Ocean Front Detection With Bi-Directional Progressive Fusion Attention Network
IEEE Geoscience and Remote Sensing Letters(2023)
摘要
Ocean fronts are a mesoscale phenomenon in the ocean. It is important for fisheries, environmental protection, and military activities. Therefore, more and more attention has been attracted to ocean front detection. However, the distribution of front and nonfront pixels is highly unbalanced in remote-sensing images, and it is not easy to establish an effective ocean front detection algorithm with high accuracy. To alleviate these problems, we model the problem of detecting ocean fronts as an edge detection task and design a new end-to-end bi-directional progressive fusion attention network (BPFANet). Specifically, BPFANet consists of an effective backbone and a bi-directional path. The whole backbone has four-stage detection blocks (SD blocks), which capture the ocean front features at different scales. Each SD block contains a side branch structure, which includes a deep residual dilated convolution (DRDC) module to enrich multiscale edge information and an attention module (AM) to enhance the feature representation in both the channel and spatial dimensions. In addition, the bi-directional path can progressively fuse the four SD blocks of ocean front information. To evaluate BPFANet, we perform experiments on the OFDS365 dataset and show its advantages over existing ocean front detection methods.
更多查看译文
关键词
Deep learning,edge detection,ocean front
AI 理解论文
溯源树
样例
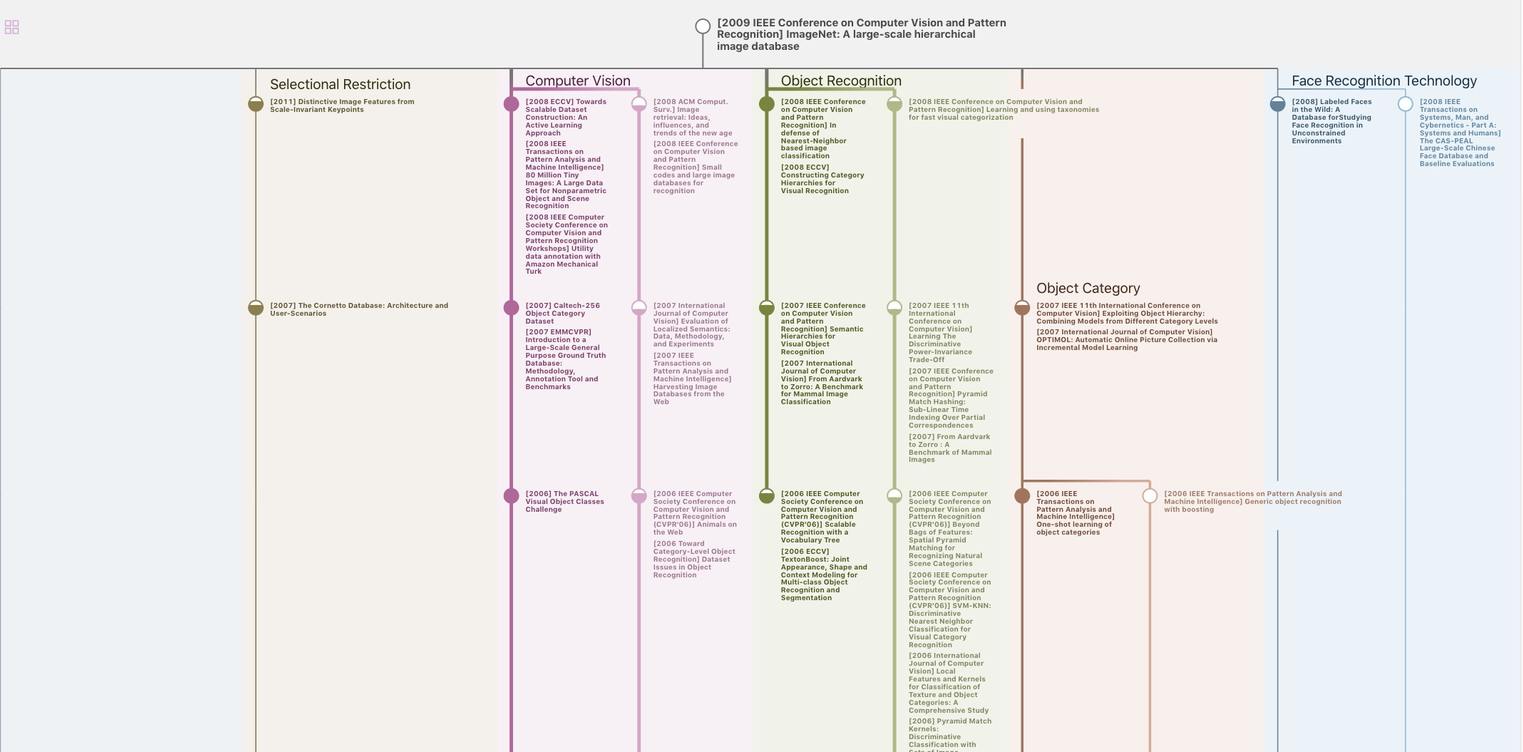
生成溯源树,研究论文发展脉络
Chat Paper
正在生成论文摘要