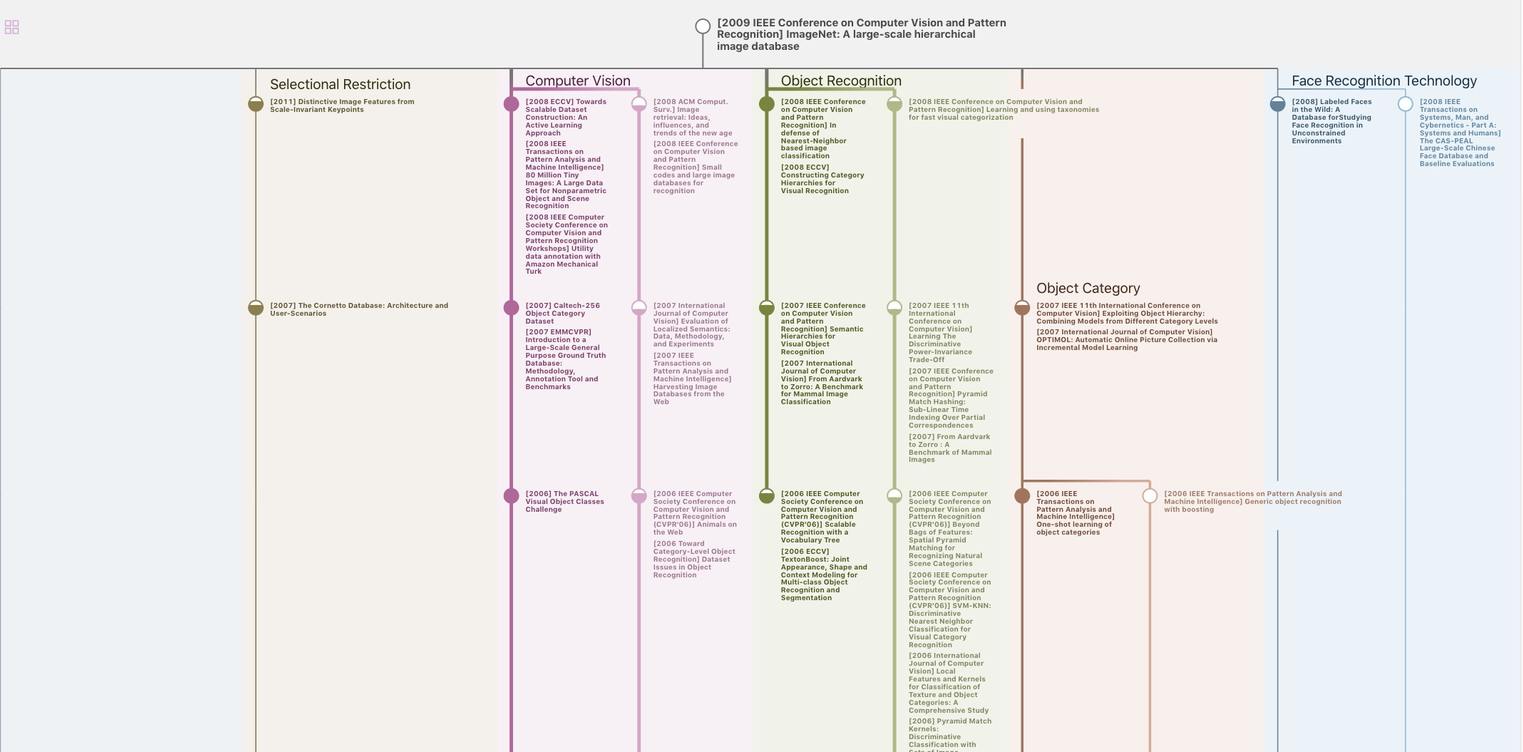
生成溯源树,研究论文发展脉络
Chat Paper
正在生成论文摘要